Learning content-aware feature fusion for guided depth map super-resolution
Signal Processing: Image Communication(2024)
摘要
RGB-D data including paired RGB color images and depth maps is widely used in downstream computer vision tasks. However, compared with the acquisition of high-resolution color images, the depth maps captured by consumer-level sensors are always in low resolution. Within decades of research, the most state-of-the-art (SOTA) methods of depth map super-resolution cannot adaptively tune the guidance fusion for all feature positions by channel-wise feature concatenation with spatially sharing convolutional kernels. This paper proposes JTFNet to resolve this issue, which simulates the traditional Joint Trilateral Filter (JTF). Specifically, a novel JTF block is introduced to adaptively tune the fusion pattern between the color features and the depth features for all feature positions. Moreover, based on the variant of JTF block whose target features and guidance features are in the cross-scale shape, the fusion for depth features is performed in a bi-directional way. Therefore, the error accumulation along scales can be effectively mitigated by iteratively HR feature guidance. Compared with the SOTA methods, the sufficient experiment is conducted on the mainstream synthetic datasets and real datasets, i.e., Middlebury, NYU and ToF-Mark, which shows remarkable improvement of our JTFNet.
更多查看译文
关键词
Convolutional neural network,Joint trilateral filter,Guided depth map super-resolution,Content-dependent network
AI 理解论文
溯源树
样例
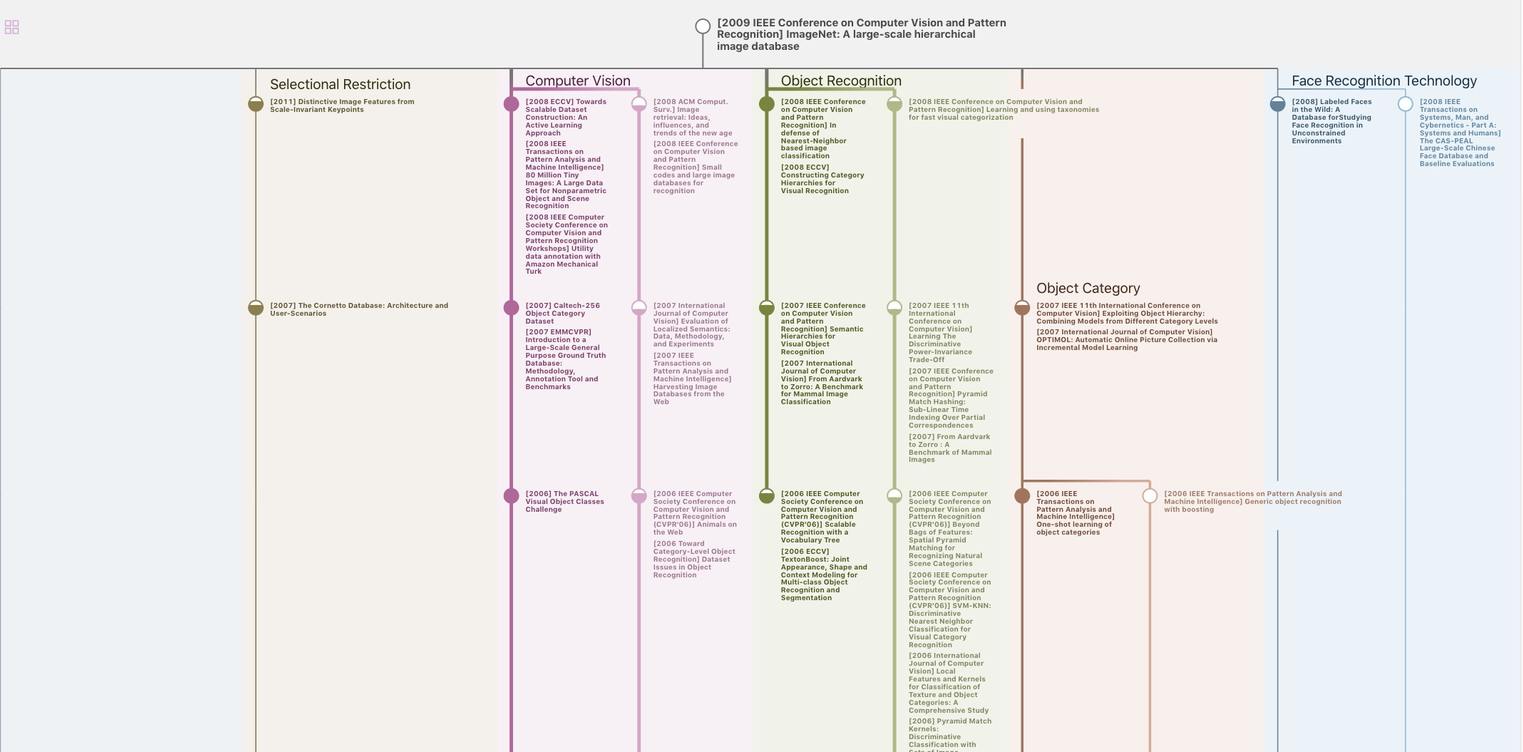
生成溯源树,研究论文发展脉络
Chat Paper
正在生成论文摘要