Measuring Strength of Joint Causal Effects
IEEE Transactions on Signal Processing(2024)
摘要
In the study of causality, we often seek not only to detect the presence of cause-effect relationships, but also to characterize how multiple causes combine to produce an effect. When the response to a change in one of the causes depends on the state of another cause, we say that there is an interaction or joint causation between the multiple causes. In this paper, we formalize a theory of joint causation based on higher-order derivatives and causal strength. Our proposed measure of joint causal strength is called the mixed differential causal effect (MDCE). We show that the MDCE approach can be naturally integrated into existing causal inference frameworks based on directed acyclic graphs or potential outcomes. We then derive a non-parametric estimator of the MDCE using Gaussian processes. We validate our approach with several experiments using synthetic data sets, demonstrating its applicability to static data as well as time series.
更多查看译文
关键词
causal effect,interaction,joint causality,Gaussian processes,nonlinear systems
AI 理解论文
溯源树
样例
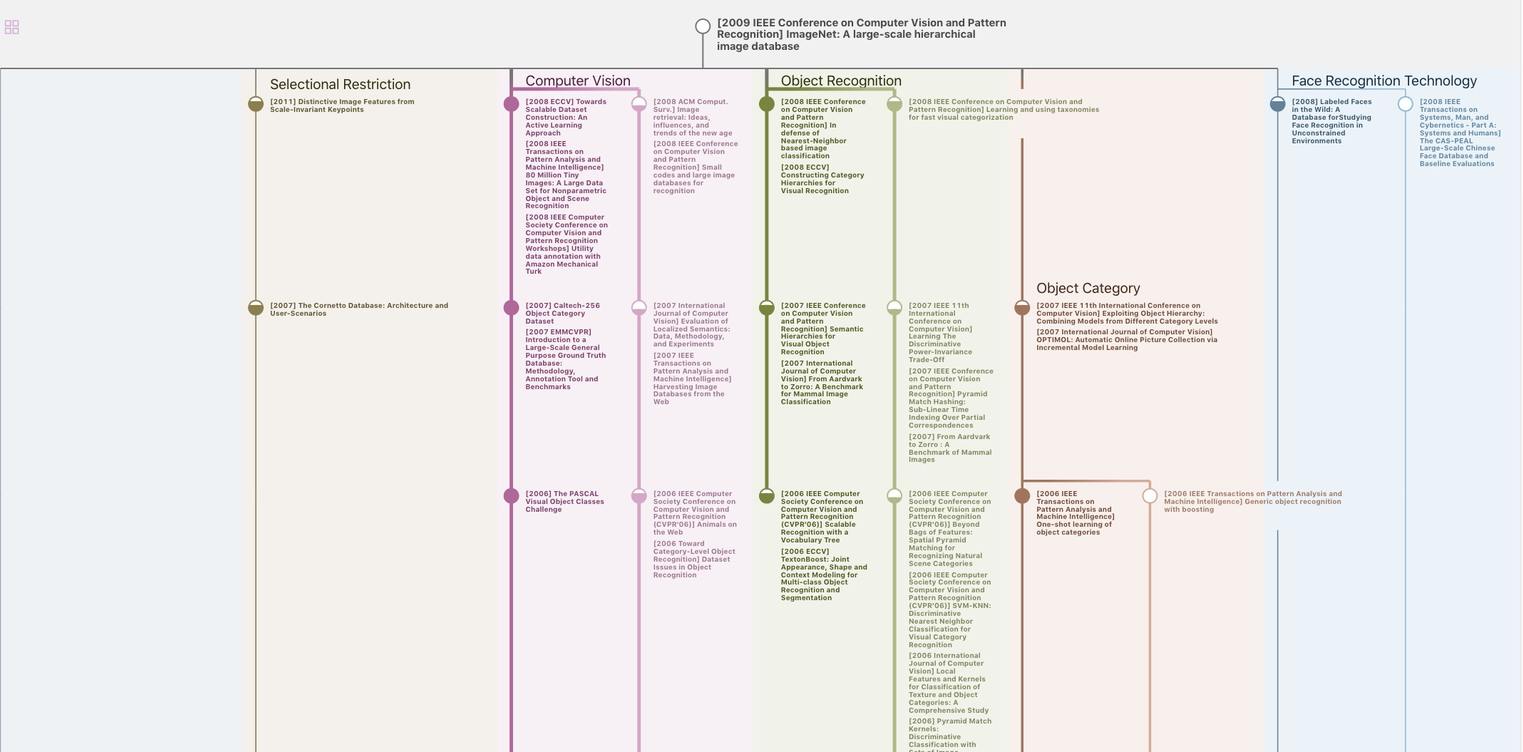
生成溯源树,研究论文发展脉络
Chat Paper
正在生成论文摘要