Lossy Compression with Data, Perception, and Classification Constraints
arxiv(2024)
摘要
Balancing diverse task objectives under limited rate is crucial for
developing robust multi-task deep learning (DL) models and improving
performance across various domains. In this paper, we consider the lossy
compression problem with human-centric and task-oriented metrics, such as
perceptual quality and classification accuracy. We investigate two ternary
relationships, namely, the rate-distortion-classification (RDC) and
rate-perception-classification (RPC). For both RDC and RPC functions, we derive
the closed-form expressions of the optimal rate for both binary and Gaussian
sources. Notably, both RDC and RPC relationships exhibit distinct
characteristics compared to the previous RDP tradeoff proposed by Blau et al.
Then, we conduct experiments by implementing a DL-based image compression
framework, incorporating rate, distortion, perception, and classification
constraints. The experimental results verify the theoretical characteristics of
RDC and RPC tradeoffs, providing information-theoretical insights into the
design of loss functions to balance diverse task objectives in deep learning.
更多查看译文
AI 理解论文
溯源树
样例
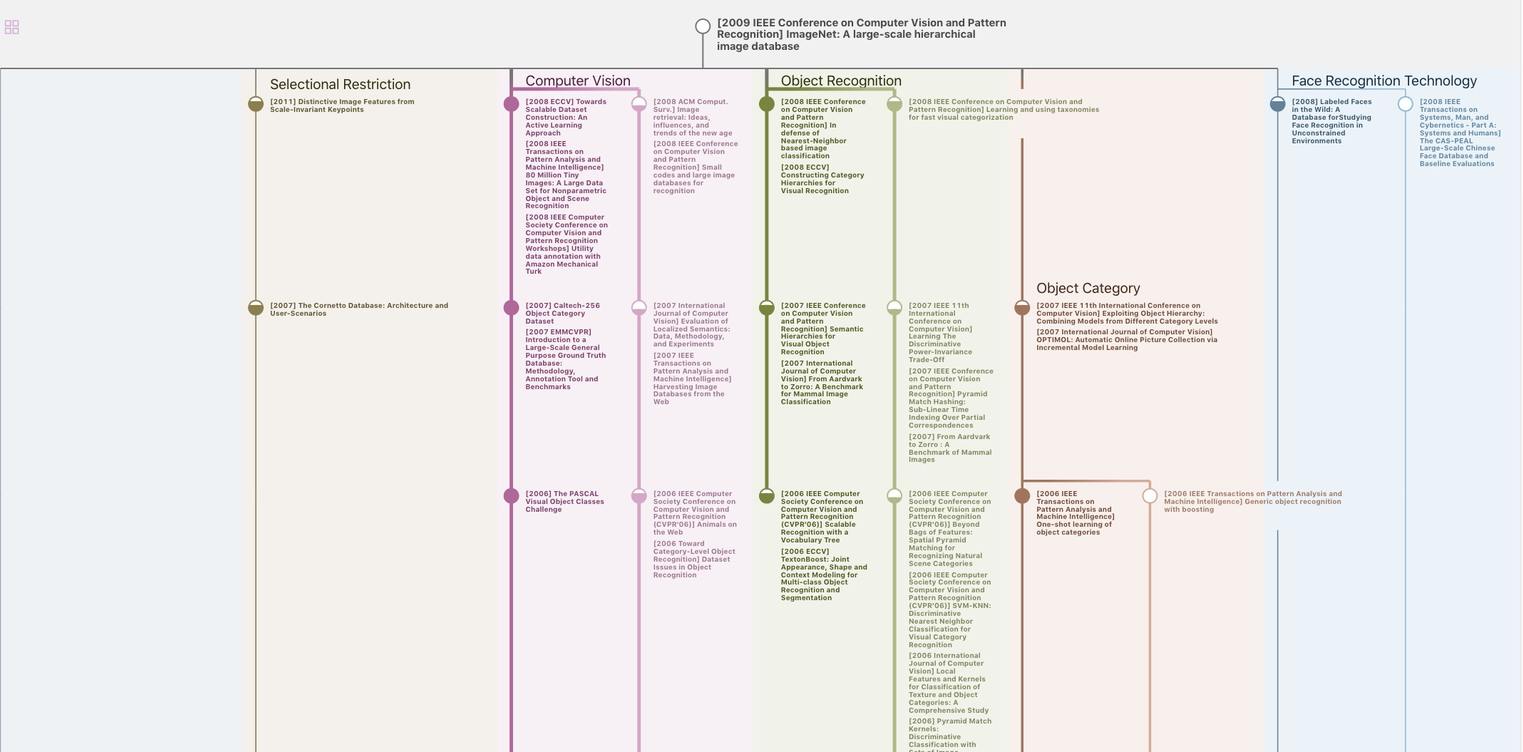
生成溯源树,研究论文发展脉络
Chat Paper
正在生成论文摘要