MIMO-ODDM Signal Detection: A Spatial-based Generative Adversarial Network Approach
IEEE Transactions on Wireless Communications(2024)
摘要
The recently emerged multiple-input multiple-output over orthogonal delay-Doppler division multiplexing (MIMO-ODDM) is gaining paramount interest as a promising solution to provide reliable communication performance for high-mobility systems. To achieve its full potential, signal detection becomes a critical issue, while the performance of existing methods is yet to be satisfactory. In this paper, we develop a novel signal detection approach for MIMO-ODDM systems by leveraging the spatial-based generative adversarial network, namely SG-ODDM, for accurate, interference-resilient and environment-robust performance. We creatively design a spatial-based generative adversarial network (spatial-based GAN) for comprehensive feature extraction and interference mitigation. We propose a transfer learning-based adaptive updating (TAU) to enhance environmental robustness by reducing the frequency and effort in updating the detection model. Extensive simulation results verify that the proposed SG-ODDM is considerably superior to state-of-the-art related works, in terms of detection accuracy, interference resilience and updating effort reduction.
更多查看译文
关键词
MIMO-ODDM,deep learning,signal detection,Spatial-based generative adversarial network
AI 理解论文
溯源树
样例
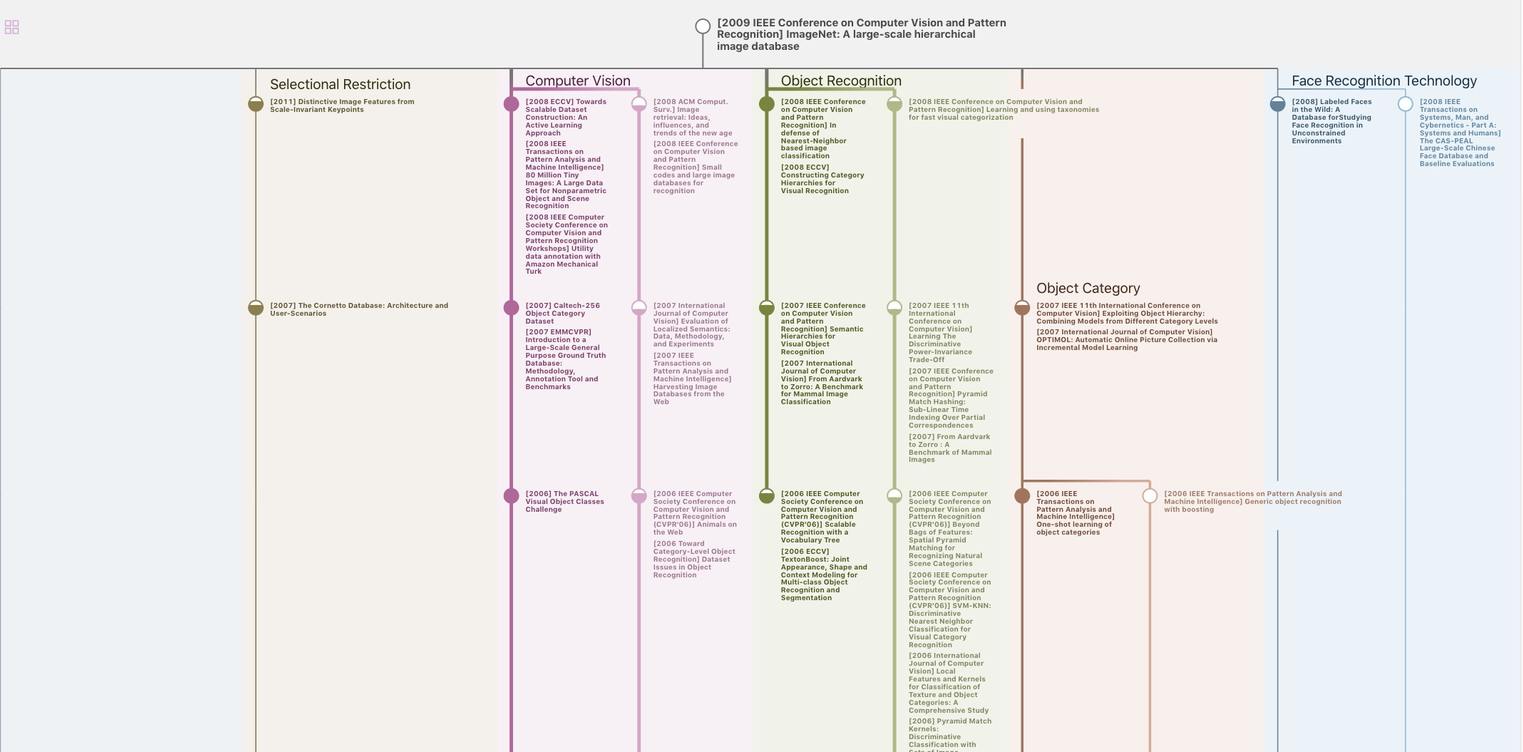
生成溯源树,研究论文发展脉络
Chat Paper
正在生成论文摘要