EOGT: Video Anomaly Detection with Enhanced Object Information and Global Temporal Dependency
ACM Transactions on Multimedia Computing, Communications, and Applications(2024)
摘要
Video anomaly detection (VAD) aims to identify events or scenes in videos that deviate from typical patterns. Existing approaches primarily focus on reconstructing or predicting frames to detect anomalies and have shown improved performance in recent years. However, they often depend highly on local spatio-temporal information and face the challenge of insufficient object feature modeling. To address the above issues, this paper proposes a video anomaly detection framework with E nhanced O bject Information and G lobal T emporal Dependencies (EOGT) and the main novelties are: (1) A L ocal O bject A nomaly S tream (LOAS) is proposed to extract local multimodal spatio-temporal anomaly features at the object level. LOAS integrates two modules: a D iffusion-based O bject R econstruction N etwork (DORN) with multimodal conditions detects anomalies with object RGB information, and an O bject P ose A nomaly Refiner (OPA) discovers anomalies with human pose information. (2) A G lobal T emporal S trengthening S tream (GTSS) with video-level temporal dependencies is proposed, which leverages video-level temporal dependencies to identify long-term and video-specific anomalies effectively. Both streams are jointly employed in EOGT to learn multimodal and multi-scale spatio-temporal anomaly features for VAD, and we finally fuse the anomaly features and scores to detect anomalies at the frame level. Extensive experiments are conducted to verify the performance of EOGT on three public datasets: ShanghaiTech Campus, CUHK Avenue, and UCSD Ped2.
更多查看译文
AI 理解论文
溯源树
样例
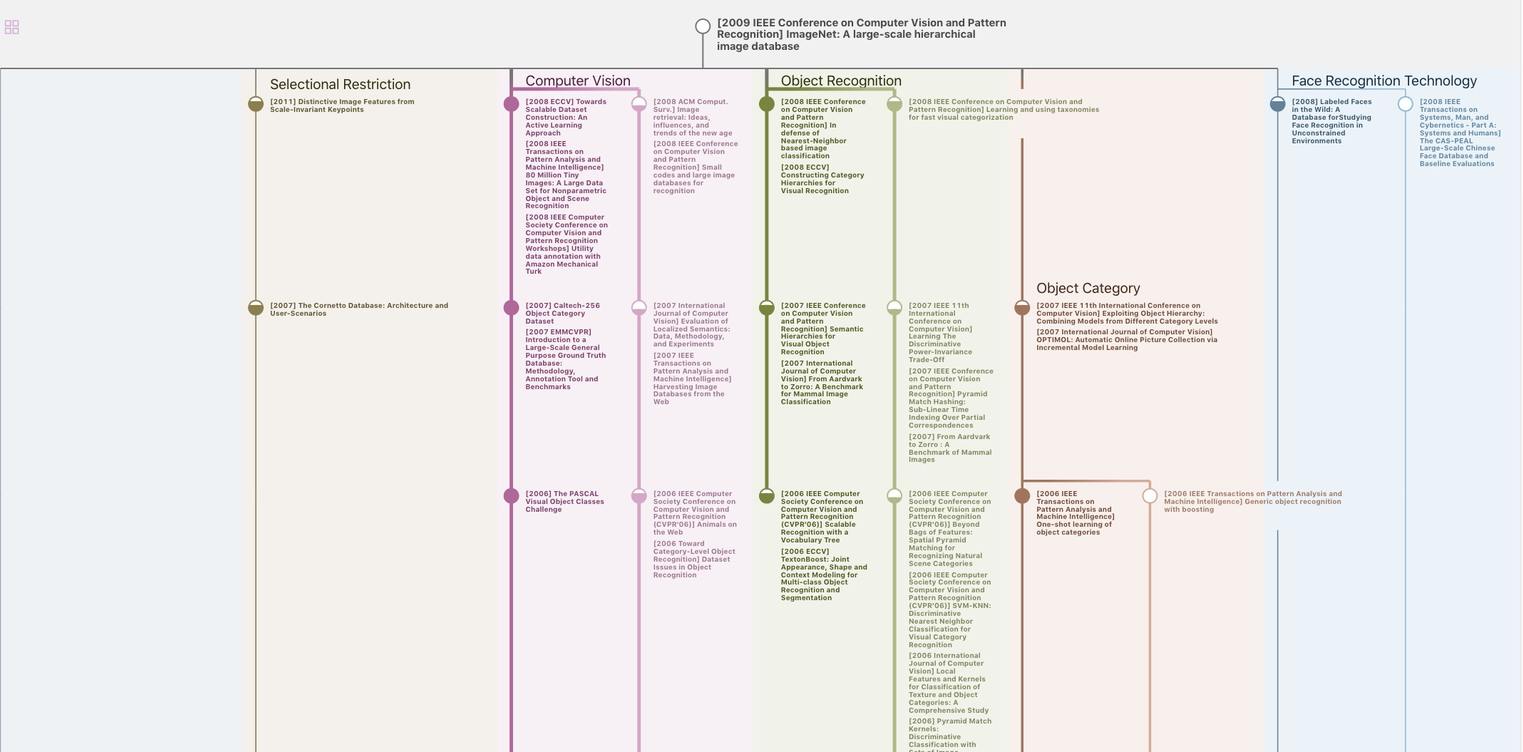
生成溯源树,研究论文发展脉络
Chat Paper
正在生成论文摘要