A Learning-to-Rank Formulation of Clustering-Based Approximate Nearest Neighbor Search
arxiv(2024)
摘要
A critical piece of the modern information retrieval puzzle is approximate
nearest neighbor search. Its objective is to return a set of k data points
that are closest to a query point, with its accuracy measured by the proportion
of exact nearest neighbors captured in the returned set. One popular approach
to this question is clustering: The indexing algorithm partitions data points
into non-overlapping subsets and represents each partition by a point such as
its centroid. The query processing algorithm first identifies the nearest
clusters – a process known as routing – then performs a nearest neighbor
search over those clusters only. In this work, we make a simple observation:
The routing function solves a ranking problem. Its quality can therefore be
assessed with a ranking metric, making the function amenable to
learning-to-rank. Interestingly, ground-truth is often freely available: Given
a query distribution in a top-k configuration, the ground-truth is the set of
clusters that contain the exact top-k vectors. We develop this insight and
apply it to Maximum Inner Product Search (MIPS). As we demonstrate empirically
on various datasets, learning a simple linear function consistently improves
the accuracy of clustering-based MIPS.
更多查看译文
AI 理解论文
溯源树
样例
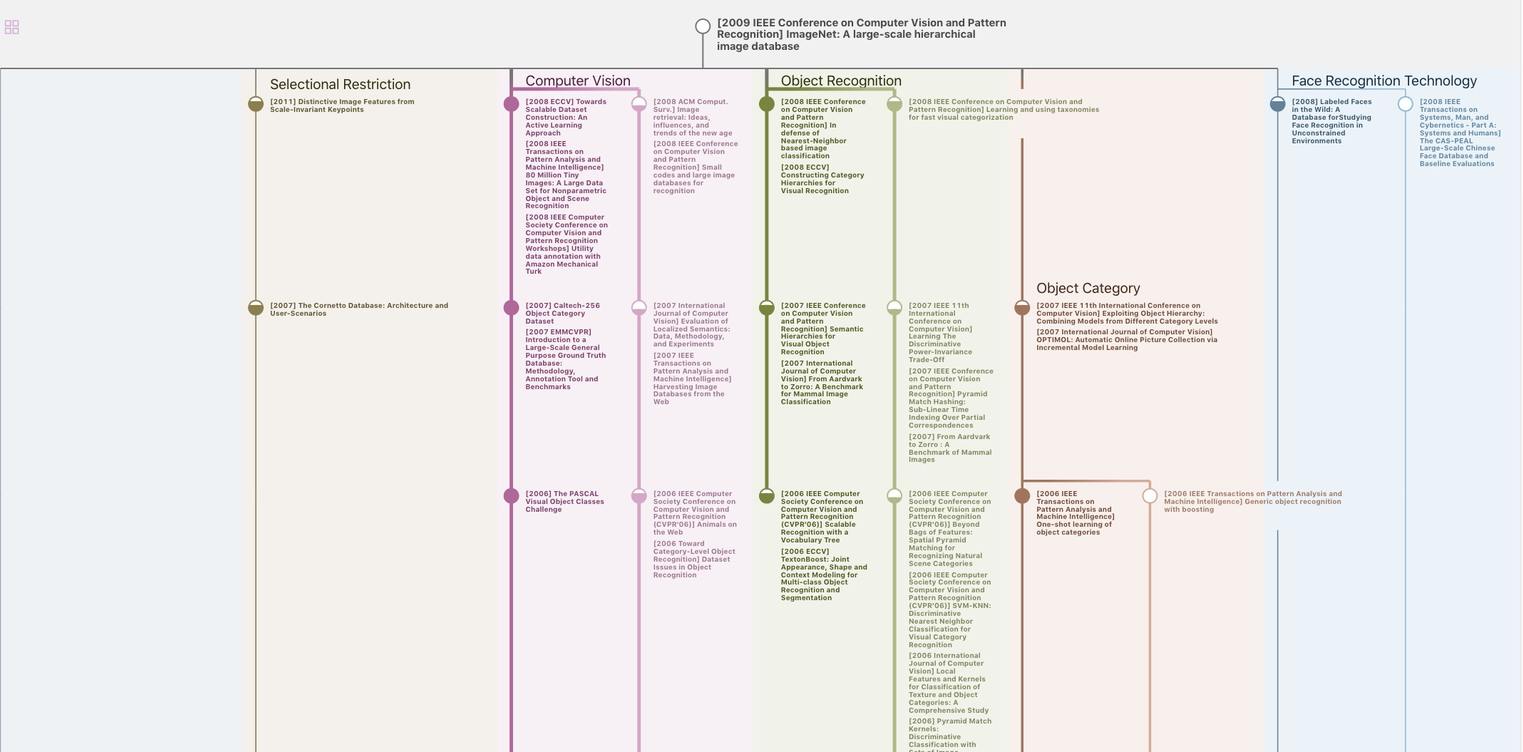
生成溯源树,研究论文发展脉络
Chat Paper
正在生成论文摘要