An attack-agnostic defense method against adversarial attacks on speaker verification by fusing downsampling and upsampling of speech signals
Information Sciences(2024)
摘要
With the advance of deep learning, adversarial attack and defense has becoming a hot research topic. However, existing defense methods rely on the prior knowledge of the adversarial attacks, and are also vulnerable to adaptive attacks. In this paper, towards a secure automatic speaker verification system, a novel attack-agnostic defense method is proposed, named by SampleShield, which consists of a pairwise random downsampling (PRD) module and an upsampling module achieved by speech super-resolution (SSR). The PRD module can destroy the adversarial perturbations by downsampling, which also introduces randomness and non-differentiability, leading to its resistance to adaptive attacks. PRD does not use any adversarial example as training data, which makes it an attack-agnostic defense method with good generalization ability. Furthermore, the upsampling module achieved by a neural network can recover the downsampled speech to its original quality. In summary, given the experimental results and analysis on the benchmark public datasets, by fusing the downsampling and upsampling modules, SampleShield achieves excellent performance. On adversarial defense, SampleShield obtains an error rate about 5.2% which is quite close to the idea lower bound value 3.2%. On speech quality, SampleShield gets a 5 dB signal-to-noise ratio improvement with respect to the best existing method.
更多查看译文
关键词
Automatic speaker verification,Adversarial defense,Adaptive attacks,Speech super-resolution,Pairwise random downsampling
AI 理解论文
溯源树
样例
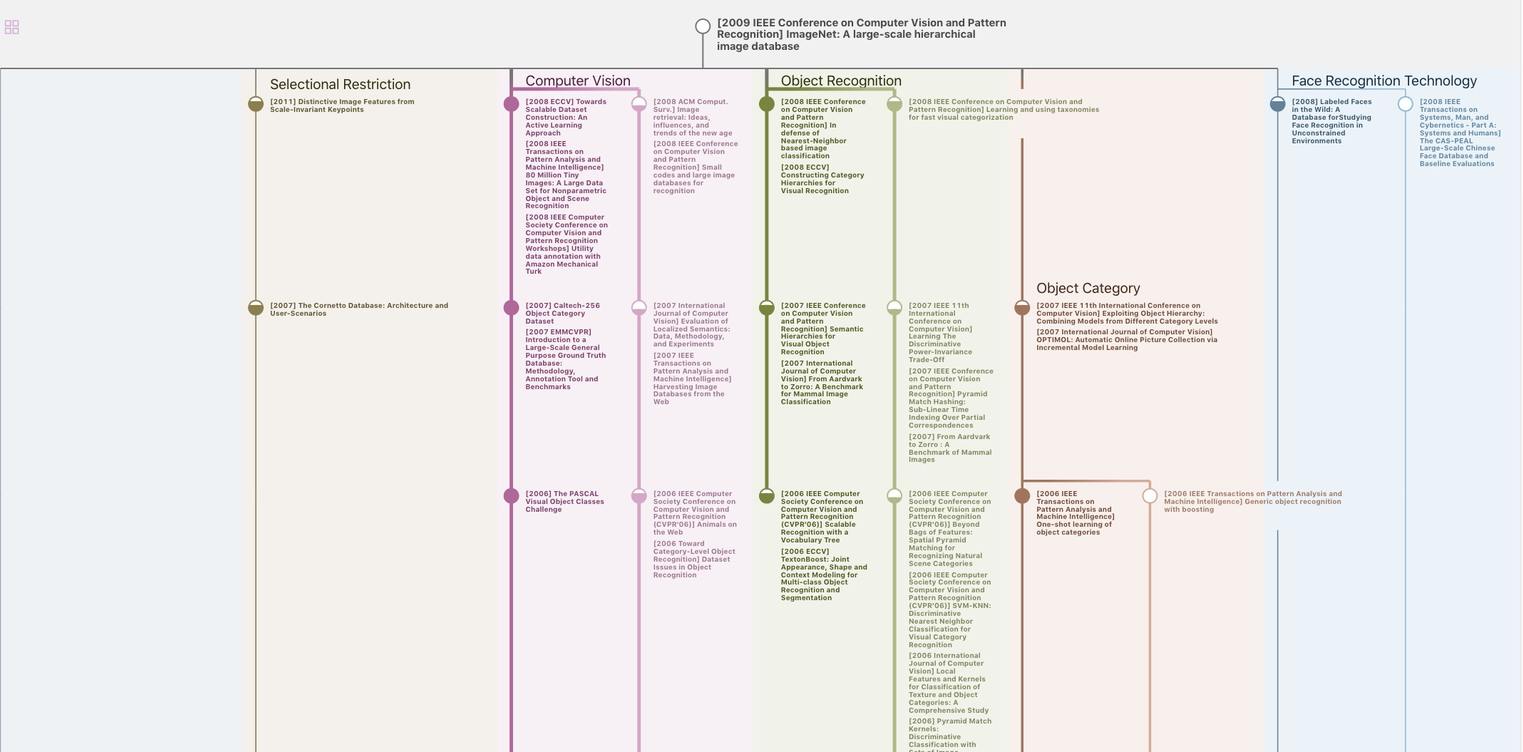
生成溯源树,研究论文发展脉络
Chat Paper
正在生成论文摘要