Text-to-Image Generation for Abstract Concepts
AAAI 2024(2024)
摘要
Recent years have witnessed the substantial progress of large-scale models across various domains, such as natural language processing and computer vision, facilitating the expression of concrete concepts. Unlike concrete concepts that are usually directly associated with physical objects, expressing abstract concepts through natural language requires considerable effort since they are characterized by intricate semantics and connotations. An alternative approach is to leverage images to convey rich visual information as a supplement. Nevertheless, existing Text-to-Image (T2I) models are primarily trained on concrete physical objects and often struggle to visualize abstract concepts. Inspired by the three-layer artwork theory that identifies critical factors, intent, object and form during artistic creation, we propose a framework of Text-to-Image generation for Abstract Concepts (TIAC). The abstract concept is clarified into a clear intent with a detailed definition to avoid ambiguity. LLMs then transform it into semantic-related physical objects, and the concept-dependent form is retrieved from an LLM-extracted form pattern set. Information from these three aspects will be integrated to generate prompts for T2I models via LLM. Evaluation results from human assessments and our newly designed metric concept score demonstrate the effectiveness of our framework in creating images that can sufficiently express abstract concepts.
更多查看译文
关键词
CV: Language and Vision,NLP: Applications,DMKM: Linked Open Data, Knowledge Graphs & KB Completio
AI 理解论文
溯源树
样例
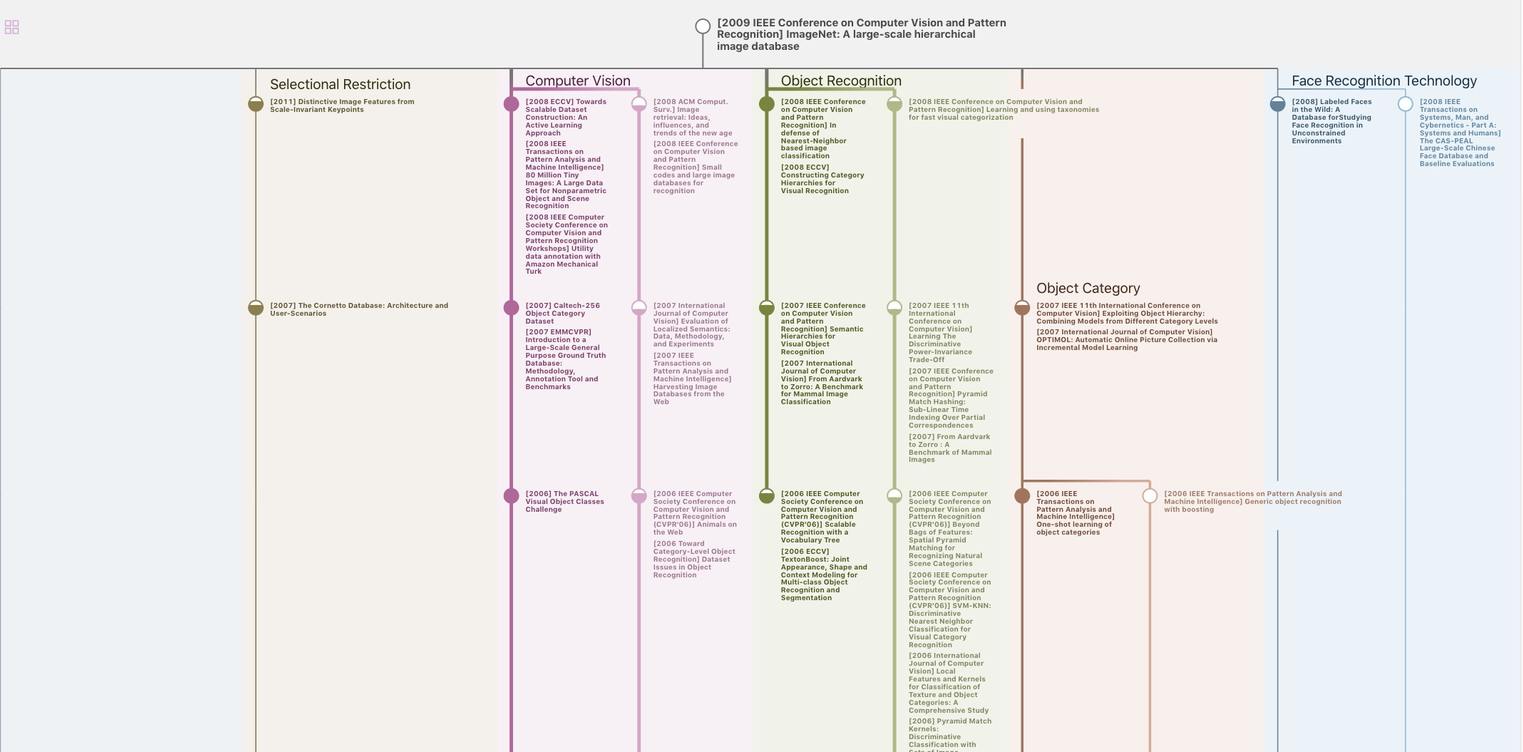
生成溯源树,研究论文发展脉络
Chat Paper
正在生成论文摘要