Automated individual cortical parcellation via consensus graph representation learning
NeuroImage(2024)
摘要
Cortical parcellation plays a pivotal role in elucidating the brain organization. Despite the growing efforts to develop parcellation algorithms using functional magnetic resonance imaging, achieving a balance between intra-individual specificity and inter-individual consistency proves challenging, making the generation of high-quality, subject-consistent cortical parcellations particularly elusive. To solve this problem, our paper proposes a fully automated individual cortical parcellation method based on consensus graph representation learning. The method integrates spectral embedding with low-rank tensor learning into a unified optimization model, which uses group-common connectivity patterns captured by low-rank tensor learning to optimize subjects’ functional networks. This not only ensures consistency in brain representations across different subjects but also enhances the quality of each subject’s representation matrix by eliminating spurious connections. More importantly, it achieves an adaptive balance between intra-individual specificity and inter-individual consistency during this process. Experiments conducted on a test-retest dataset from the Human Connectome Project (HCP) demonstrate that our method outperforms existing methods in terms of reproducibility, functional homogeneity, and alignment with task activation. Extensive network-based comparisons on the HCP S900 dataset reveal that the functional network derived from our cortical parcellation method exhibits greater capabilities in gender identification and behavior prediction than other approaches.
更多查看译文
关键词
Cortical parcellation,Spectral embedding,Low-rank tensor learning,Functional magnetic resonance imaging
AI 理解论文
溯源树
样例
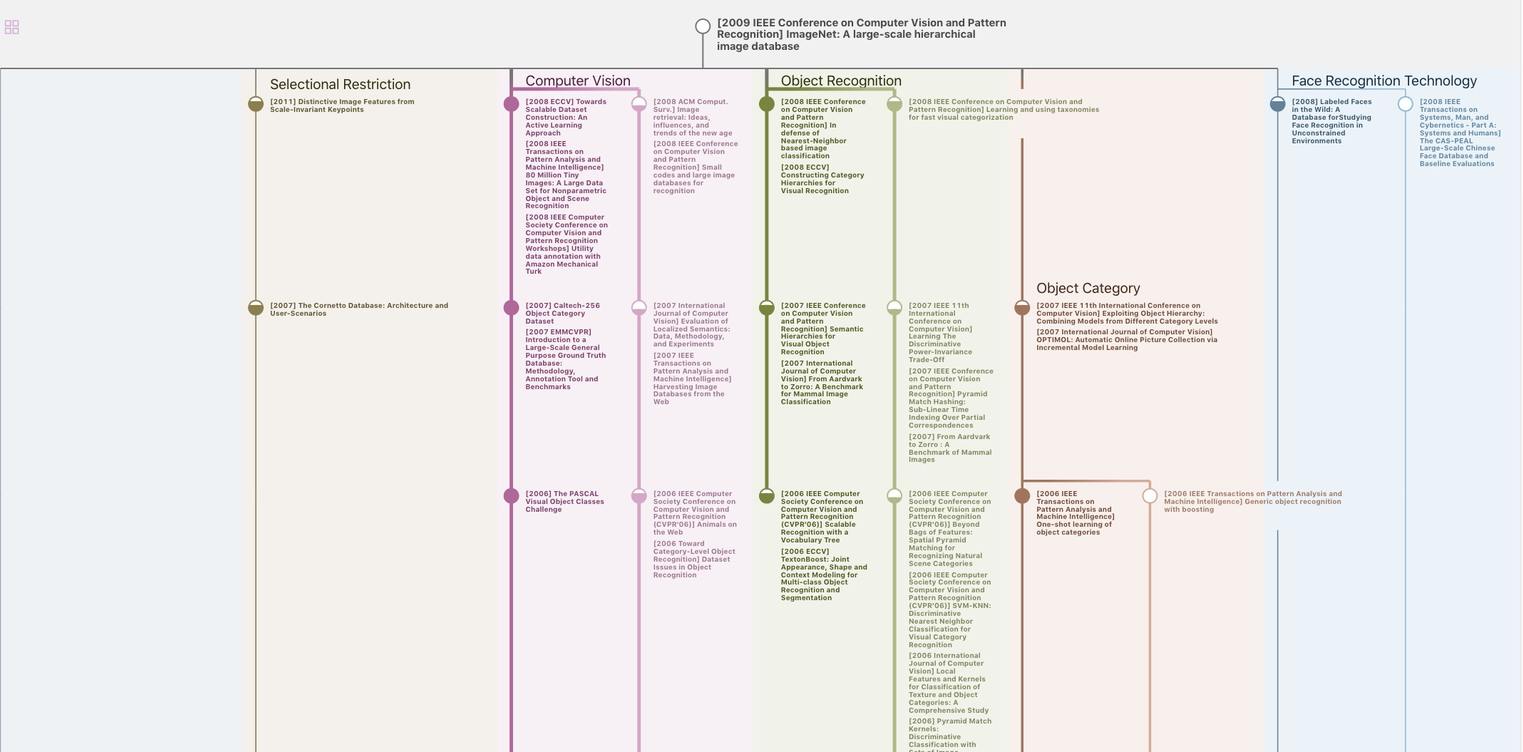
生成溯源树,研究论文发展脉络
Chat Paper
正在生成论文摘要