GA-GGD: Improving semantic discriminability in graph contrastive learning via Generative Adversarial Network
Information Fusion(2024)
摘要
Graph contrastive learning has garnered considerable research interest due to its ability to effectively embed graph data without manual labels. Among them, methods based on Deep Graph Infomax (DGI) have been widely studied and favored in the industry because of their fast training speed, applicability to large-scale data. DGI-based methods usually obtain a noise graph through node shuffling. The proxy task of these methods encourages the encoder to distinguish whether the nodes come from the original graph or the noise graph, thereby maximizing the mutual information between the node representation and the graph it belongs to, while also maximizing the Jenson-Shannon divergence between the nodes of original graph and noise graph. However, we argue that these approaches only enable the encoder to differentiate between semantically meaningful graphs and noise graphs, but not to effectively identify different semantic graphs. This leads to the inability of the encoder to effectively embed information between different semantics, significantly reducing the robustness and affecting the performance of downstream tasks. In addition, this training mode makes the model more sensitive to attacks. To improve their semantic discriminability, we take advantage of the natural ability of generative adversarial networks to generate semantic data, proposing a method called Generative Adversarial Graph Group Discrimination (GA-GGD). Specifically, it consists of a graph group discriminator and a semantic attack generator. The discriminator aims to encode the graph and identify whether nodes originate from the original graph. The goal of the generator is to use random features and graph structure to find vulnerabilities of discriminator and generate node representations with similar but wrong semantic to confuse the discriminator. GA-GGD can improve the model’s semantic information embedding without significantly increasing computational overhead and memory occupancy. We test the effectiveness of the proposed model on commonly used data sets and large-scale datasets, as well as in various downstream tasks such as classification, clustering, and adversarial attacks defence. A wealth of experimental results confirm the efficacy of the proposed model.
更多查看译文
关键词
Graph representation learning,Generative Adversarial Network,Graph contrastive learning,Adversarial Machine Learning,Semantic discriminability
AI 理解论文
溯源树
样例
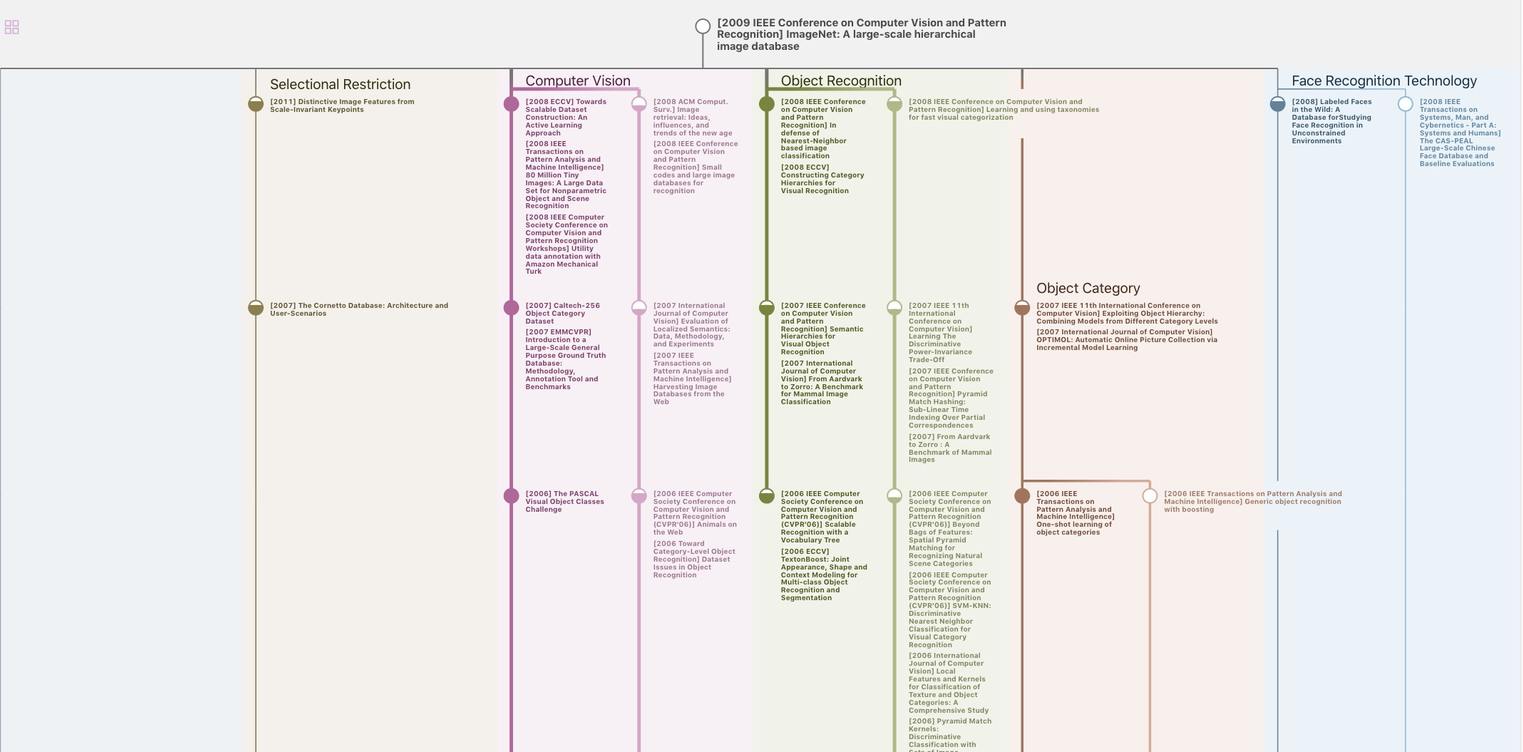
生成溯源树,研究论文发展脉络
Chat Paper
正在生成论文摘要