Optimizing Malware Detection in IoT Networks: Leveraging Resource-Aware Distributed Computing for Enhanced Security
CoRR(2024)
摘要
In recent years, networked IoT systems have revo- lutionized connectivity,
portability, and functionality, offering a myriad of advantages. However, these
systems are increasingly targeted by adversaries due to inherent security
vulnerabilities and limited computational and storage resources. Malicious
applications, commonly known as malware, pose a significant threat to IoT
devices and networks. While numerous malware detection techniques have been
proposed, existing approaches often overlook the resource constraints inherent
in IoT environ- ments, assuming abundant resources for detection tasks. This
oversight is compounded by ongoing workloads such as sens- ing and on-device
computations, further diminishing available resources for malware detection. To
address these challenges, we present a novel resource- and workload-aware
malware detection framework integrated with distributed computing for IoT
networks. Our approach begins by analyzing available resources for malware
detection using a lightweight regression model. Depending on resource
availability, ongoing workload executions, and communication costs, the malware
detection task is dynamically allocated either on-device or offloaded to
neighboring IoT nodes with sufficient resources. To safeguard data integrity
and user privacy, rather than transferring the entire malware detection task,
the classifier is partitioned and distributed across multiple nodes, and
subsequently integrated at the parent node for comprehensive malware detection.
Experimental analysis demonstrates the efficacy of our proposed technique,
achieving a remarkable speed-up of 9.8x compared to on-device inference, while
maintaining a high malware detection accuracy of 96.7
更多查看译文
AI 理解论文
溯源树
样例
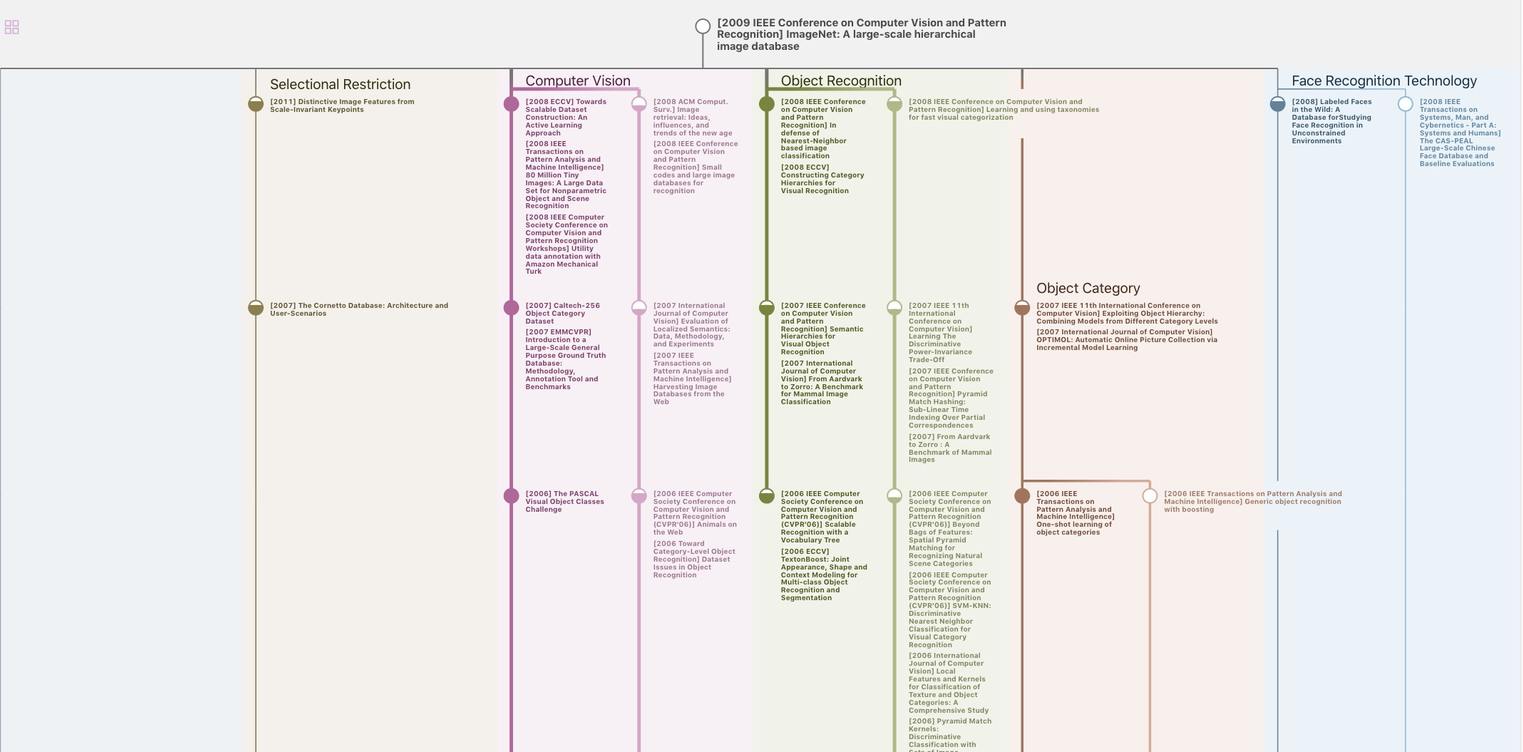
生成溯源树,研究论文发展脉络
Chat Paper
正在生成论文摘要