Auto robust relative radiometric normalization via latent change noise modeling
IEEE Transactions on Geoscience and Remote Sensing(2024)
摘要
Relative radiometric normalization (RRN) of different satellite images of the same terrain is essential for change detection, object classification/segmentation, and mapmaking tasks. However, identifying increasingly comprehensive no-change points for better normalization generalization is crucial. Traditional RRN models struggle to robustly derive the complete set of no-change points, often being disrupted by object changes. Additionally, RRN models that account for object changes precisely may require human involvement and instructions. This paper proposes automated and robust relative radiometric normalization methods through latent change noise modeling. They capitalize on the observation that no-change points exhibit small-scale noise under relative radiometric normalization, while change points exhibit large-scale noise after normalization. The proposed model benefits from a strong theoretical foundation grounded in probabilistic theory and mathematical deduction. Specifically, we integrate histogram matching with a mixture of Gaussian noise (HM-RRN-MoG) as the relative radiometric normalization learning scheme. This model demonstrates effective performance and has been successfully applied to hundreds of image production tasks. HM-RRN-MoG has impressive nochange prediction accuracy (70%) and superior relative radiometric normalization performance compared to state-of-the-art models. Furthermore, it exhibits robust behavior against clouds and changes. Our method also offers a natural and valuable evaluation metric for RRN: the root mean square error within the no-change set. Applying the HM-RRN-MoG model to vegetation/water change detection reduces radiometric contrast and normalized difference vegetation index (NDVI)/ normalized difference water index (NDWI) differences within the no-change set, leading to more consistent and comparable results. Similarly, in the building change detection task, utilizing the no-change set effectively reduces pseudo-changes and boosts precision. The code is available at https://github.com/647LiuSQ/HM-MoG-RRN/tree/main.
更多查看译文
关键词
Relative radiometric normalization,Change detection,Noise modeling,Histogram matching,Iteratively weighted least square regression,Stochastic expectation maximization
AI 理解论文
溯源树
样例
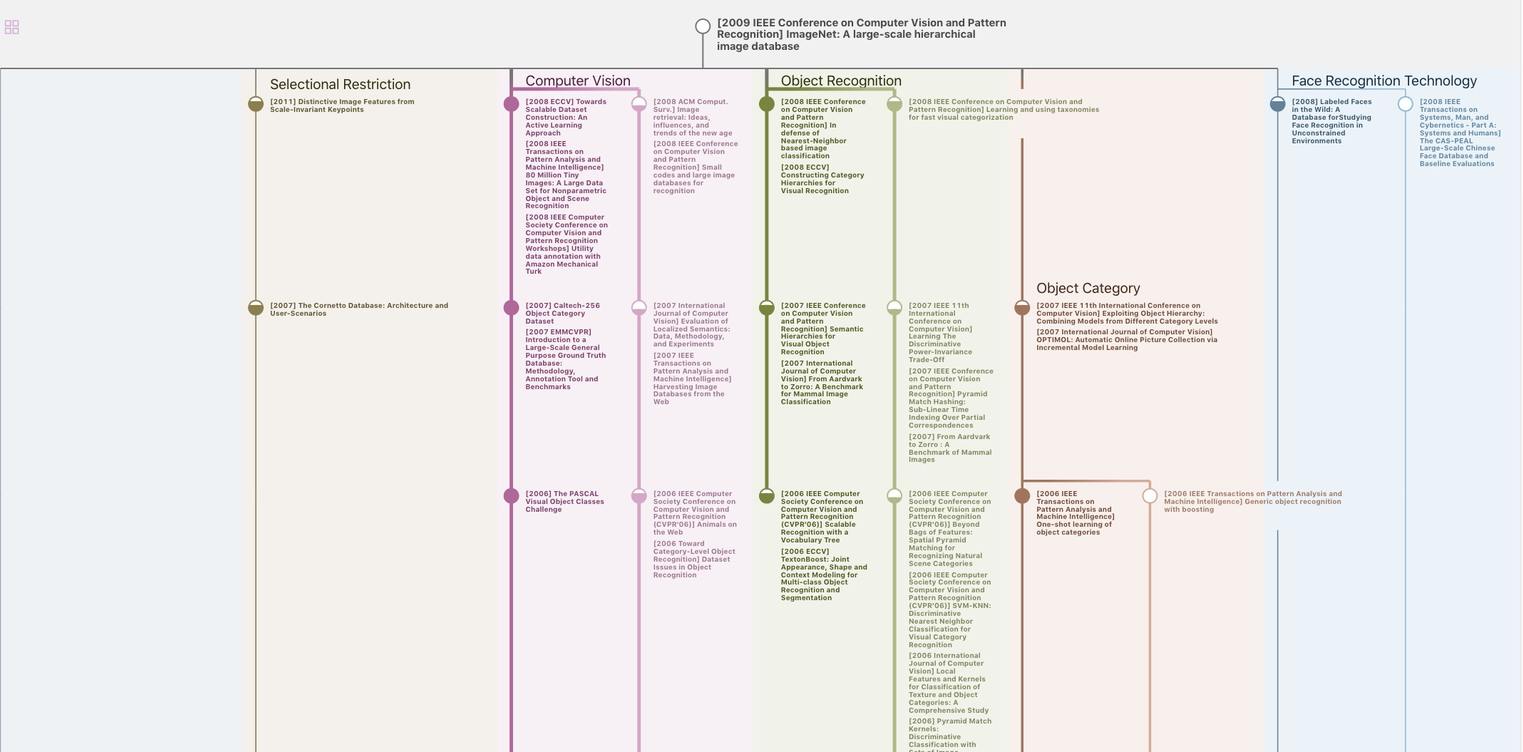
生成溯源树,研究论文发展脉络
Chat Paper
正在生成论文摘要