InternLM-XComposer2-4KHD: A Pioneering Large Vision-Language Model Handling Resolutions from 336 Pixels to 4K HD
CoRR(2024)
摘要
The Large Vision-Language Model (LVLM) field has seen significant
advancements, yet its progression has been hindered by challenges in
comprehending fine-grained visual content due to limited resolution. Recent
efforts have aimed to enhance the high-resolution understanding capabilities of
LVLMs, yet they remain capped at approximately 1500 x 1500 pixels and
constrained to a relatively narrow resolution range. This paper represents
InternLM-XComposer2-4KHD, a groundbreaking exploration into elevating LVLM
resolution capabilities up to 4K HD (3840 x 1600) and beyond. Concurrently,
considering the ultra-high resolution may not be necessary in all scenarios, it
supports a wide range of diverse resolutions from 336 pixels to 4K standard,
significantly broadening its scope of applicability. Specifically, this
research advances the patch division paradigm by introducing a novel extension:
dynamic resolution with automatic patch configuration. It maintains the
training image aspect ratios while automatically varying patch counts and
configuring layouts based on a pre-trained Vision Transformer (ViT) (336 x
336), leading to dynamic training resolution from 336 pixels to 4K standard.
Our research demonstrates that scaling training resolution up to 4K HD leads to
consistent performance enhancements without hitting the ceiling of potential
improvements. InternLM-XComposer2-4KHD shows superb capability that matches or
even surpasses GPT-4V and Gemini Pro in 10 of the 16 benchmarks. The
InternLM-XComposer2-4KHD model series with 7B parameters are publicly available
at https://github.com/InternLM/InternLM-XComposer.
更多查看译文
AI 理解论文
溯源树
样例
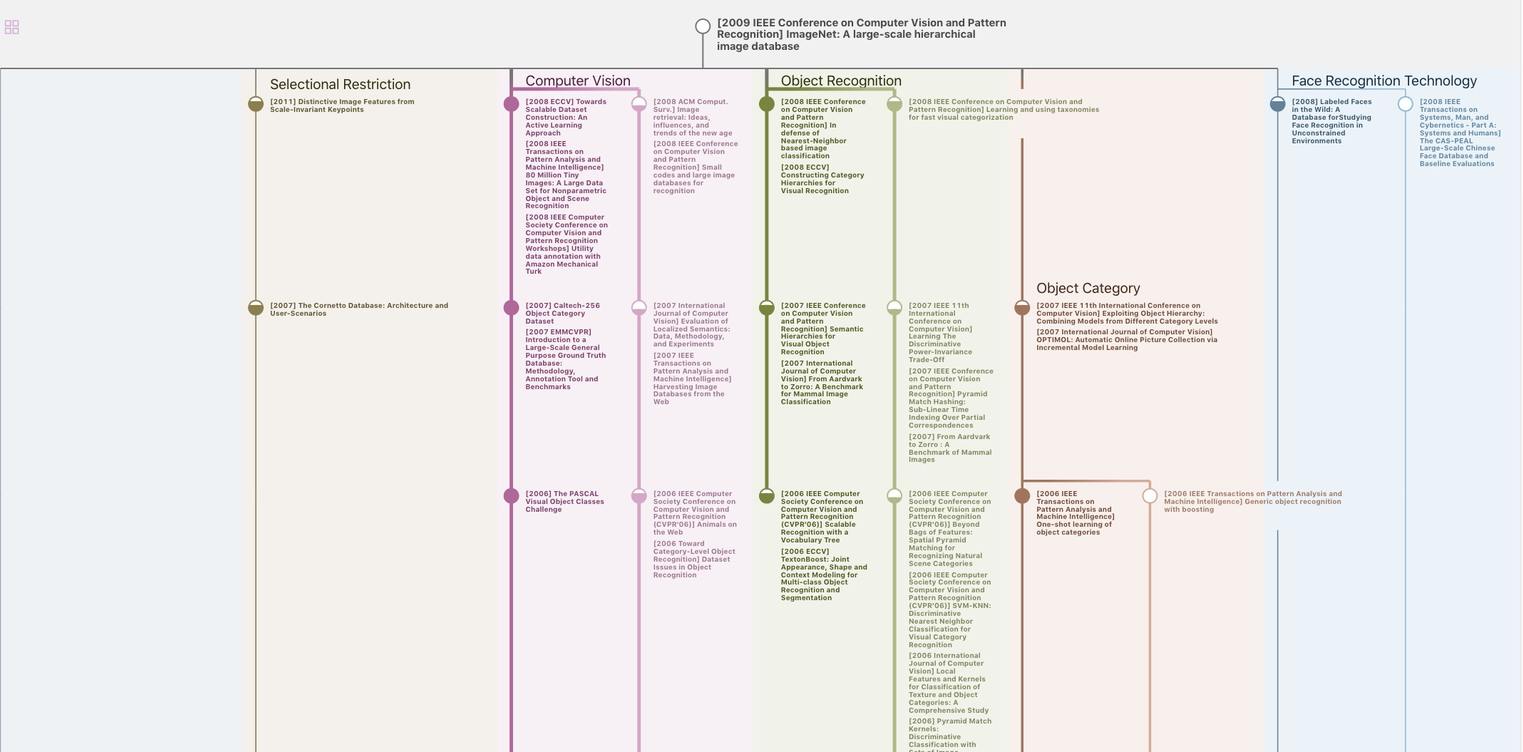
生成溯源树,研究论文发展脉络
Chat Paper
正在生成论文摘要