Beyond Collaborative Filtering: A Relook at Task Formulation in Recommender Systems
arxiv(2024)
摘要
Recommender Systems (RecSys) have become indispensable in numerous
applications, profoundly influencing our everyday experiences. Despite their
practical significance, academic research in RecSys often abstracts the
formulation of research tasks from real-world contexts, aiming for a clean
problem formulation and more generalizable findings. However, it is observed
that there is a lack of collective understanding in RecSys academic research.
The root of this issue may lie in the simplification of research task
definitions, and an overemphasis on modeling the decision outcomes rather than
the decision-making process. That is, we often conceptualize RecSys as the task
of predicting missing values in a static user-item interaction matrix, rather
than predicting a user's decision on the next interaction within a dynamic,
changing, and application-specific context. There exists a mismatch between the
inputs accessible to a model and the information available to users during
their decision-making process, yet the model is tasked to predict users'
decisions. While collaborative filtering is effective in learning general
preferences from historical records, it is crucial to also consider the dynamic
contextual factors in practical settings. Defining research tasks based on
application scenarios using domain-specific datasets may lead to more
insightful findings. Accordingly, viable solutions and effective evaluations
can emerge for different application scenarios.
更多查看译文
AI 理解论文
溯源树
样例
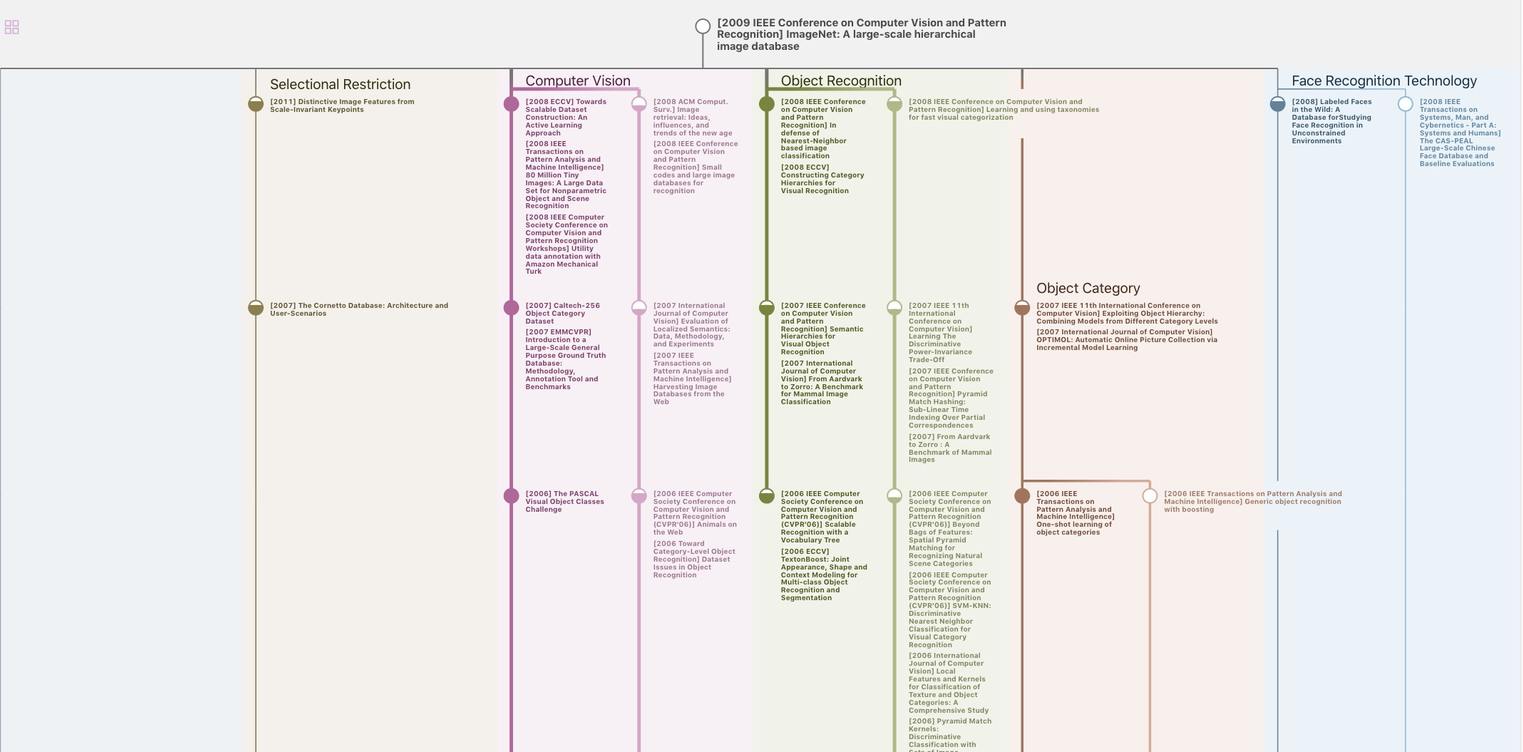
生成溯源树,研究论文发展脉络
Chat Paper
正在生成论文摘要