Balanced Unfolding Induced Tensor Nuclear Norms for High-Order Tensor Completion
IEEE TRANSACTIONS ON NEURAL NETWORKS AND LEARNING SYSTEMS(2024)
摘要
The recently proposed tensor tubal rank has been witnessed to obtain extraordinary success in real-world tensor data completion. However, existing works usually fix the transform orientation along the third mode and may fail to turn multidimensional low-tubal-rank structure into account. To alleviate these bottlenecks, we introduce two unfolding induced tensor nuclear norms (TNNs) for the tensor completion (TC) problem, which naturally extends tensor tubal rank to high-order data. Specifically, we show how multidimensional low-tubal-rank structure can be captured by utilizing a novel balanced unfolding strategy, upon which two TNNs, namely, overlapped TNN (OTNN) and latent TNN (LTNN), are developed. We also show the immediate relationship between the tubal rank of unfolding tensor and the existing tensor network (TN) rank, e.g., CANDECOMP/PARAFAC (CP) rank, Tucker rank, and tensor ring (TR) rank, to demonstrate its efficiency and practicality. Two efficient TC models are then proposed with theoretical guarantees by analyzing a unified nonasymptotic upper bound. To solve optimization problems, we develop two alternating direction methods of multipliers (ADMM) based algorithms. The proposed models have been demonstrated to exhibit superior performance based on experimental findings involving synthetic and real-world tensors, including facial images, light field images, and video sequences.
更多查看译文
关键词
High-order tensor recovery,low-rank tensor completion (LRTC),tensor decomposition,tensor nuclear norm (TNN),tensor singular value decomposition (t-SVD)
AI 理解论文
溯源树
样例
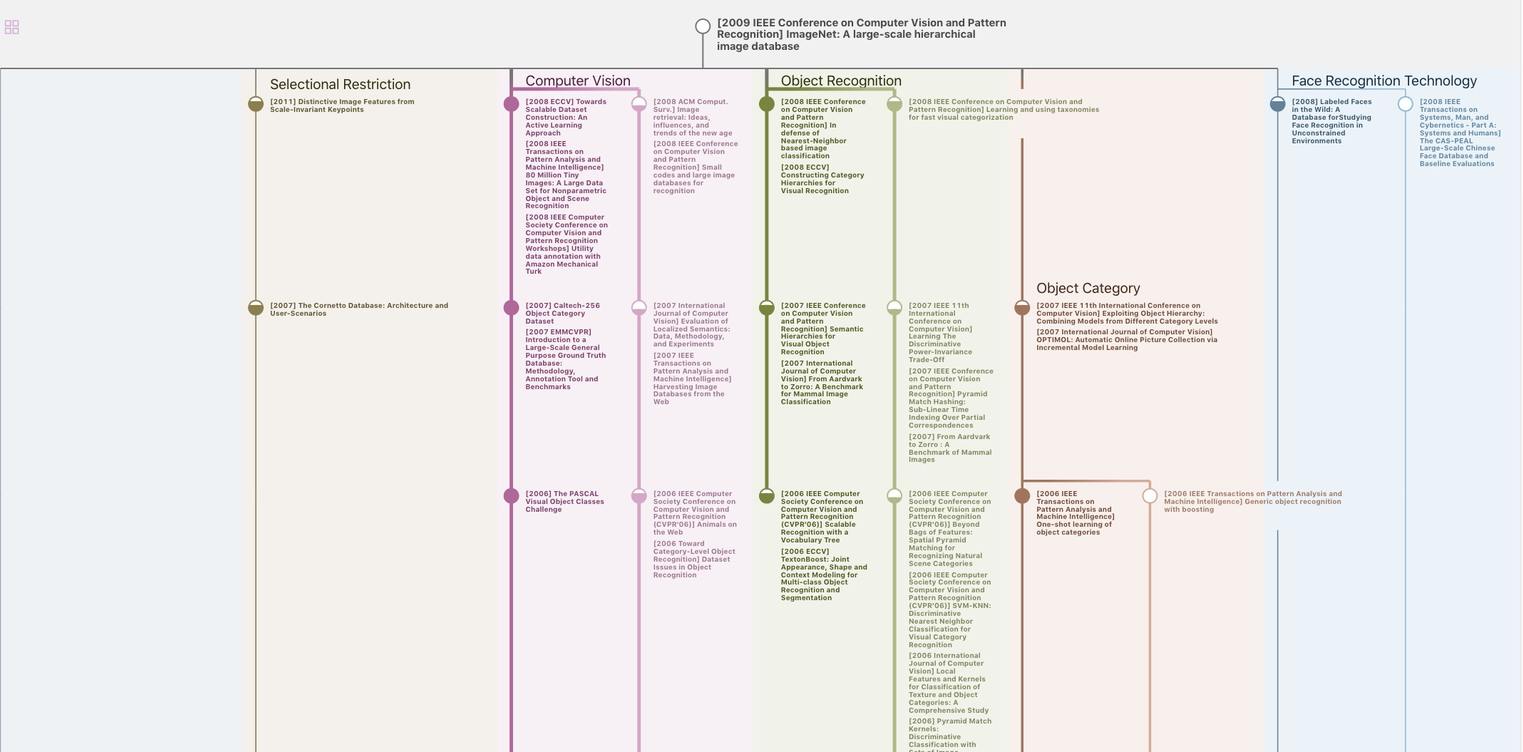
生成溯源树,研究论文发展脉络
Chat Paper
正在生成论文摘要