Cascading Failure Prediction in Power Grid Using Node and Edge Attributed Graph Neural Networks
2024 IEEE Green Technologies Conference (GreenTech)(2024)
摘要
Probabilistic data-driven methods allow faster exploration at low computational cost for cascading failure analysis in power systems compared to statistical physics-based methods. Motivated by the nature of failure propagation, we propose using Graph Neural Networks (GNNs) to study cascading failures in power grids in an end-to-end manner. The goal is to train GNNs using power grid profiles conditioned on component failures and predict the vulnerability of buses and branches after cascade termination. We empirically verify several formulations of GNNs on these tasks. Our evaluation demonstrates the efficiency of GNN-based methods for end-to-end cascading failure prediction on the IEEE 39-bus and 118-bus test systems. Code at https://github.com/karuna-bhaila/gnn-cascading-failure.
更多查看译文
关键词
Power grids,Cascading failures,Graph neural networks
AI 理解论文
溯源树
样例
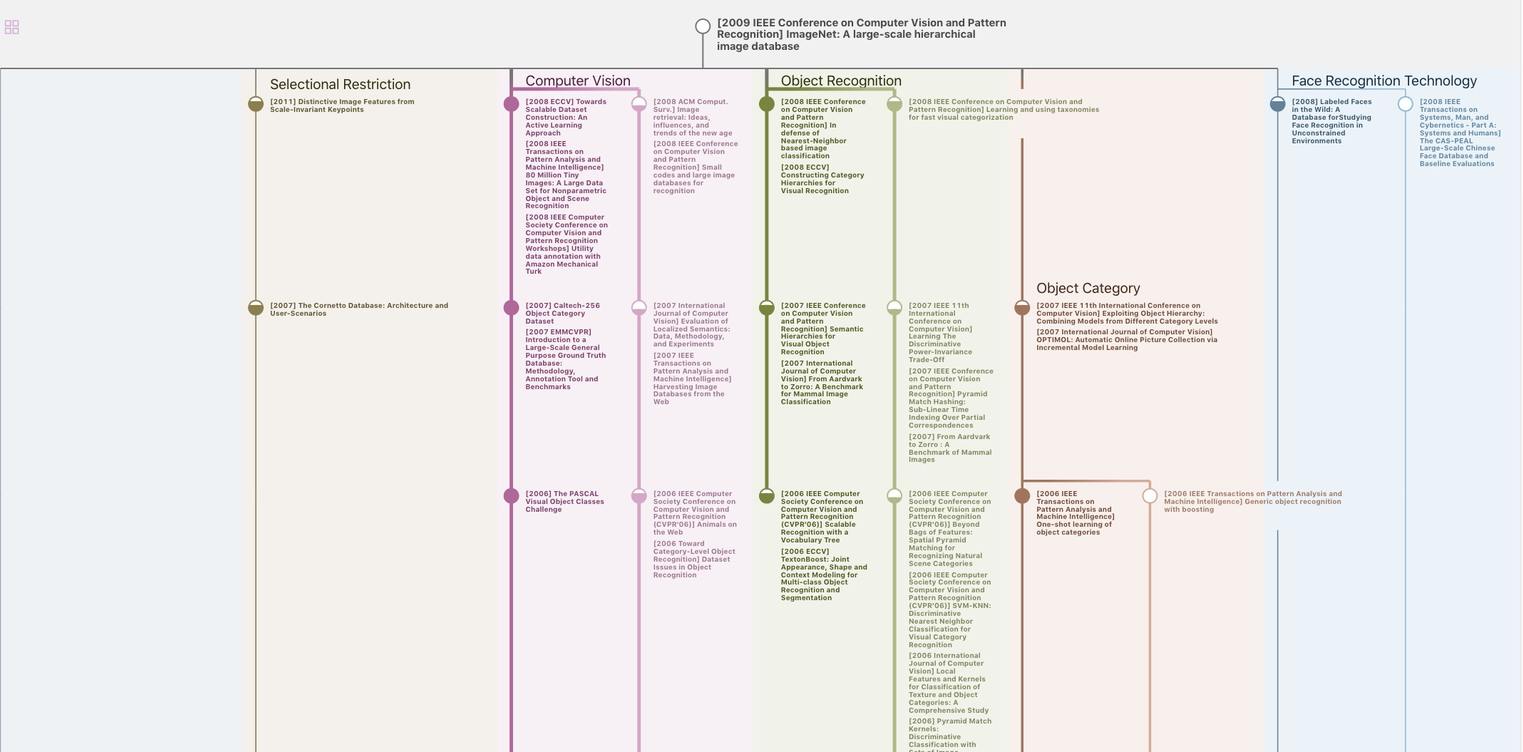
生成溯源树,研究论文发展脉络
Chat Paper
正在生成论文摘要