Entropic regularization of neural networks: Self-similar approximations
Journal of Statistical Planning and Inference(2024)
摘要
This paper focuses on entropic regularization and its multiscale extension in neural network learning. We leverage established results that characterize the optimizer of entropic regularization methods and their connection with generalization bounds. To avoid the significant computational complexity involved in sampling from the optimal multiscale Gibbs distributions, we describe how to make measured concessions in optimality by using self-similar approximating distributions. We study such scale-invariant approximations for linear neural networks and further extend the approximations to neural networks with nonlinear functions. We then illustrate the application of our proposed approach through empirical simulation. By navigating the interplay between optimization and computational efficiency, our research contributes to entropic regularization theory, proposing a practical method that embraces symmetry across scales.
更多查看译文
关键词
Machine learning,Information theory,Chaining,Gibbs distribution,Scale-invariance
AI 理解论文
溯源树
样例
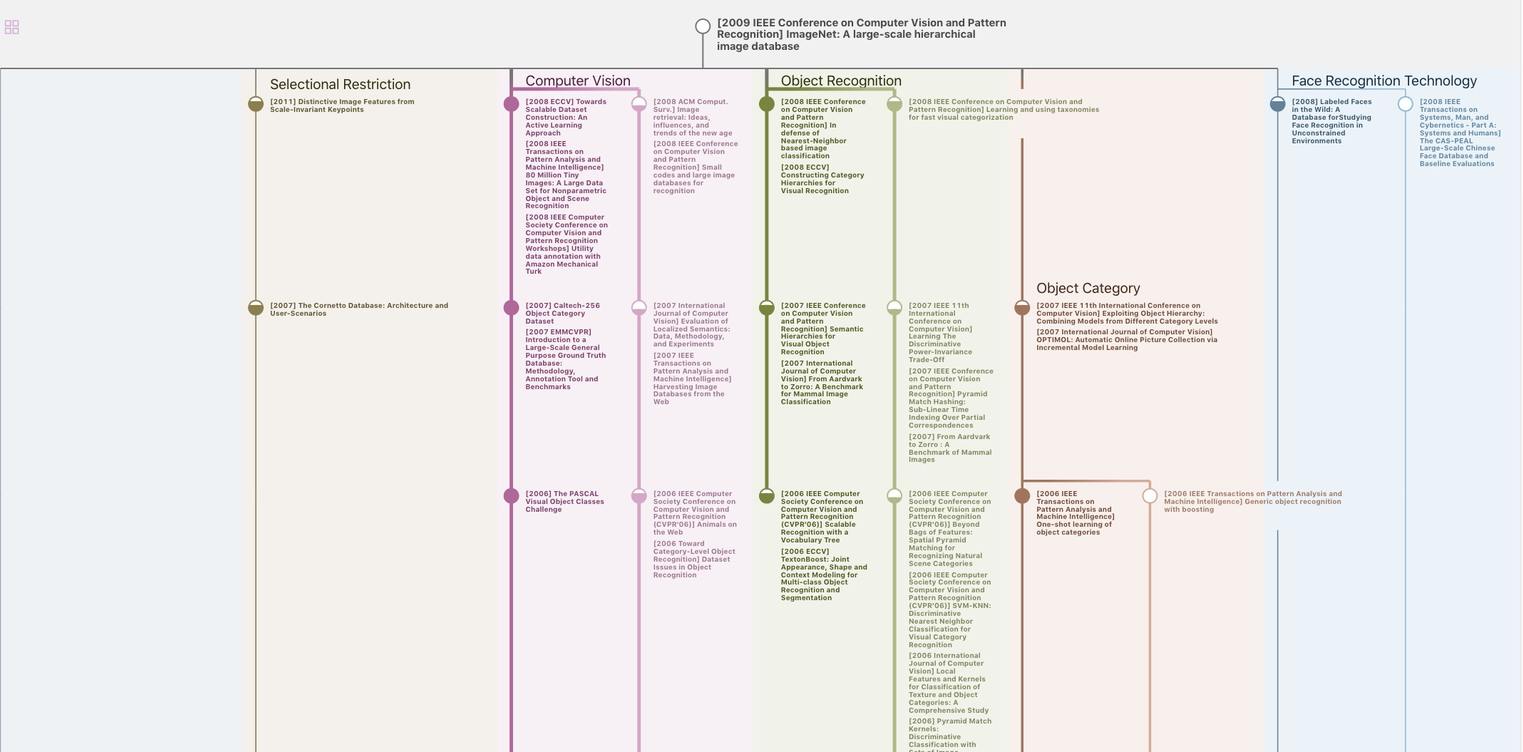
生成溯源树,研究论文发展脉络
Chat Paper
正在生成论文摘要