Hierarchical Dependencies in Classroom Settings Influence Algorithmic Bias Metrics
FOURTEENTH INTERNATIONAL CONFERENCE ON LEARNING ANALYTICS & KNOWLEDGE, LAK 2024(2024)
摘要
Measuring algorithmic bias in machine learning has historically focused on statistical inequalities pertaining to specific groups. However, the most common metrics (i.e., those focused on individualor group-conditioned error rates) are not currently well-suited to educational settings because they assume that each individual observation is independent from the others. This is not statistically appropriate when studying certain common educational outcomes, because such metrics cannot account for the relationship between students in classrooms or multiple observations per student across an academic year. In this paper, we present novel adaptations of algorithmic bias measurements for regression for both independent and nested data structures. Using hierarchical linear models, we rigorously measure algorithmic bias in a machine learning model of the relationship between student engagement in an intelligent tutoring system and year-end standardized test scores. We conclude that classroom-level influences had a small but significant effect on models. Examining significance with hierarchical linear models helps determine which inequalities in educational settings might be explained by small sample sizes rather than systematic differences.
更多查看译文
关键词
Interactive learning environments,Algorithmic bias,Intelligent tutoring systems,Predictive analytics
AI 理解论文
溯源树
样例
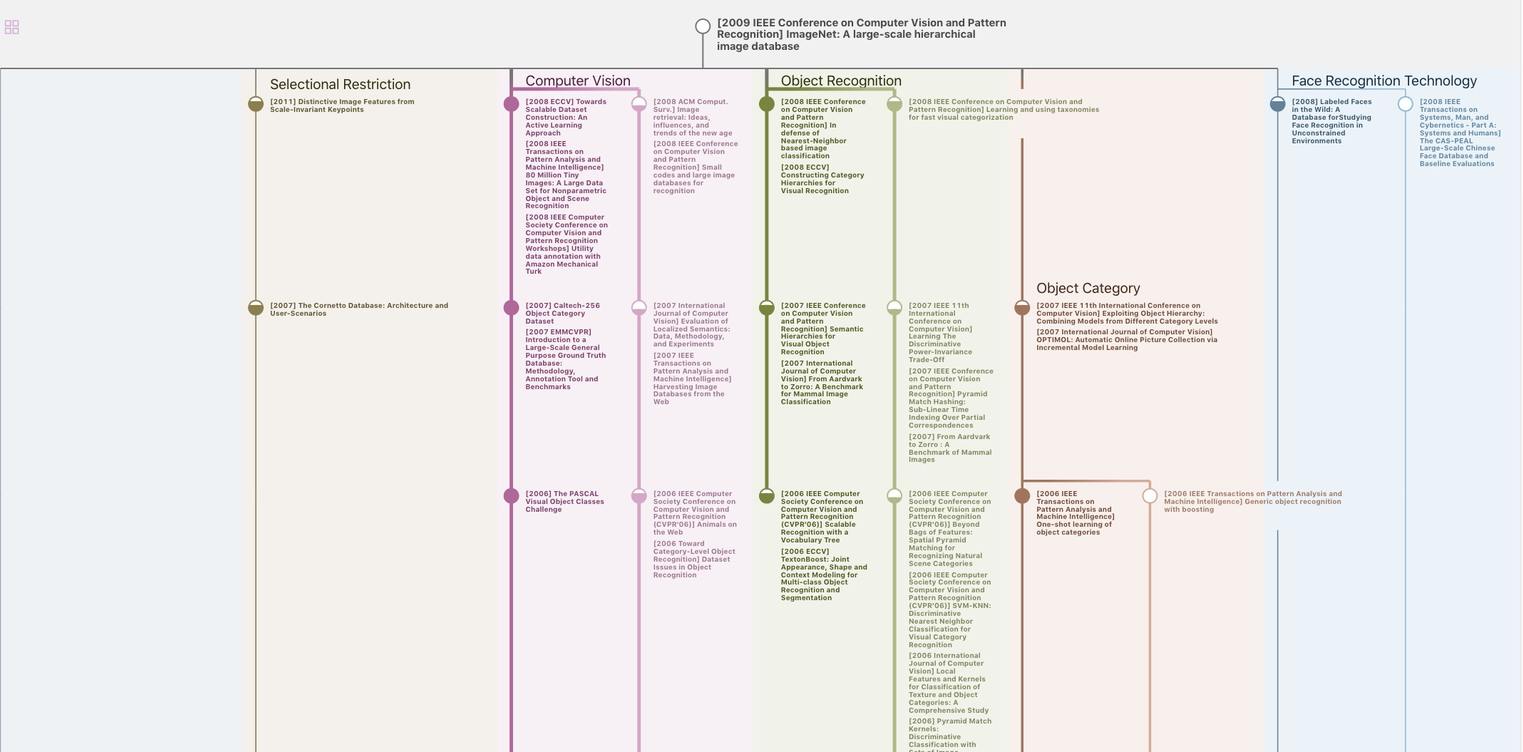
生成溯源树,研究论文发展脉络
Chat Paper
正在生成论文摘要