Leveraging Bilateral Correlations for Multi-Label Few-Shot Learning
IEEE TRANSACTIONS ON NEURAL NETWORKS AND LEARNING SYSTEMS(2024)
摘要
Multi-label few-shot learning (ML-FSL) refers to the task of tagging previously unseen images with a set of relevant labels, giving a small number of training examples. Modeling the correlations between instances and labels, formulated in the existing methods, allows us to extract more available knowledge from limited examples. However, they simply explore the instance and label correlations with a uniform importance assumption without considering the discrepancy of importance in different instances or labels, making the utilization of instance and label correlations a bottleneck for ML-FSL. To tackle the issue, we propose a unified framework named bilateral correlation reconstruction (BCR) to enable the network to effectively mine underlying instance and label correlations with varying importance information from both instance-to-label and label-to-instance perspectives. Specifically, from the instance-to-label perspective, we refine prototypes per category by reweighting each image with its specific instance-importance degree extracted from the similarity between the instance and the corresponding category. From the label-to-instance perspective, we smooth labels for each image by recovering latent label-importance with considering the integrated topology of all samples in a task. Experimental results on multiple benchmarks validate that BCR could outperform existing ML-FSL methods by large margins.
更多查看译文
关键词
Correlation,Prototypes,Task analysis,Image reconstruction,Vectors,Training,Cows,Few-shot learning,label correlation,multi-label few-shot learning (ML-FSL),multi-label learning (MLL)
AI 理解论文
溯源树
样例
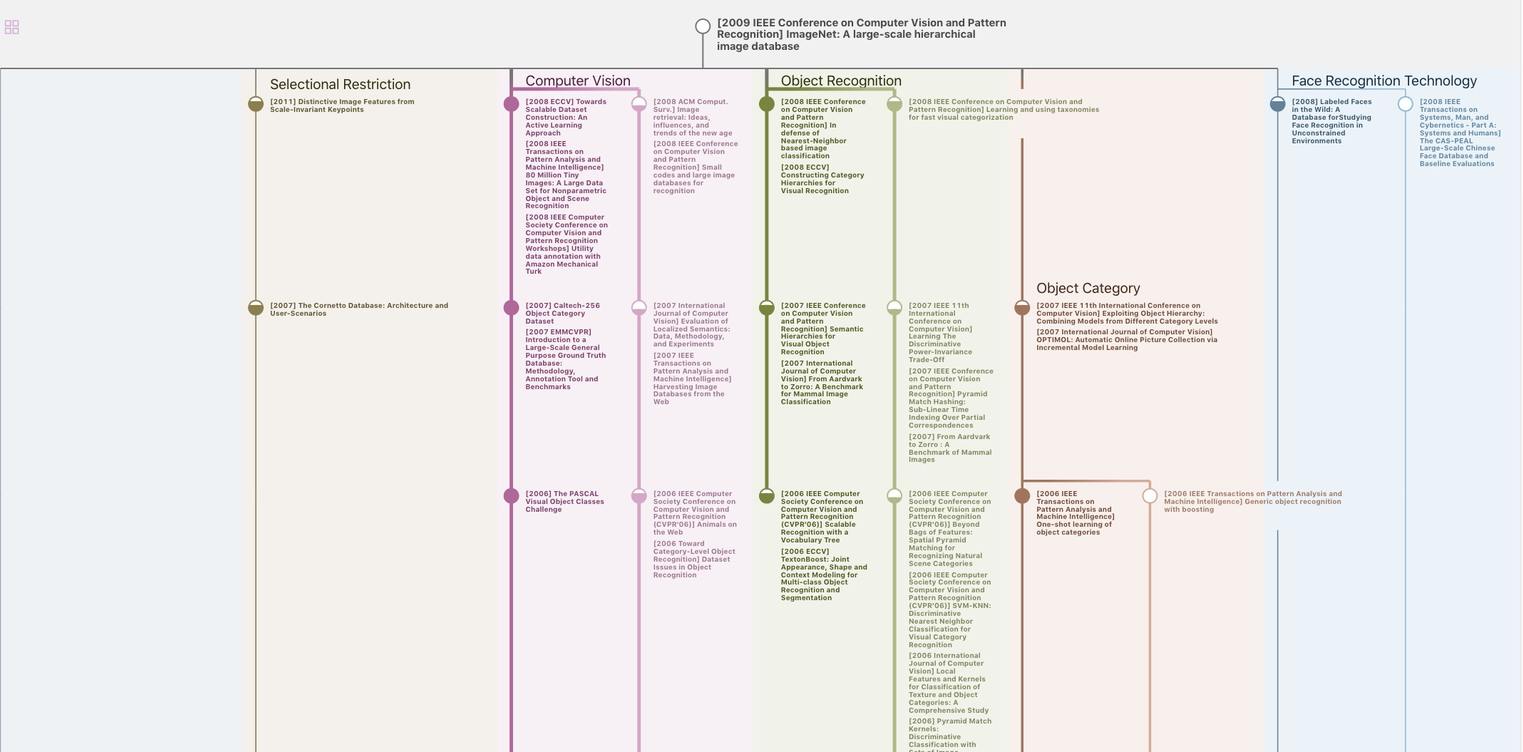
生成溯源树,研究论文发展脉络
Chat Paper
正在生成论文摘要