Structure learning of Hamiltonians from real-time evolution
arxiv(2024)
摘要
We initiate the study of Hamiltonian structure learning from real-time
evolution: given the ability to apply e^-i Ht for an unknown local
Hamiltonian H = ∑_a = 1^m λ_a E_a on n qubits, the goal is to
recover H. This problem is already well-studied under the assumption that the
interaction terms, E_a, are given, and only the interaction strengths,
λ_a, are unknown. But is it possible to learn a local Hamiltonian
without prior knowledge of its interaction structure?
We present a new, general approach to Hamiltonian learning that not only
solves the challenging structure learning variant, but also resolves other open
questions in the area, all while achieving the gold standard of
Heisenberg-limited scaling. In particular, our algorithm recovers the
Hamiltonian to ε error with an evolution time scaling with
1/ε, and has the following appealing properties: (1) it does not
need to know the Hamiltonian terms; (2) it works beyond the short-range
setting, extending to any Hamiltonian H where the sum of terms interacting
with a qubit has bounded norm; (3) it evolves according to H in constant time
t increments, thus achieving constant time resolution. To our knowledge, no
prior algorithm with Heisenberg-limited scaling existed with even one of these
properties. As an application, we can also learn Hamiltonians exhibiting
power-law decay up to accuracy ε with total evolution time beating
the standard limit of 1/ε^2.
更多查看译文
AI 理解论文
溯源树
样例
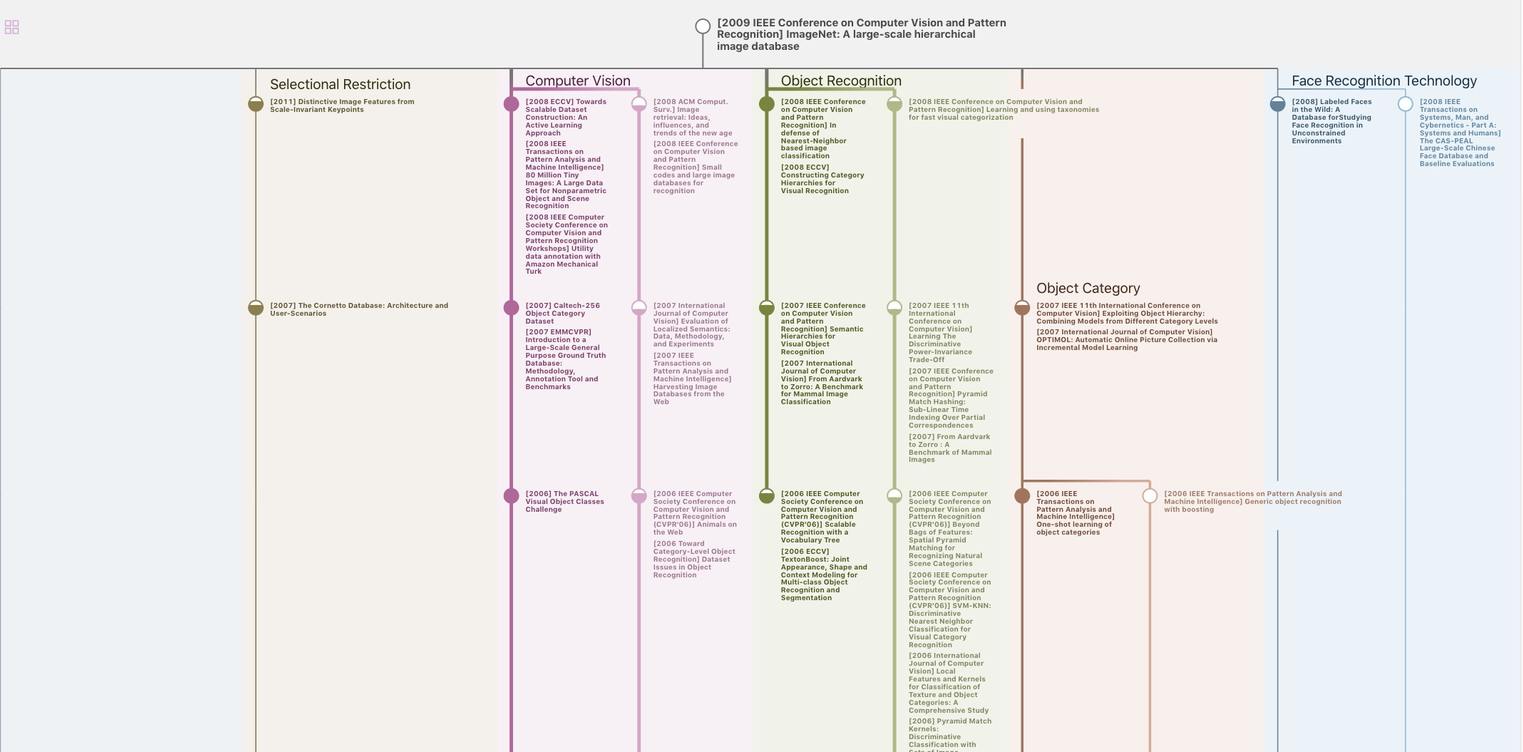
生成溯源树,研究论文发展脉络
Chat Paper
正在生成论文摘要