High-Order Neighbors Aware Representation Learning for Knowledge Graph Completion
IEEE TRANSACTIONS ON NEURAL NETWORKS AND LEARNING SYSTEMS(2024)
摘要
As a building block of knowledge acquisition, knowledge graph completion (KGC) aims at inferring missing facts in knowledge graphs (KGs) automatically. Previous studies mainly focus on graph convolutional network (GCN)-based KG embedding (KGE) to determine the representations of entities and relations, accordingly predicting missing triplets. However, most existing KGE methods suffer from limitations in predicting tail entities that are far away or even unreachable in KGs. This limitation can be attributed to the related high-order information being largely ignored. In this work, we focus on learning the information from the related high-order neighbors in KGs to improve the performance of prediction. Specifically, we first introduce a set of new nodes called pedal nodes to augment the KGs for facilitating message passing between related high-order entities, effectively injecting the information of high-order neighbors into entity representation. Additionally, we propose strength-guided graph neural networks to aggregate neighboring entity representations. To address the issue of transmitting irrelevant higher order information to entities through pedal nodes, which can potentially hurt entity representation, we further propose to dynamically integrate the aggregated representation of each node with its corresponding self-representation. Extensive experiments have been conducted on three benchmark datasets and the results demonstrate the superiority of our method compared to strong baseline models.
更多查看译文
关键词
Tail,Task analysis,Semantics,Message passing,Knowledge graphs,Convolutional neural networks,Training,Graph convolutional neural network,high-order neighbors,knowledge graph completion (KGC),representation learning
AI 理解论文
溯源树
样例
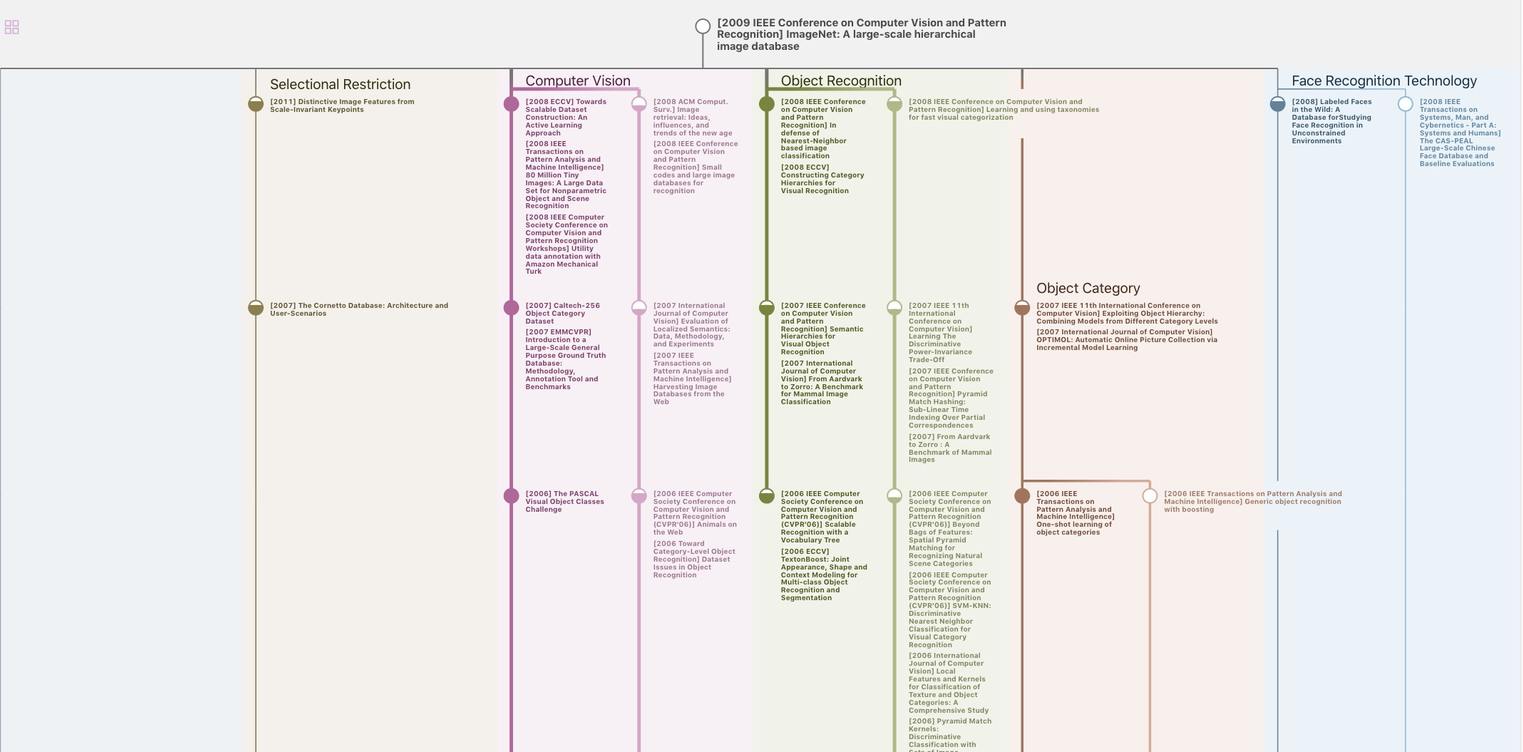
生成溯源树,研究论文发展脉络
Chat Paper
正在生成论文摘要