Multilayer Correlation Clustering
arxiv(2024)
摘要
In this paper, we establish Multilayer Correlation Clustering, a novel
generalization of Correlation Clustering (Bansal et al., FOCS '02) to the
multilayer setting. In this model, we are given a series of inputs of
Correlation Clustering (called layers) over the common set V. The goal is
then to find a clustering of V that minimizes the ℓ_p-norm (p≥ 1)
of the disagreements vector, which is defined as the vector (with dimension
equal to the number of layers), each element of which represents the
disagreements of the clustering on the corresponding layer. For this
generalization, we first design an O(Llog n)-approximation algorithm, where
L is the number of layers, based on the well-known region growing technique.
We then study an important special case of our problem, namely the problem with
the probability constraint. For this case, we first give an
(α+2)-approximation algorithm, where α is any possible
approximation ratio for the single-layer counterpart. For instance, we can take
α=2.5 in general (Ailon et al., JACM '08) and α=1.73+ϵ for
the unweighted case (Cohen-Addad et al., FOCS '23). Furthermore, we design a
4-approximation algorithm, which improves the above approximation ratio of
α+2=4.5 for the general probability-constraint case. Computational
experiments using real-world datasets demonstrate the effectiveness of our
proposed algorithms.
更多查看译文
AI 理解论文
溯源树
样例
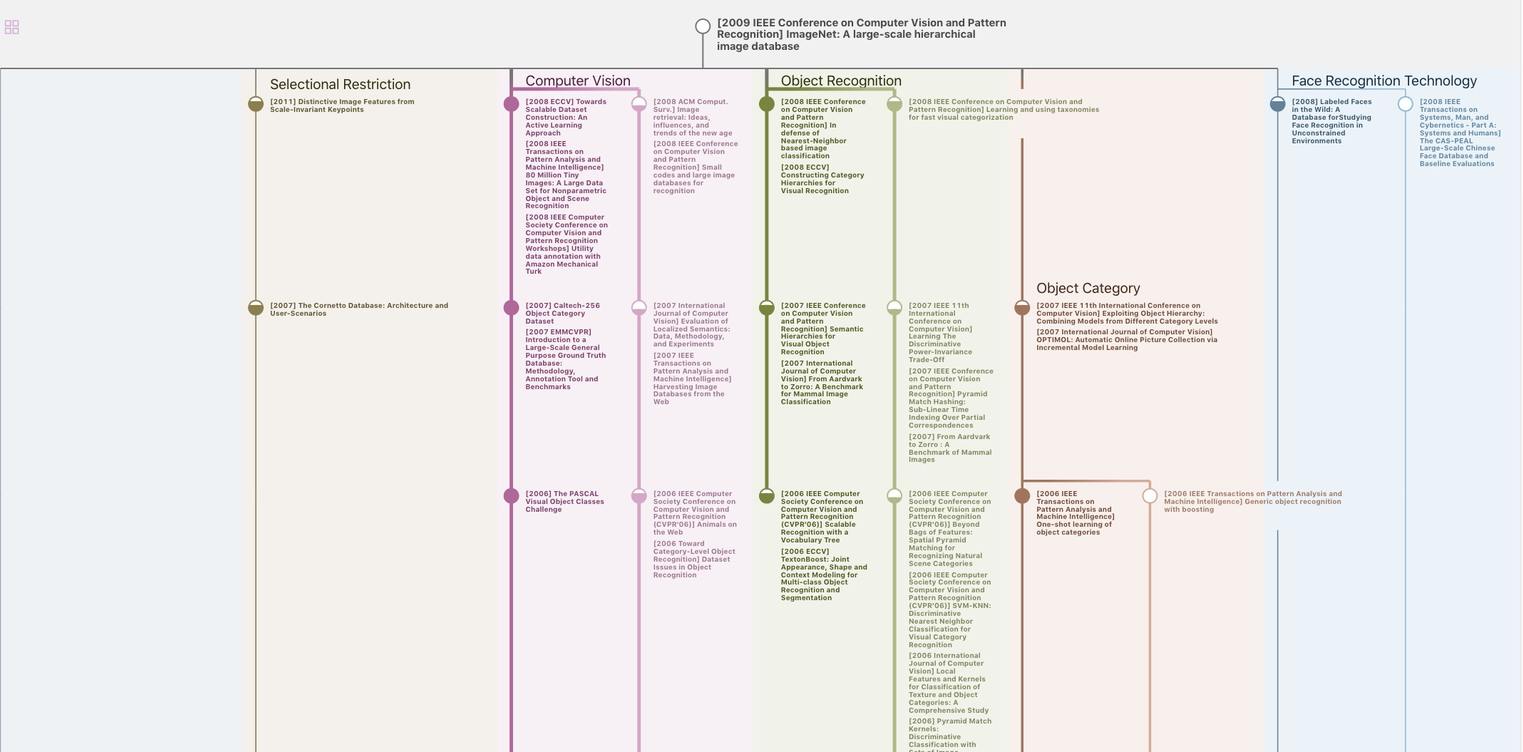
生成溯源树,研究论文发展脉络
Chat Paper
正在生成论文摘要