P2D: Plug and Play Discriminator for accelerating GAN frameworks.
IEEE/CVF Winter Conference on Applications of Computer Vision(2024)
摘要
Most image classification tasks benefit from using pre-trained feature stacks. In contrast, the discriminator for adversarial losses is trained at the same time as the model because using a pretrained feature stack yields a very poor model. Recent work has shown that an implicit regularization scheme allows using pretrained feature stacks to construct a discriminator, which improves both speed of training and quality of results. However, we observe that changes in hyperparameters can result in substantial changes in generator behavior.We show that using a modified version of the R1 regularization scheme that regularizes in the feature space instead of the image space results in a plug-and-play discriminator– P2D. Our scheme results in a method that is highly stable across changes in architecture and framework; that significantly speeds up training; and that produces models that reliably beat SOTA in quality. The huge reduction in training resources required means that P2D could make training powerful generative models over specific datasets accessible to most researchers.
更多查看译文
关键词
Algorithms,Generative models for image,video,3D,etc.,Algorithms,Computational photography,image and video synthesis
AI 理解论文
溯源树
样例
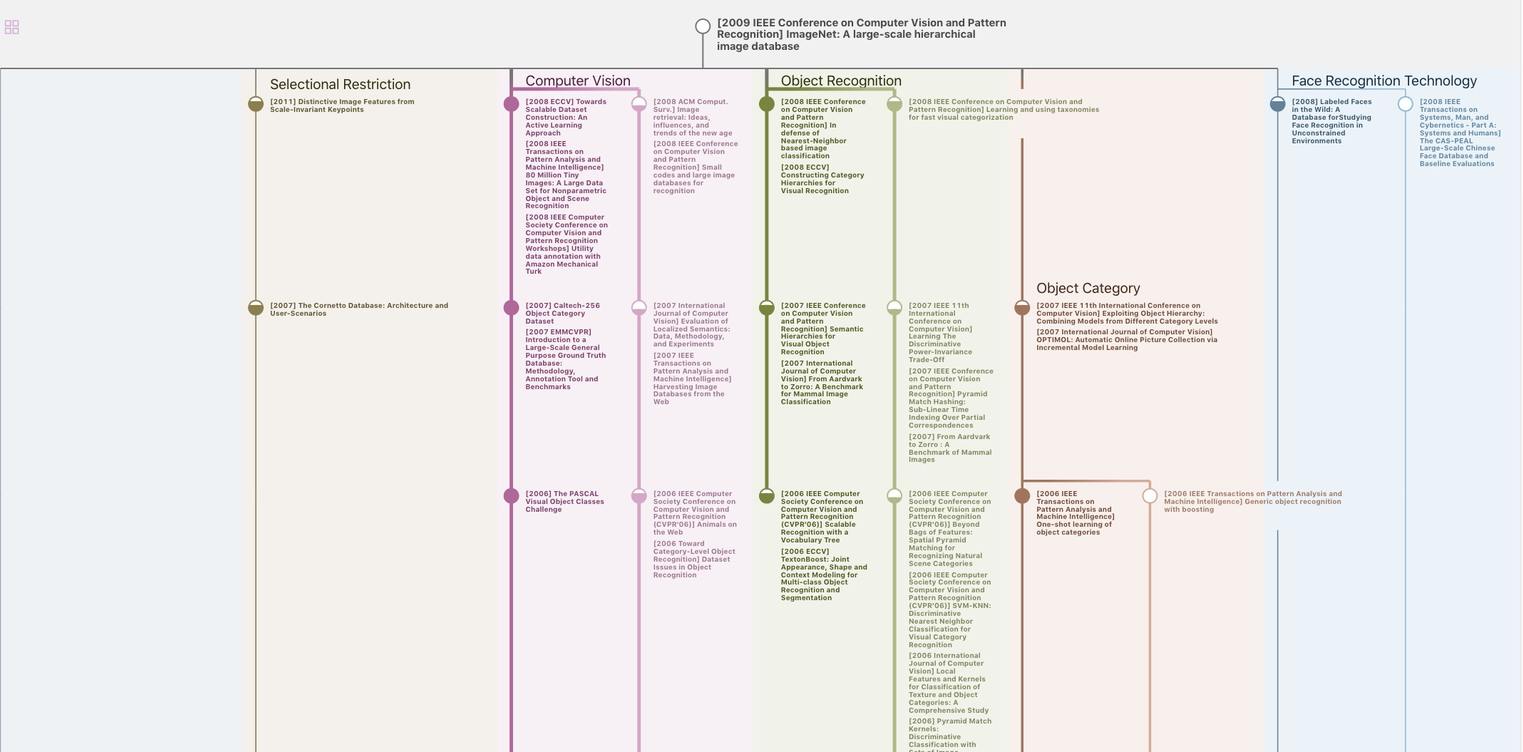
生成溯源树,研究论文发展脉络
Chat Paper
正在生成论文摘要