Multi-Scale and Multi-Level Feature Fusion Network for Quantitative Precipitation Estimation with Passive Microwave
IEEE Transactions on Geoscience and Remote Sensing(2024)
摘要
Passive microwave (PMW) radiometers have been widely utilized for quantitative precipitation estimation (QPE) by leveraging the relationship between brightness temperature (Tb) and rain rate. Nevertheless, accurate precipitation estimation remains a challenge due to the intricate relationship between them, which is influenced by a diverse range of complex atmospheric and surface properties. Additionally, the inherent skew distribution of rainfall values prevents models from correctly addressing extreme precipitation events, leading to a significant underestimation. This paper presents a novel model called the Multi-Scale and Multi-Level Feature Fusion Network (MSMLNet), consisting of two essential components: a multi-scale feature extractor and a multi-level regression predictor. The feature extractor is specifically designed to extract characteristics from multiple scales, enabling the model to incorporate various meteorological conditions, as well as atmospheric and surface information in the surrounding environment. The regression predictor first assesses the probabilities of multiple rainfall levels for each observed pixel and then extracts features of different levels separately. The multi-level features are fused according to the predicted probabilities. This approach allows each sub-module only to focus on a specific range of precipitation, avoiding the undesirable effects of skew distributions. To evaluate the performance of MSMLNet, various deep learning methods are adapted for the precipitation retrieval task, and a PWM-based product from the Global Precipitation Measurement (GPM) mission is also used for comparison. Extensive experiments show that MSMLNet surpasses GMI-based products and the most advanced deep learning approaches by 17.9% and 2.5% in RMSE, and 54.2% and 4.0% in CSI-10, respectively. Moreover, we demonstrate that MSMLNet significantly mitigates the propensity for underestimating heavy precipitation events and has a consistent and outstanding performance in estimating precipitation across various levels.
更多查看译文
关键词
Quantitative precipitation estimation (QPE),passive microwave (PWM),vision transformer (ViT),convolution neural network (CNN)
AI 理解论文
溯源树
样例
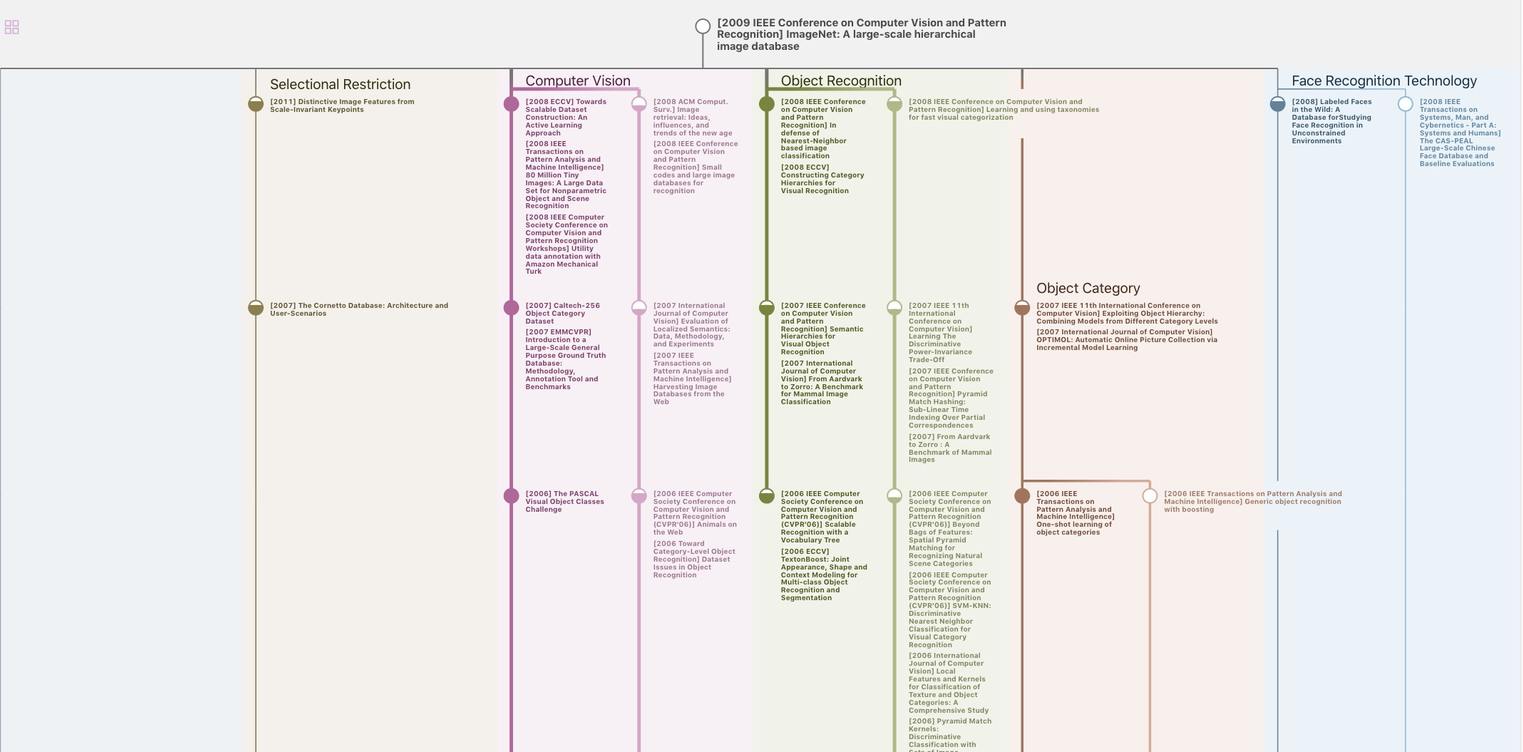
生成溯源树,研究论文发展脉络
Chat Paper
正在生成论文摘要