Optimal PMU Placement for Fault Classification and Localization Using Enhanced Feature Selection in Machine Learning Algorithms
International Journal of Energy Research(2024)
摘要
Machine learning (ML) algorithms are increasingly used in power systems applications. One important application is the classification and localization of various types of transmission line faults. Using voltage and current measurements from phasor measurement units (PMUs), a number of useful features can be extracted, which can form the basis of a ML-based prediction of the fault type, line, and distance on the line. This paper proposes a technique to find the optimal number and placement of PMUs by performing thorough feature selection. The features are selected to maximize the accuracy of the ML classification and regression algorithms. The results show that for the IEEE 14 bus system, the use of only five PMUs is sufficient to obtain high levels of accuracy. For example, a testing accuracy of 99.0% and 97.1% can be achieved for the fault type and fault line location, respectively. As for the fault distance along the line, the testing MAE of 3.1% can be obtained along with an R2 score of 94.4%. Adding more PMUs does not provide any additional value in terms of accuracy.
更多查看译文
AI 理解论文
溯源树
样例
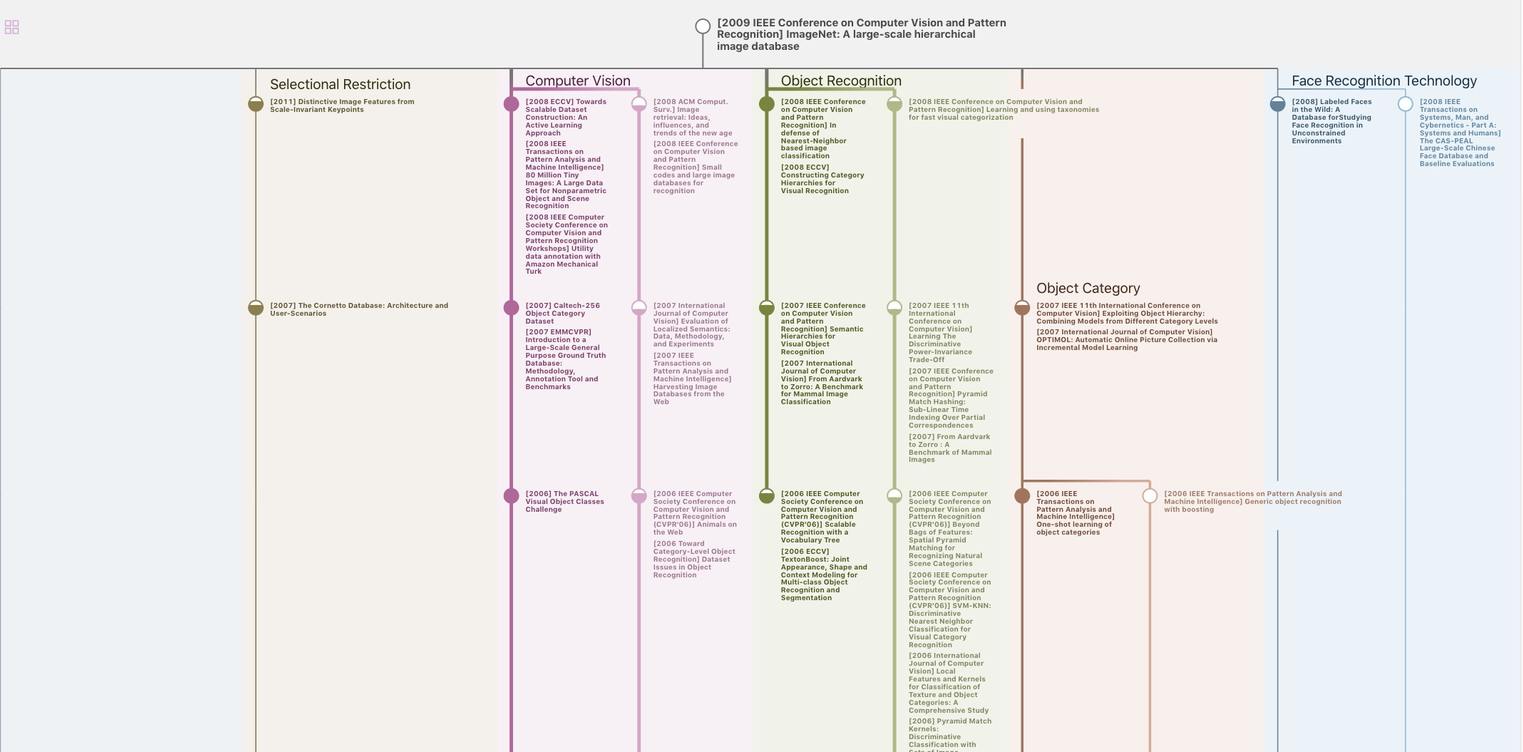
生成溯源树,研究论文发展脉络
Chat Paper
正在生成论文摘要