Reading Broadly to Open Your Mind: Improving Open Relation Extraction With Search Documents Under Self-Supervisions
IEEE TRANSACTIONS ON KNOWLEDGE AND DATA ENGINEERING(2024)
摘要
Open relation extraction is the task of extracting open-domain relation facts from natural language sentences. Existing works either utilize distant-supervised annotations to train a supervised classifier over pre-defined relations, or adopt unsupervised methods with additional dependency on external assumptions. However, these works can only obtain information signals from limited existing knowledge bases or datasets. In this work, we propose a self-supervised framework named Web-SelfORE, which exploits self-supervised signals by requiring a large pretrained language model to extensively read real-world relevant documents from the web, and obtain contextualized relational features by mixing contextualized representations of entities from different documents. We perform adaptive clustering on contextualized relational features and bootstrap the self-supervised signals by improving contextualized features in relation classification. We additionally compare the effectiveness of self-supervisions brought by different document sources, and introduce relevance and redundancy evaluation metrics to obtain higher-quality self-supervisions. Experimental results on four public datasets show the effectiveness and robustness of Web-SelfORE on open-domain relation extraction task when comparing with competitive baselines.
更多查看译文
关键词
Internet,Data mining,Online services,Encyclopedias,Annotations,Feature extraction,Knowledge based systems,Relation extraction,open-domain learning,retrieval-based learning,self-supervised learning
AI 理解论文
溯源树
样例
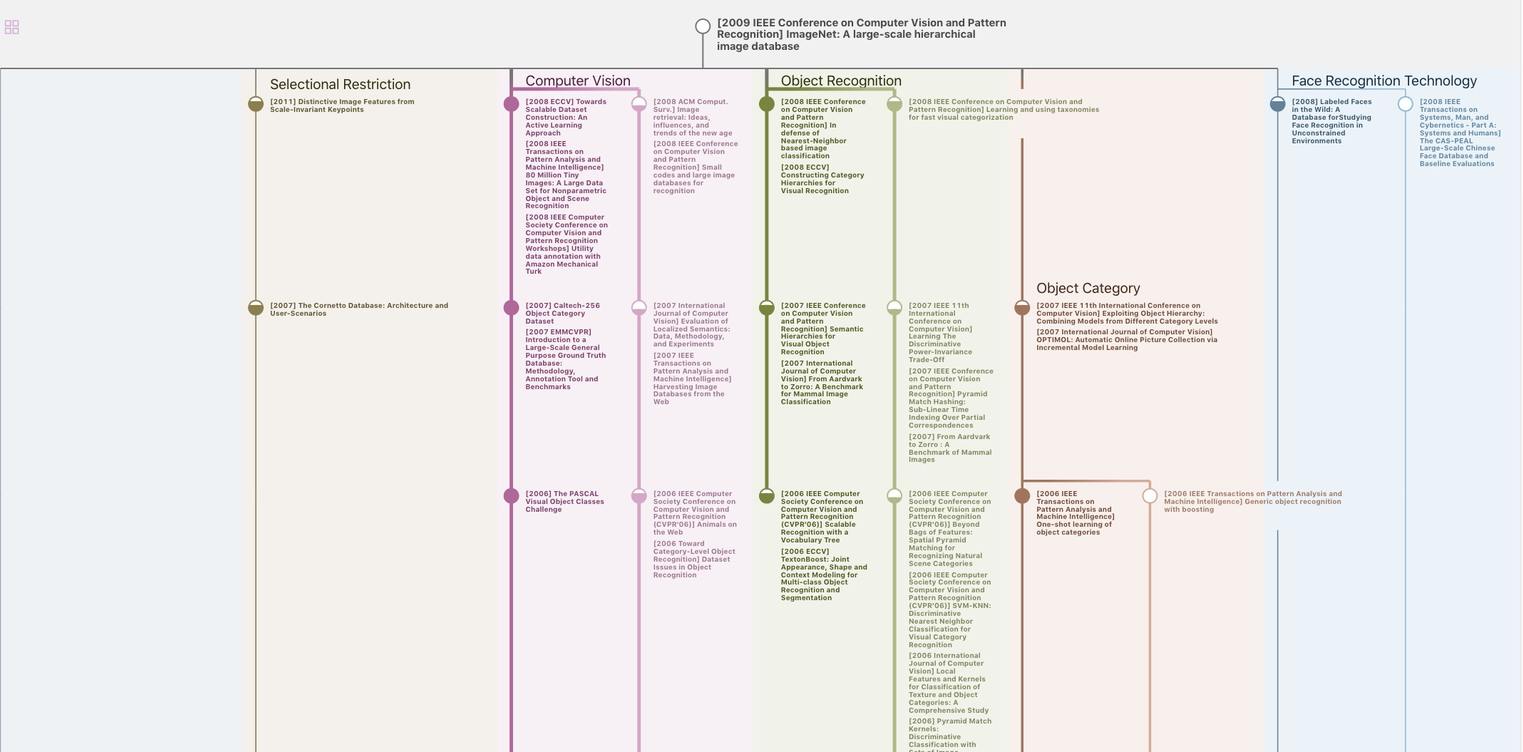
生成溯源树,研究论文发展脉络
Chat Paper
正在生成论文摘要