AdaNAS: Adaptively Postprocessing With Self-Supervised Neural Architecture Search for Ensemble Rainfall Forecasts
IEEE TRANSACTIONS ON GEOSCIENCE AND REMOTE SENSING(2024)
摘要
Previous postprocessing studies on rainfall forecasts using numerical weather prediction (NWP) mainly focus on statistics-based aspects, while learning-based aspects are rarely investigated. Although some manually designed models are proposed to raise accuracy, they are customized networks, which need to be repeatedly tried and verified, at a huge cost in time and labor. Therefore, a self-supervised neural architecture search (NAS) method without significant manual efforts called AdaNAS is proposed in this study to perform rainfall forecast postprocessing and predict rainfall with high accuracy. In addition, we design a rainfall-aware search space to significantly improve forecasts for high-rainfall areas. Furthermore, we propose a rainfall-level regularization function to eliminate the effect of noise data during the training. Validation experiments have been performed under the cases of None, Light, Moderate, Heavy, and Violent on a large-scale precipitation benchmark named TIGGE. Finally, the average mean-absolute error (MAE) and average root-mean-square error (RMSE) of the proposed AdaNAS model are 0.98 and 2.04 mm/day, respectively. Additionally, the proposed AdaNAS model is compared with other NAS methods and previous studies. Compared results reveal the satisfactory performance and superiority of the proposed AdaNAS model in terms of precipitation amount prediction and intensity classification. Concretely, the proposed AdaNAS model outperformed previous best-performing manual methods with MAE and RMSE improving by 80.5% and 80.3%, respectively.
更多查看译文
关键词
Automated machine learning,neural architecture search (NAS),precipitation prediction,rainfall forecasts,rainfall-level regularization,self-supervised learning
AI 理解论文
溯源树
样例
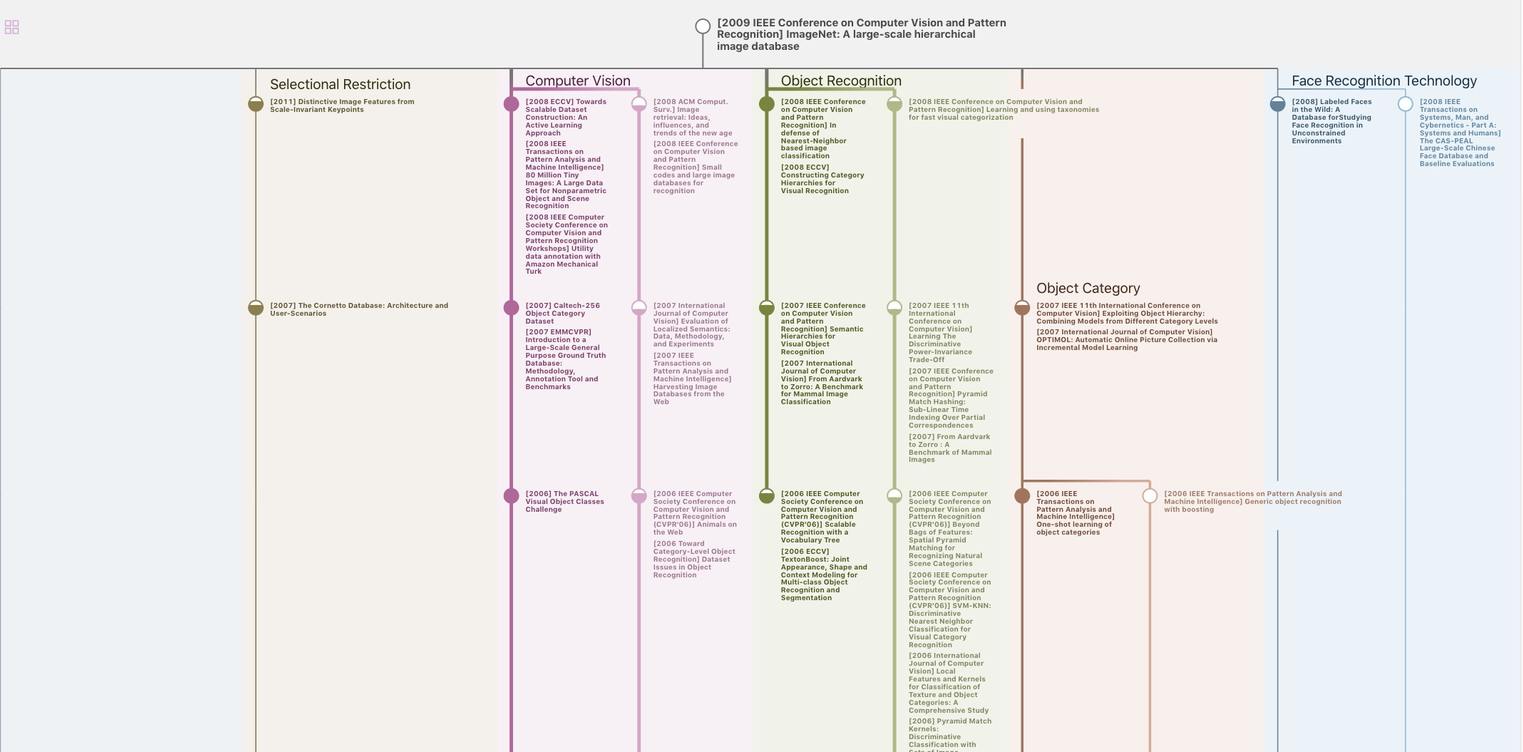
生成溯源树,研究论文发展脉络
Chat Paper
正在生成论文摘要