Explaining Siamese networks in few-shot learning
Machine Learning(2024)
摘要
Machine learning models often struggle to generalize accurately when tested on new class distributions that were not present in their training data. This is a significant challenge for real-world applications that require quick adaptation without the need for retraining. To address this issue, few-shot learning frameworks, which includes models such as Siamese Networks, have been proposed. Siamese Networks learn similarity between pairs of records through a metric that can be easily extended to new, unseen classes. However, these systems lack interpretability, which can hinder their use in certain applications. To address this, we propose a data-agnostic method to explain the outcomes of Siamese Networks in the context of few-shot learning. Our explanation method is based on a post-hoc perturbation-based procedure that evaluates the contribution of individual input features to the final outcome. As such, it falls under the category of post-hoc explanation methods. We present two variants, one that considers each input feature independently, and another that evaluates the interplay between features. Additionally, we propose two perturbation procedures to evaluate feature contributions. Qualitative and quantitative results demonstrate that our method is able to identify highly discriminant intra-class and inter-class characteristics, as well as predictive behaviors that lead to misclassification by relying on incorrect features.
更多查看译文
关键词
Explainable artificial intelligence,Interpretable machine learning,Siamese networks,Few-shot learning,Data-agnostic explanations
AI 理解论文
溯源树
样例
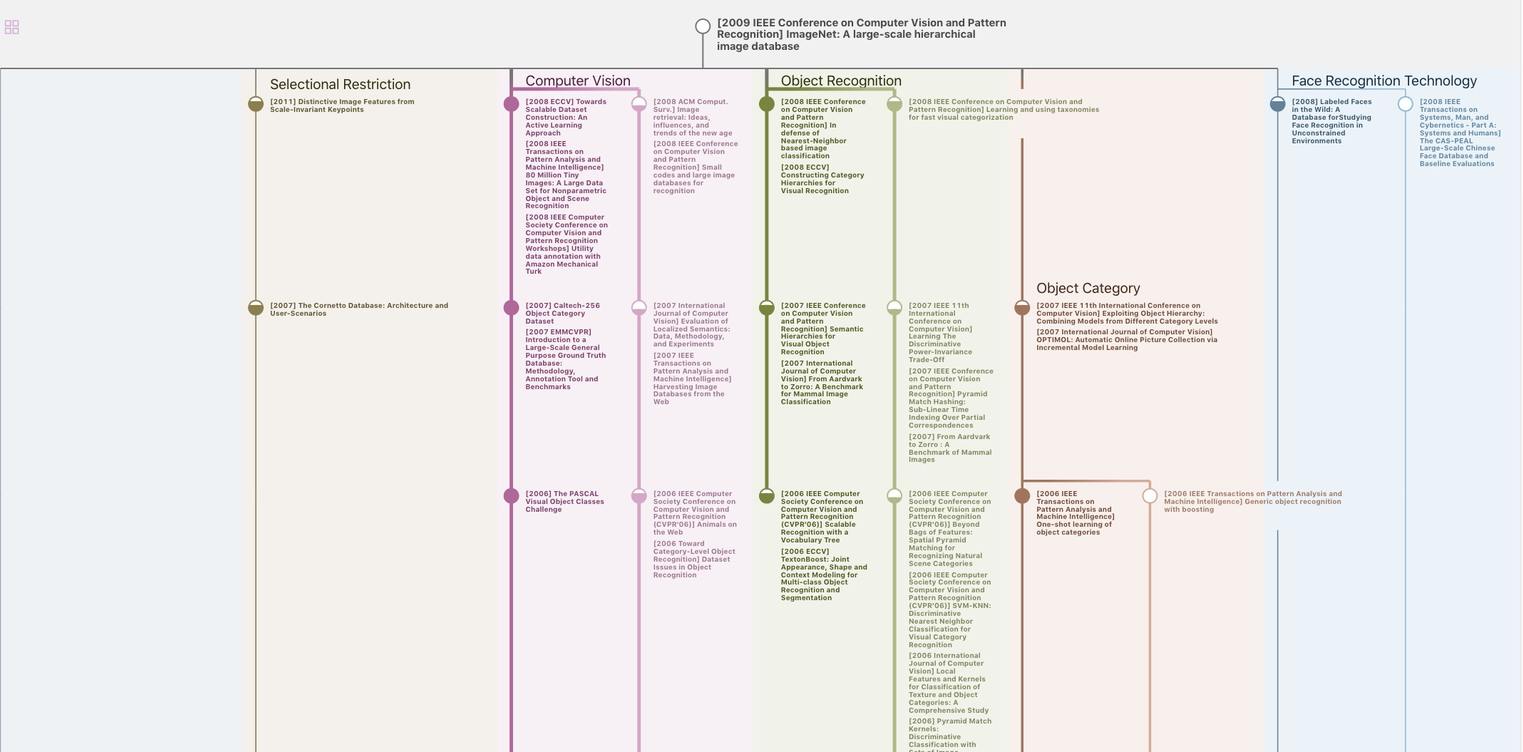
生成溯源树,研究论文发展脉络
Chat Paper
正在生成论文摘要