How do Categorical Duplicates Affect ML? A New Benchmark and Empirical Analyses.
Proc. VLDB Endow.(2024)
摘要
The tedious grunt work involved in data preparation (prep) before ML reduces ML user productivity. It is also a roadblock to industrial-scale cloud AutoML workflows that build ML models for millions of datasets. One important data prep step for ML is cleaning duplicates in the Categorical columns, e.g., deduplicating CA with California in a State column. However, how such Categorical duplicates impact ML is ill-understood as there exist almost no in-depth scientific studies to assess their significance. In this work, we take the first step towards empirically characterizing the impact of Categorical duplicates on ML classification with a three-pronged approach. We first study how Categorical duplicates exhibit themselves by creating a labeled dataset of 1262 Categorical columns. We then curate a downstream benchmark suite of 16 real-world datasets to make observations on the effect of Categorical duplicates on five popular classifiers and five encoding mechanisms. We finally use simulation studies to validate our observations. We find that Logistic Regression and Similarity encoding are more robust to Categorical duplicates than two One-hot encoded high-capacity classifiers. We provide actionable takeaways that can potentially help AutoML developers to build better platforms and ML practitioners to reduce grunt work. While some of the presented insights have remained folklore for practitioners, our work presents the first systematic scientific study to analyze the impact of Categorical duplicates on ML and put this on an empirically rigorous footing. Our work presents novel data artifacts and benchmarks, as well as novel empirical analyses to spur more research on this topic.
更多查看译文
AI 理解论文
溯源树
样例
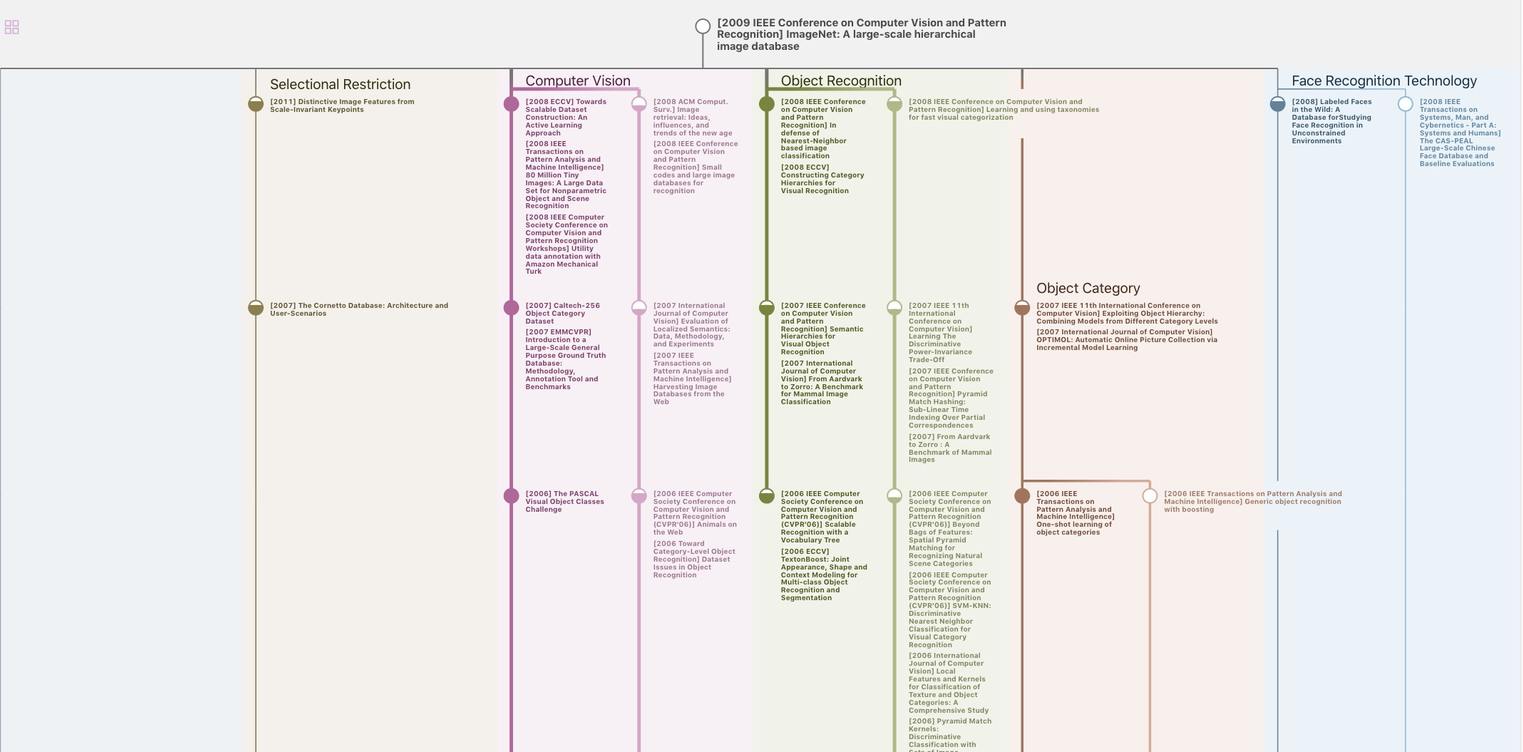
生成溯源树,研究论文发展脉络
Chat Paper
正在生成论文摘要