The Average Spectrum Norm and Near-Optimal Tensor Completion
arxiv(2024)
摘要
We introduce a new tensor norm, the average spectrum norm, to study sample
complexity of tensor completion problems based on the canonical polyadic
decomposition (CPD). Properties of the average spectrum norm and its dual norm
are investigated, demonstrating their utility for low-rank tensor recovery
analysis. Our novel approach significantly reduces the provable sample rate for
CPD-based noisy tensor completion, providing the best bounds to date on the
number of observed noisy entries required to produce an arbitrarily accurate
estimate of an underlying mean value tensor. Under Poisson and Bernoulli
multivariate distributions, we show that an N-way CPD rank-R parametric
tensor ℳ∈ℝ^I×⋯× I
generating noisy observations can be approximated by large likelihood
estimators from 𝒪(IR^2log^N+2(I)) revealed entries. Furthermore,
under nonnegative and orthogonal versions of the CPD we improve the result to
depend linearly on the rank, achieving the near-optimal rate
𝒪(IRlog^N+2(I)).
更多查看译文
AI 理解论文
溯源树
样例
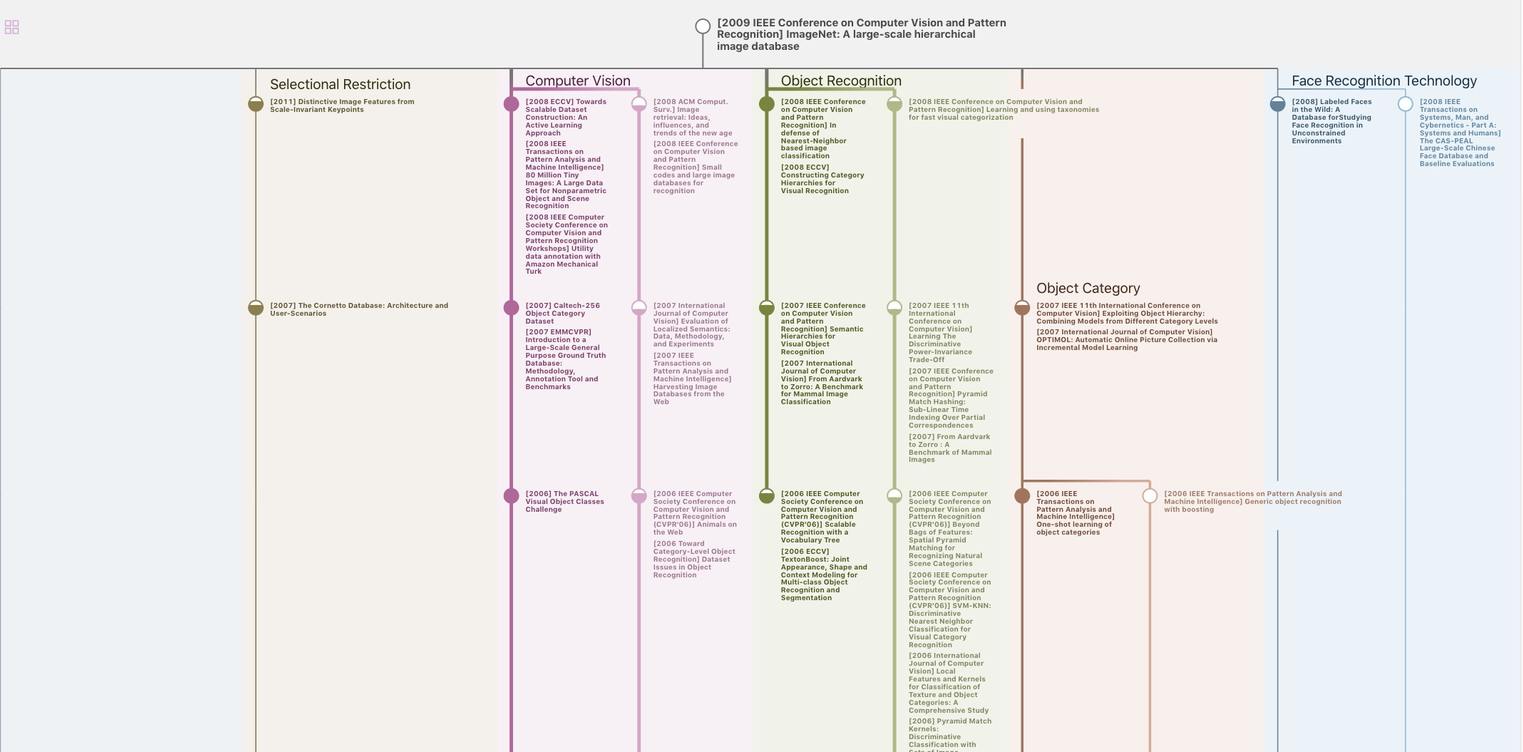
生成溯源树,研究论文发展脉络
Chat Paper
正在生成论文摘要