Improving Work-Nonwork Balance with Data-Driven Implementation Intention and Mental Contrasting
Proceedings of the ACM on Human-Computer Interaction(2024)
摘要
Work-nonwork balance is an important aspect of workplace well-being with associations to improved physical and mental health, job performance, and quality of life. However, realizing work-nonwork balance goals is challenging due to competing demands and limited resources within organizational and interpersonal contexts. These challenges are compounded by technologies that blur the boundaries of work and nonwork in the always-on work cultures. At an individual level, such challenges can be subsided through the effective application of self-regulation techniques, such as implementation intentions and mental contrasting (IIMC). Further supporting these techniques through reflection on personal data, we implement the idea of data-driven IIMC into a self-tracking and behavior planning system and evaluate it in a three-week between-participant study with 43 information workers who used our system for improving work-nonwork balance. We find evidence that reflection on personal data improves awareness of behavior plan compliance and rescheduling, which are important in realizing work-nonwork balance goals. We also observe the value of micro-reflection, reflection on limited data of the very recent past, for IIMC. Our findings highlight opportunities for automation in data collection and sense-making and for further exploring the role of data-driven IIMC as boundary negotiating artifacts in support of work-nonwork balance goals.
更多查看译文
AI 理解论文
溯源树
样例
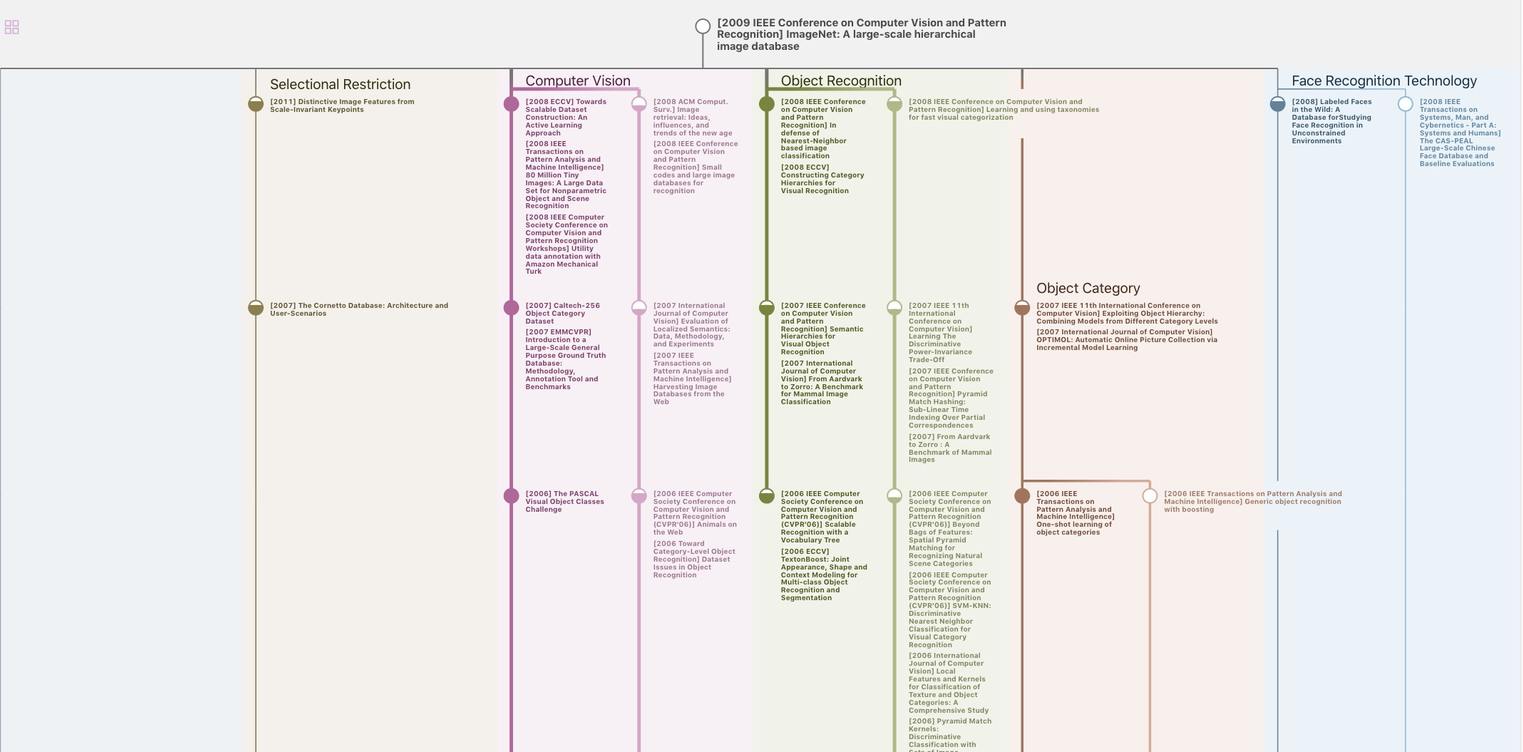
生成溯源树,研究论文发展脉络
Chat Paper
正在生成论文摘要