Introduction to the ACSAC’22 Special Issue
Digital Threats: Research and Practice(2024)
摘要
The Annual Computer Security Applications Conference (ACSAC) brings together cutting-edge researchers, with a broad cross-section of security professionals drawn from academia, industry, and government, gathered to present and discuss the latest security results and topics. ACSAC’s core mission is to investigate practical solutions for computer and network security technology. The 38th ACSAC was held in Austin, Texas from December 5-9, 2022. As in the previous year, ACSAC especially encouraged contributions on a hard topic theme, in this year in the area of Trustworthy Systems . Trustworthy systems generally involve the development of capabilities that offer security, safety, and reliability guarantees. ACSAC has always solicited work on applied security; with this hard topic, we put great emphasize on deployable trustworthy systems, including (but not limited to) approaches applied at the intersection of operation systems, formal methods, and programming languages; approaches applied at the architecture level; trustworthy artificial intelligence with emphasize on explainability, correctness, and robustness to attacks; zero-trust solutions that assume no implicit trust, but continually assess risk; and trustworthy systems form a user’s perspective. This topic does not necessarily mean building a complete solution, but identifying key challenges, explaining the deficiencies in state-of-the-art solutions, and demonstrating the effectiveness of the proposed approaches and (potential) impact to the real world. In addition, ACSAC continues to encourage authors of accepted papers to submit software and data artifacts and make them publicly available to the entire community. Releasing software and data artifacts represents an important step towards facilitating the reproducibility of research results, and ultimately contributes to the real-world deployment of novel security solutions. For this special issue we invited authors of papers that appeared at ACSAC 2022 and that successfully passed an evaluation of their software and/or data artifacts to submit an extended version of their papers. This selection criteria ensured that the research has a high potential for being deployed in real-world environments and to be used to implement practical defense systems. This volume contains three manuscripts on topics from three different areas: IoT security and privacy, adversarial machine learning, and backdoor attacks against federated learning. In “SPACELORD: Private and Secure Smart Space Sharing,” Bae et al. address security and privacy issues of smart devices when installed in shared spaces, such as vacation rentals and co-working meeting rooms. Their approach allows for securely time-sharing by transferring control and the configuration of devices to temporary users, as well as resetting devices and removing any private information when a user leaves a space. The authors extended their original solution with different hardware and software configurations, a discussion of alternative designs, compatibility with existing systems, and design limitations. In “Multi-SpacePhish: Extending the Evasion-space of Adversarial Attacks against Phishing Website Detectors using Machine Learning,” Yuan et al. systematically compare adversarial attacks against machine learning systems for the detection of phishing websites. They investigate how realistic different attacks are by performing 12 different attacks and considering different models, feature spaces, and datasets. The authors also formalize and compare evasion-spaces, e.g., perturbations in the problem-space with those in the feature-space. The authors extended their original work with additional experiments and considering more perturbations, as well as the definition and investigation of multi-space attacks considering attackers that introduce perturbations across spaces. In “Unveiling the Threat: Investigating Distributed and Centralized Backdoor Attacks in Federated Graph Neural Networks,” Xu et al. investigate two types of backdoor attacks against federated learning, in particular graph neural networks: centralized backdoor attacks and distributed backdoor attacks. The authors evaluate the performance of these attacks in different scenarios, as well as their resilience to two defense mechanisms. The authors extended their original experiments with two new datasets to explore attacks in real-world application scenarios, as well investigate the effectiveness of an additional defense mechanism. As Associate Editors for this special issue, we are very pleased that the authors of the above papers have significantly extended and improved their ACSAC’22 publications, and that they provide their artifacts to the public to foster the reproducibility of their research results. We wish to thank the authors, reviewers and ACSAC’22 program committee members who have contributed to selecting the papers that appear in this special issue. We would also like to thank the DTRAP Co-Editors in Chief and the ACM for the opportunity to work on this special issue.
更多查看译文
AI 理解论文
溯源树
样例
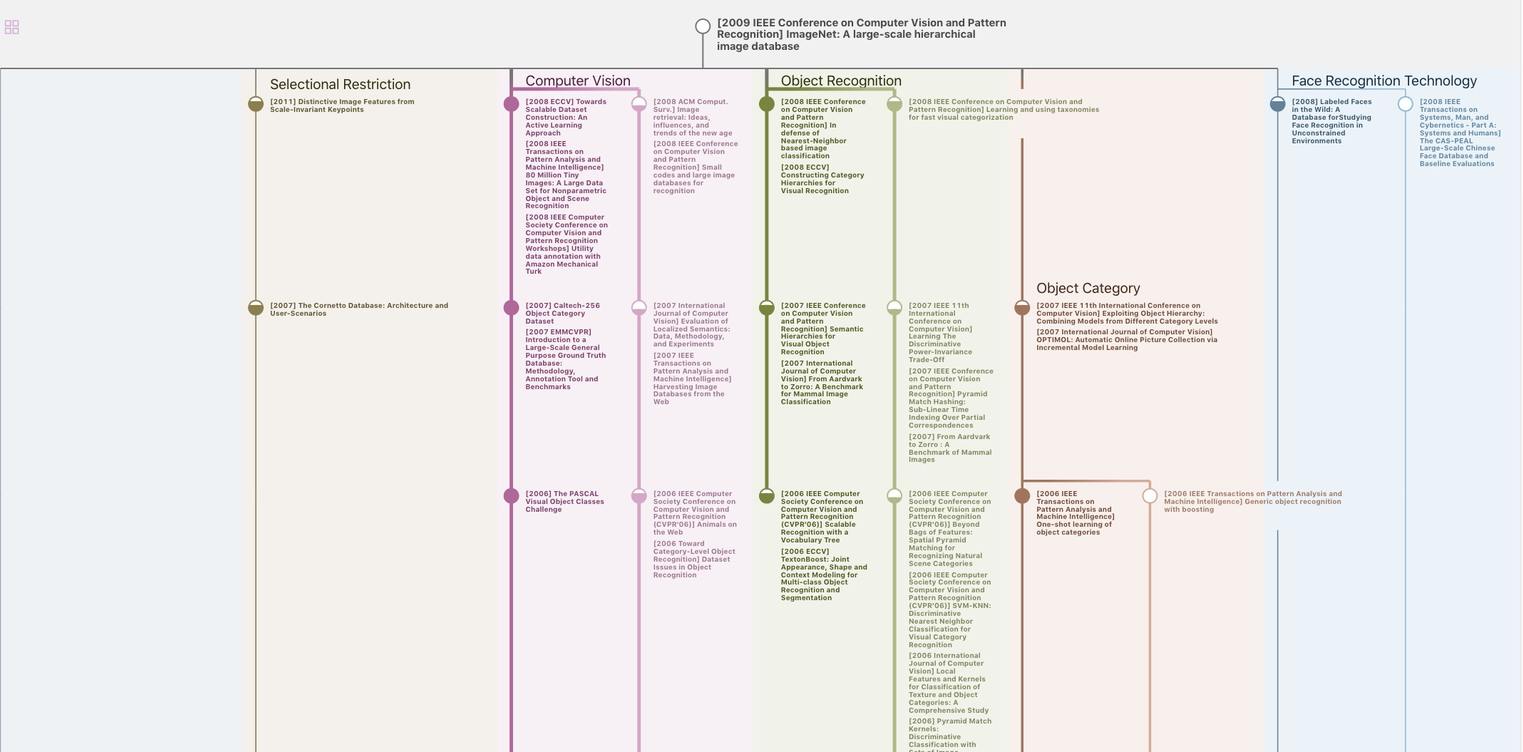
生成溯源树,研究论文发展脉络
Chat Paper
正在生成论文摘要