Unified Visual Preference Learning for User Intent Understanding
PROCEEDINGS OF THE 17TH ACM INTERNATIONAL CONFERENCE ON WEB SEARCH AND DATA MINING, WSDM 2024(2024)
摘要
In the world of E-Commerce, the core task is to understand the personalized preference from various kinds of heterogeneous information, such as textual reviews, item images and historical behaviors. In current systems, these heterogeneous information are mainly exploited to generate better item or user representations. For example, in scenario of visual search, the importance of modeling query image has been widely acknowledged. But, these existing solutions focus on improving the representation quality of the query image, overlooking the personalized visual preference of the user. Note that the visual features affect the user's decision significantly, e.g., a user could be more likely to click the items with her preferred design. Hence, it is fruitful to exploit the visual preference to deliver better capacity for personalization. To this end, we propose a simple yet effective target-aware visual preference learning framework (named Tavern) for both item recommendation and search. The proposed Tavern works as an individual and generic model that can be smoothly plugged into different downstream systems. Specifically, for visual preference learning, we utilize the image of the target item to derive the visual preference signals for each historical clicked item. This procedure is modeled as a form of representation disentanglement, where the visual preference signals are extracted by taking off the noisy information irrelevant to visual preference from the shared visual information between the target and historical items. During this process, a novel selective orthogonality disentanglement is proposed to avoid the significant information loss. Then, a GRU network is utilized to aggregate these signals to form the final visual preference representation. Extensive experiments over three large-scale real-world datasets covering visual search, product search and recommendation well demonstrate the superiority of our proposed Tavern against existing technical alternatives. Further ablation study also confirms the validity of each design choice
更多查看译文
关键词
Visual Preference Modeling,Disentangled Representation Learning,Search and Recommendation
AI 理解论文
溯源树
样例
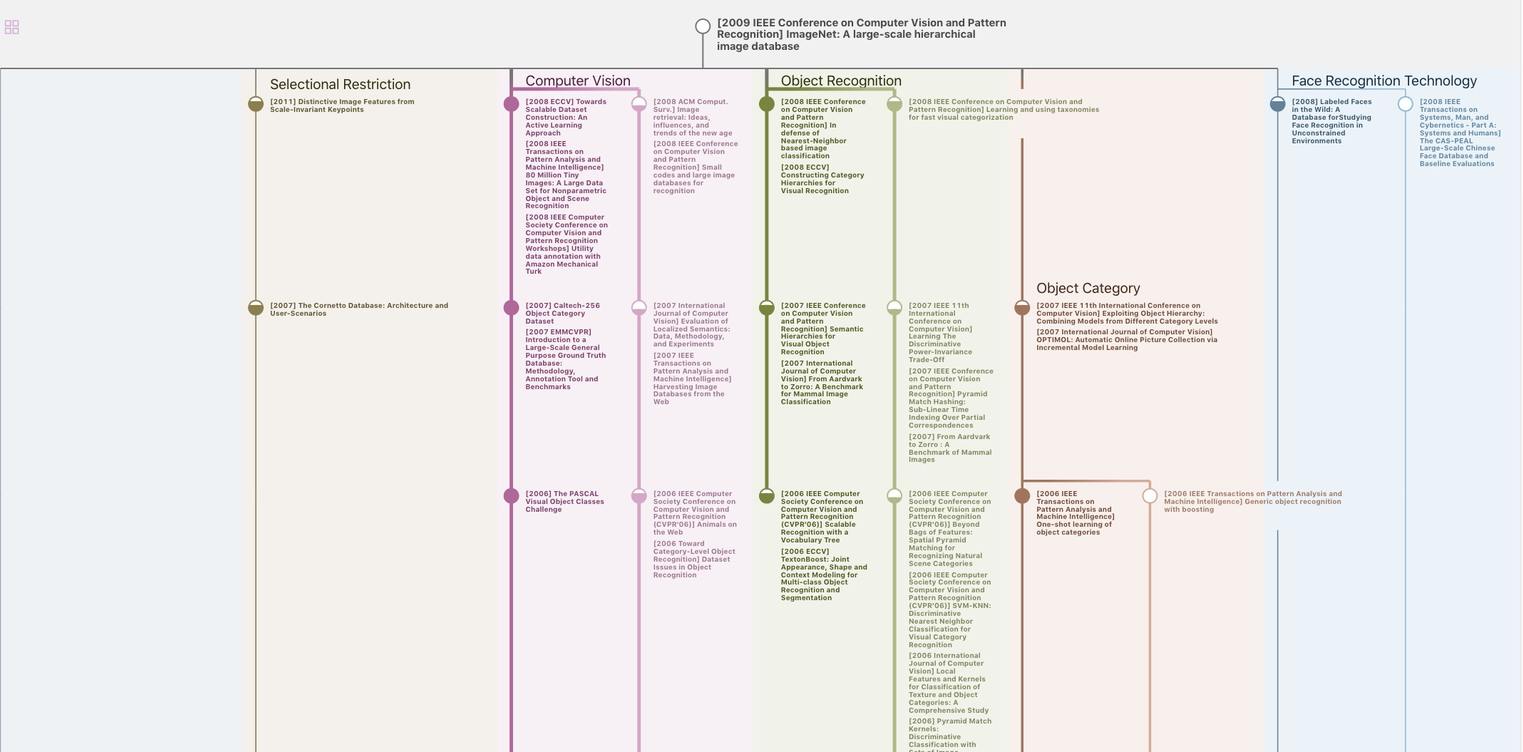
生成溯源树,研究论文发展脉络
Chat Paper
正在生成论文摘要