Binary Classification Under l0 Attacks for General Noise Distribution
IEEE TRANSACTIONS ON INFORMATION THEORY(2024)
摘要
Adversarial examples have recently drawn considerable attention in the field of machine learning due to the fact that small perturbations in the data can result in major performance degradation. This phenomenon is usually modeled by a malicious adversary that can apply perturbations to the data in a constrained fashion, such as being bounded in a certain norm. In this paper, we study this problem when the adversary is constrained by the l(0) norm; i.e., it can perturb a certain number of coordinates in the input, but has no limit on how much it can perturb those coordinates. Due to the combinatorial nature of this setting, we need to go beyond the standard techniques in robust machine learning to address this problem. We consider a binary classification scenario where root d noisy data samples of the true label are provided to us after adversarial perturbations. We introduce a classification method which employs a nonlinear component called truncation, and show in an asymptotic scenario, as long as the adversary is restricted to perturb no more than root d data samples, we can almost achieve the optimal classification error in the absence of the adversary, i.e., we can completely neutralize adversary's effect. Surprisingly, we observe a phase transition in the sense that using a converse argument, we show that if the adversary can perturb more than root d coordinates, no classifier can do better than a random guess.
更多查看译文
关键词
Robust classification,sparse adversarial attacks,adversarial learning
AI 理解论文
溯源树
样例
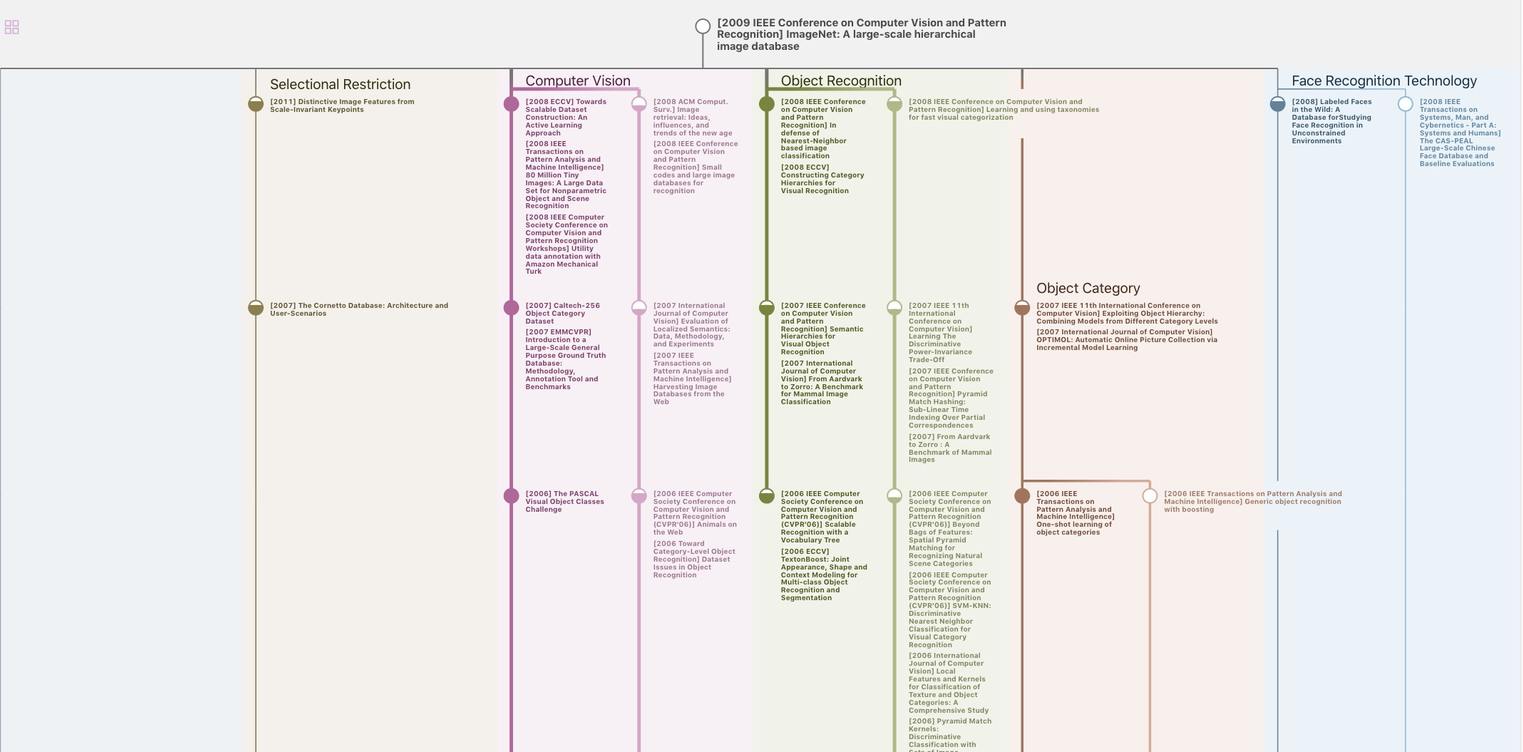
生成溯源树,研究论文发展脉络
Chat Paper
正在生成论文摘要