Real-to-Real Motor Learning of Tendon-Driven Soft Caterpillar Locomotion with World Model
2024 IEEE 7th International Conference on Soft Robotics (RoboSoft)(2024)
摘要
Controlling soft mobile robots that perform limb-less locomotion is costly to develop due to the need to consider friction and the complexity of movement mechanics. There are methods using reinforcement learning (RL) to create controllers for complex soft caterpillar robots. However, these often involve learning through simulation, and model inaccuracies can lead to reduced controller performance upon deployment. In this paper, we created a soft caterpillar robot driven by tendons with two motors and trained a controller using RL. By training with real soft robots without using simulations, we created a learning model that works effectively even with soft robots' complex dynamics, without performance degradation upon deployment. Using a model-based learning algorithm enabled quick policy learning, even with real robots that typically require time-consuming sampling. The learning model we developed could achieve locomotion in forward tasks after about one hour of training. After training, the actual robot was capable of moving at approximately 36.7 mm/s. To the best of our knowledge, this is the first instance of learning locomotion for a soft mobile robot's crawling using only a real robot.
更多查看译文
关键词
Caterpillars,Learning Models,Complex Dynamics,Mobile Robot,Policy Learning,Soft Robots,Real Robot,Actuator,Complex Environment,State Space,Previous Step,Error Model,Robotic System,Actor Network,Shape Memory,Soft Structures,Critic Network,Central Pattern Generator,Simple Environment,Average Reward,Discrete State Space,Goal Position,Quadruped Robot,Replay Buffer,Decoding Model,Serial Communication,Model-based Reinforcement Learning,Mm In Width,Motor Position,Robot Movement
AI 理解论文
溯源树
样例
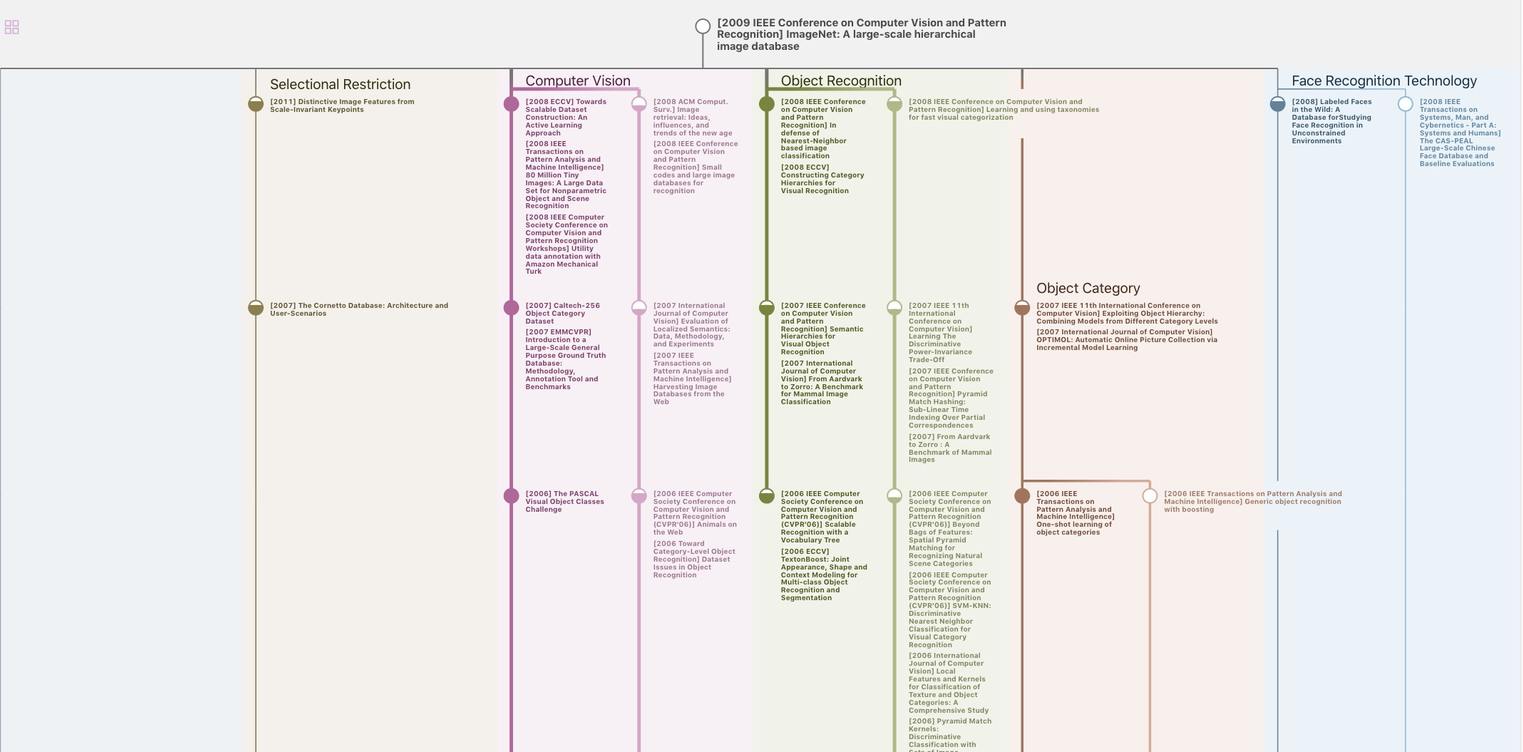
生成溯源树,研究论文发展脉络
Chat Paper
正在生成论文摘要