Don't Chase Your Tail! Missing Key Aspects Augmentation in Textual Vulnerability Descriptions of Long-tail Software through Feature Inference
arxiv(2024)
摘要
Augmenting missing key aspects in Textual Vulnerability Descriptions (TVDs)
for software with a large user base (referred to as non-long-tail software) has
greatly advanced vulnerability analysis and software security research.
However, these methods often overlook software instances that have a limited
user base (referred to as long-tail software) due to limited TVDs, variations
in software features, and domain-specific jargon, which hinders vulnerability
analysis and software repairs. In this paper, we introduce a novel software
feature inference framework designed to augment the missing key aspects of TVDs
for long-tail software. Firstly, we tackle the issue of non-standard software
names found in community-maintained vulnerability databases by
cross-referencing government databases with Common Vulnerabilities and
Exposures (CVEs). Next, we employ Large Language Models (LLMs) to generate the
missing key aspects. However, the limited availability of historical TVDs
restricts the variety of examples. To overcome this limitation, we utilize the
Common Weakness Enumeration (CWE) to classify all TVDs and select cluster
centers as representative examples. To ensure accuracy, we present Natural
Language Inference (NLI) models specifically designed for long-tail software.
These models identify and eliminate incorrect responses. Additionally, we use a
wiki repository to provide explanations for proprietary terms. Our evaluations
demonstrate that our approach significantly improves the accuracy of augmenting
missing key aspects of TVDs for log-tail software from 0.27 to 0.56 (+107
Interestingly, the accuracy of non-long-tail software also increases from 64
to 71
that require complete TVD information.
更多查看译文
AI 理解论文
溯源树
样例
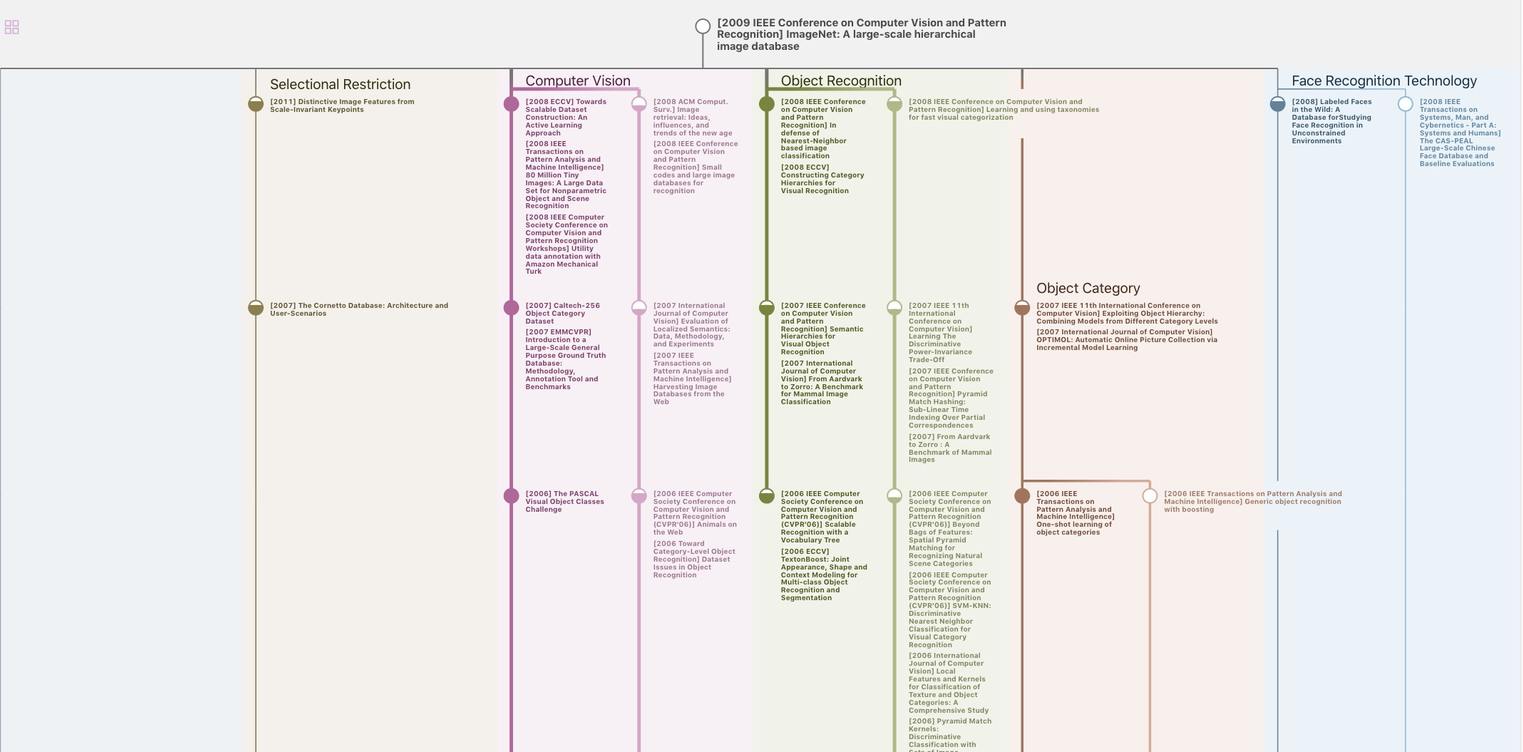
生成溯源树,研究论文发展脉络
Chat Paper
正在生成论文摘要