Automated machine learning: past, present and future
Artificial Intelligence Review(2024)
摘要
Automated machine learning (AutoML) is a young research area aiming at making high-performance machine learning techniques accessible to a broad set of users. This is achieved by identifying all design choices in creating a machine-learning model and addressing them automatically to generate performance-optimised models. In this article, we provide an extensive overview of the past and present, as well as future perspectives of AutoML. First, we introduce the concept of AutoML, formally define the problems it aims to solve and describe the three components underlying AutoML approaches: the search space, search strategy and performance evaluation. Next, we discuss hyperparameter optimisation (HPO) techniques commonly used in AutoML systems design, followed by providing an overview of the neural architecture search, a particular case of AutoML for automatically generating deep learning models. We further review and compare available AutoML systems. Finally, we provide a list of open challenges and future research directions. Overall, we offer a comprehensive overview for researchers and practitioners in the area of machine learning and provide a basis for further developments in AutoML.
更多查看译文
关键词
Automated machine learning,Neural architecture search,Hyperparameter optimisation,Search space,Search strategy
AI 理解论文
溯源树
样例
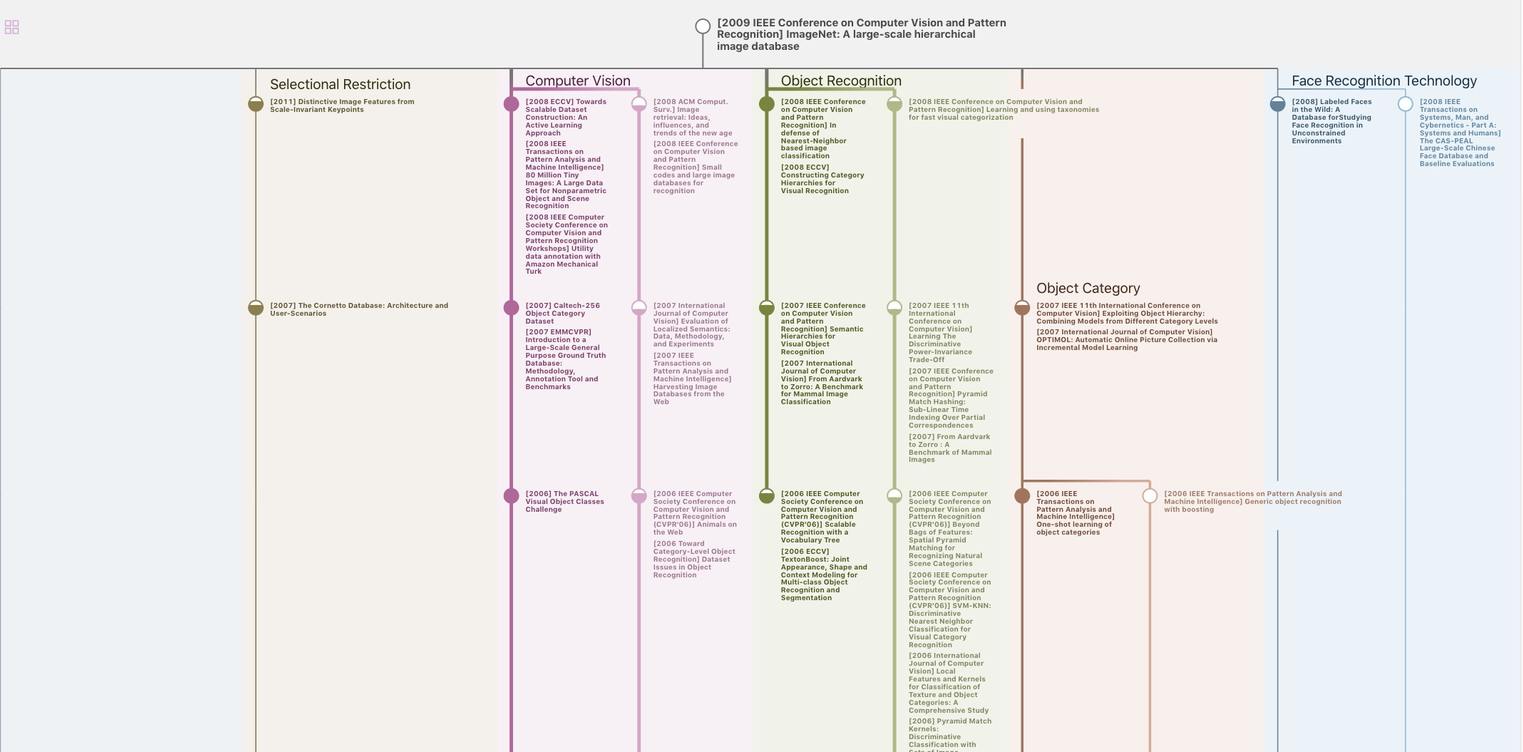
生成溯源树,研究论文发展脉络
Chat Paper
正在生成论文摘要