FastLloyd: Federated, Accurate, Secure, and Tunable k-Means Clustering with Differential Privacy
arxiv(2024)
摘要
We study the problem of privacy-preserving k-means clustering in the
horizontally federated setting. Existing federated approaches using secure
computation, suffer from substantial overheads and do not offer output privacy.
At the same time, differentially private (DP) k-means algorithms assume a
trusted central curator and do not extend to federated settings. Naively
combining the secure and DP solutions results in a protocol with impractical
overhead. Instead, our work provides enhancements to both the DP and secure
computation components, resulting in a design that is faster, more private, and
more accurate than previous work. By utilizing the computational DP model, we
design a lightweight, secure aggregation-based approach that achieves four
orders of magnitude speed-up over state-of-the-art related work. Furthermore,
we not only maintain the utility of the state-of-the-art in the central model
of DP, but we improve the utility further by taking advantage of constrained
clustering techniques.
更多查看译文
AI 理解论文
溯源树
样例
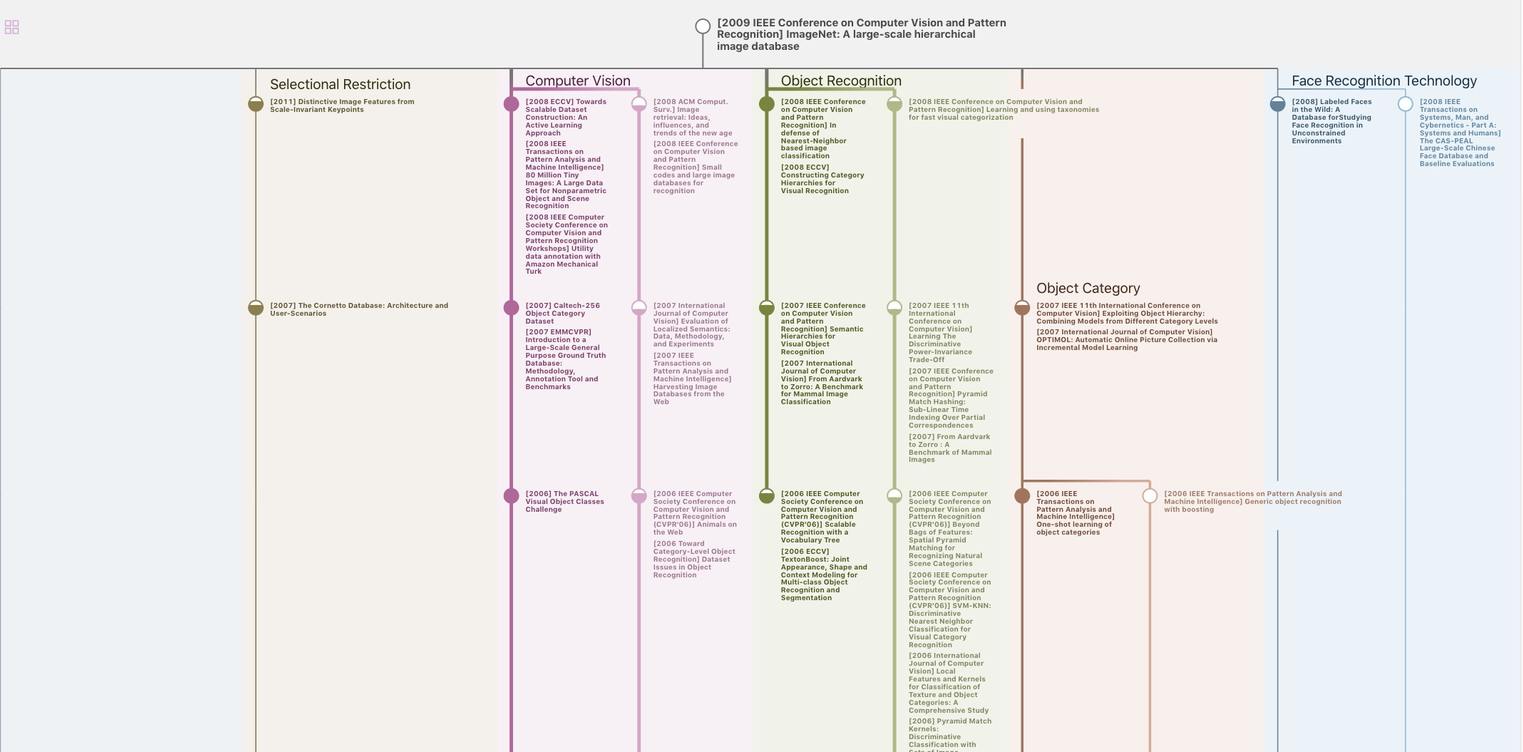
生成溯源树,研究论文发展脉络
Chat Paper
正在生成论文摘要