Advancing Paraphasia Detection with End-to-End Learning: A Comparative Approach Study (Preprint)
crossref(2024)
摘要
BACKGROUND Paraphasias are speech errors that are often characteristic of aphasia and they represent an important signal in assessing disease severity and subtype. Traditionally, clinicians manually identify paraphasias by transcribing and analyzing speech-language samples, which can be a time-consuming and burdensome process. Automatic paraphasia detection can greatly help clinicians with the transcription process and ultimately facilitate more efficient and consistent aphasia assessment. OBJECTIVE This study investigates a novel machine learning framework for automatic paraphasia detection that is trained end-to-end (i.e., a unified network that takes speech audio as input and outputs text that indicates what was said and identifies which words are paraphasias). We use the AphasiaBank corpus, which contains audio data collected from persons with aphasia (PWAs) that has been transcribed and labeled with paraphasias by trained speech-language pathologists. METHODS We propose a novel sequence-to-sequence (seq2seq) architecture for performing both automatic speech recognition (ASR) and paraphasia detection tasks. We explore the impact of leveraging pretrained speech models as well as different learning objectives for optimizing this model. This approach can be advantageous in learning synergistic representations that benefit both ASR and paraphasia detection tasks. We compare against a previous state-of-the art method that uses a multi-step pipeline approach consisting of ASR, hand-engineered feature extraction, and paraphasia detection. RESULTS We show that the proposed seq2seq is able to outperform the multi-step pipeline approach for word-level and utterance-level paraphasia detection. We achieve word-level performance improvements of 16.9%, 36.4%, and 9.5% and utterance-level improvements of 5.2%, 13.9%, 18.9% for phonemic, neologistic, and phonemic+neologistic paraphasias, respectively. CONCLUSIONS These results highlight the performance improvements of learning to detect paraphasias end-to-end rather than through a multi-step pipeline approach with separate ASR and paraphasia detection models. The advantage of learning both ASR and paraphasia detection tasks end-to-end is that this unified model can learn joint representations that are beneficial to both ASR and paraphasia detection tasks rather than optimizing both of these separately. Future work will explore the efficacy of a deployed paraphasia detection model at assisting medical professionals with annotation.
更多查看译文
AI 理解论文
溯源树
样例
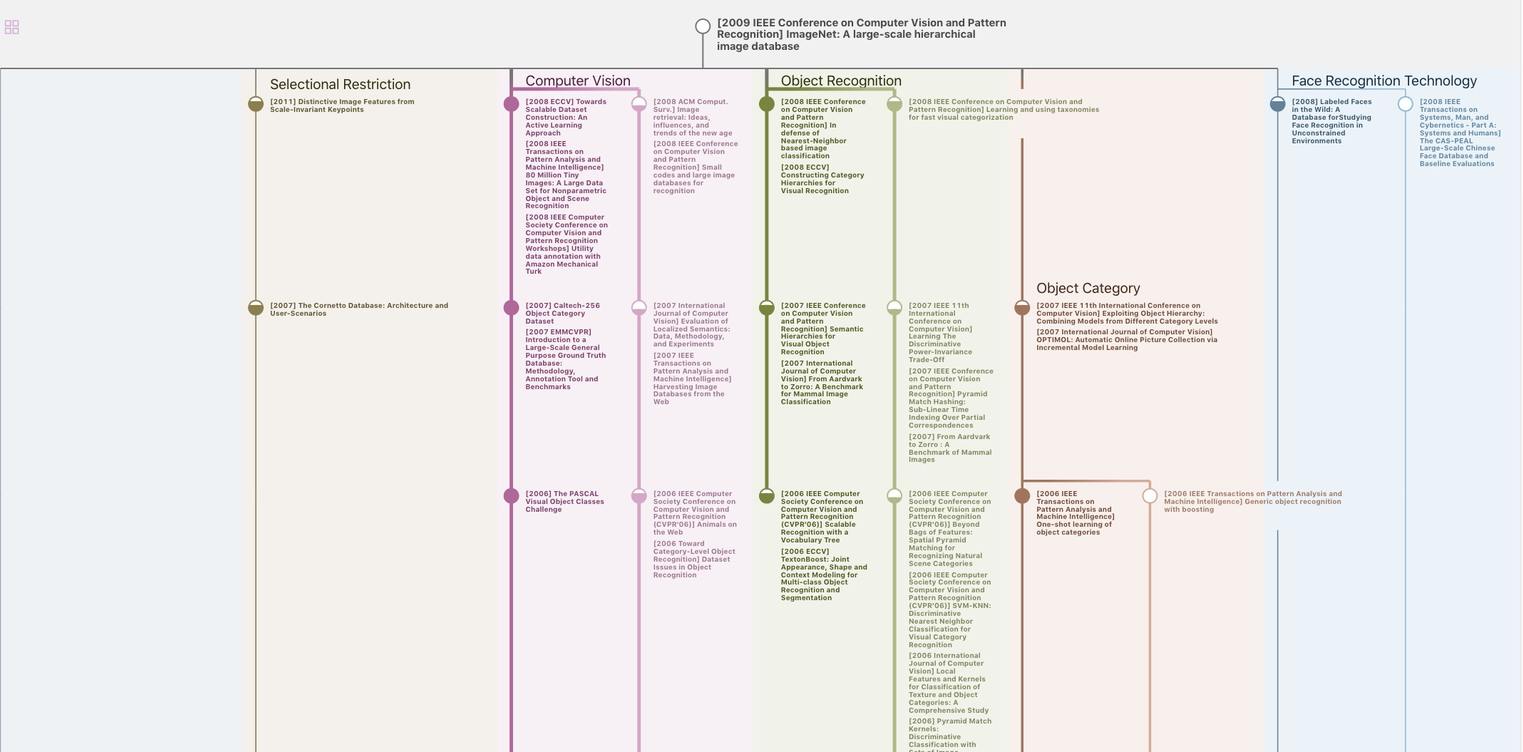
生成溯源树,研究论文发展脉络
Chat Paper
正在生成论文摘要