Neural Novel Actor: Learning a Generalized Animatable Neural Representation for Human Actors.
arxiv(2023)
摘要
We propose a new method for learning a generalized animatable neural human representation from a sparse set of multi-view imagery of multiple persons. The learned representation can be used to synthesize novel view images of an arbitrary person and further animate them with the user's pose control. While most existing methods can either generalize to new persons or synthesize animations with user control, none of them can achieve both at the same time. We attribute this accomplishment to the employment of a 3D proxy for a shared multi-person human model, and further the warping of the spaces of different poses to a shared canonical pose space, in which we learn a neural field and predict the person- and pose-dependent deformations, as well as appearance with the features extracted from input images. To cope with the complexity of the large variations in body shapes, poses, and clothing deformations, we design our neural human model with disentangled geometry and appearance. Furthermore, we utilize the image features both at the spatial point and on the surface points of the 3D proxy for predicting person- and pose-dependent properties. Experiments show that our method significantly outperforms the state-of-the-arts on both tasks.
更多查看译文
关键词
Neural Rendering,Neural Radiance Field,Human Synthesis
AI 理解论文
溯源树
样例
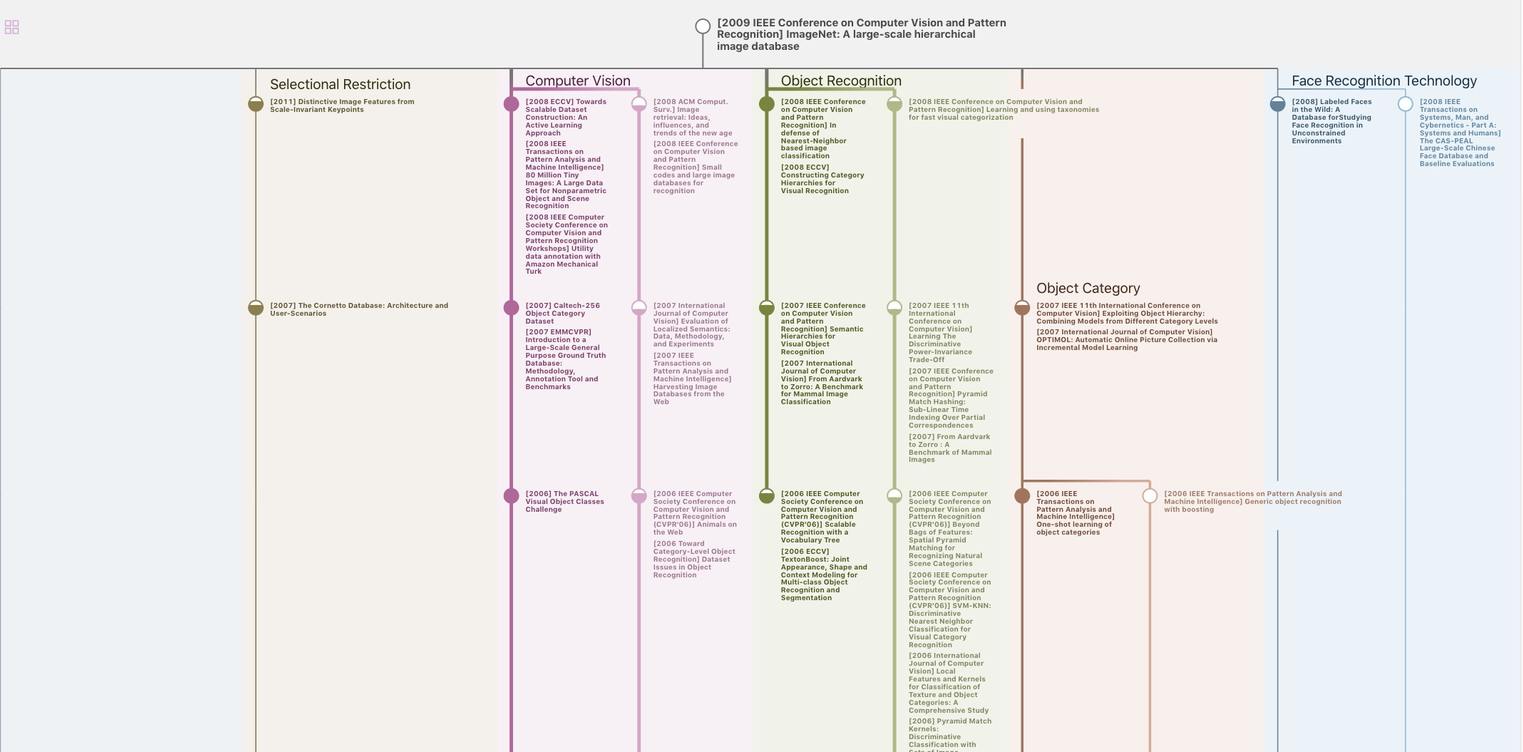
生成溯源树,研究论文发展脉络
Chat Paper
正在生成论文摘要