Robust Image Classification with Noisy Labels by Negative Learning and Feature Space Renormalization
IEEE Transactions on Multimedia(2024)
摘要
Correctly labeled data significantly impacts the success of deep learning for image classification and other computer vision tasks. However, the accuracy of labels annotated by humans often decreases as the amount of data increases, and a rise in the noisy label rate deteriorates a neural network's performance. Thus, deep learning with noisy labels has attracted extensive attention. In this paper, we propose a novel multi-network method that achieves robust performances in image classification with noisy labels. In this method, two models are trained at each epoch using supervised learning and semi-supervised learning. As the selection of the labeled data has a great impact on the semi-supervised learning performance, we improve the sample selection method to obtain a better division of the dataset. Specifically, we divide the semi-supervised learning dataset using a combination of the per-sample losses and model memory. To enhance the semi-supervised learning performance, we propose a novel method to train the unlabeled data by combining negative learning and feature space renormalization. Finally, we verify the performance of our method for learning with noisy labels on four benchmark datasets, which include Cifar10, Cifar100 and Clothing1M. The experimental results show the effectiveness and robustness of our method.
更多查看译文
关键词
feature space renormalization,negative learning,image classification with noisy labels,multi-network architecture
AI 理解论文
溯源树
样例
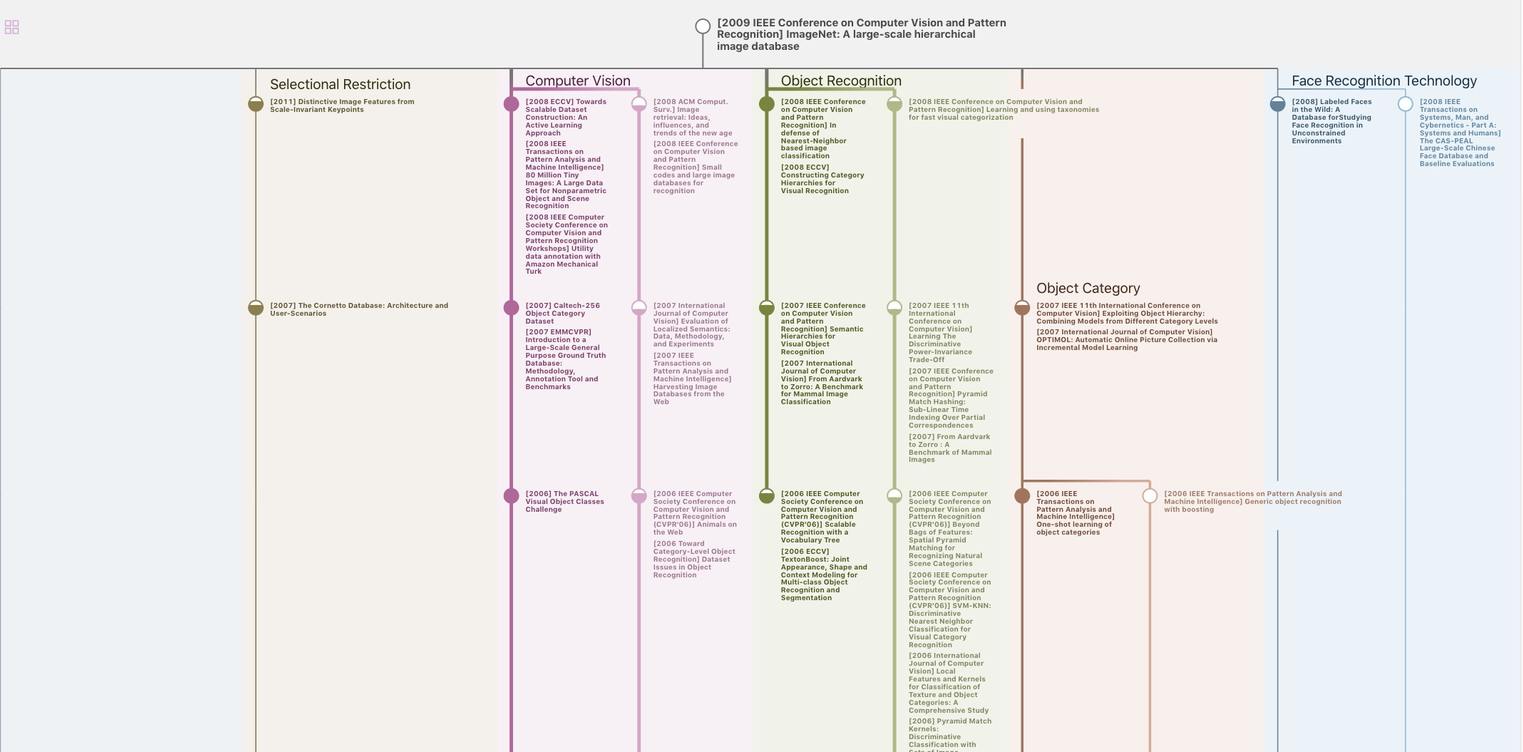
生成溯源树,研究论文发展脉络
Chat Paper
正在生成论文摘要