Efficient, Direct, and Restricted Black-Box Graph Evasion Attacks to Any-Layer Graph Neural Networks via Influence Function
PROCEEDINGS OF THE 17TH ACM INTERNATIONAL CONFERENCE ON WEB SEARCH AND DATA MINING, WSDM 2024(2024)
摘要
Graph neural network (GNN), the mainstream method to learn on graph data, is vulnerable to graph evasion attacks, where an attacker slightly perturbing the graph structure can fool trainedGNNmodels. Existing work has at least one of the following drawbacks: 1) limited to directly attack two-layer GNNs; 2) inefficient; and 3) impractical, as they need to know full or part of GNN model parameters. We address the above drawbacks and propose an influence-based efficient, direct, and restricted black-box evasion attack to any-layer GNNs. Specifically, we first introduce two influence functions, i.e., feature-label influence and label influence, that are defined on GNNs and label propagation (LP), respectively. Then we observe that GNNs and LP are strongly connected in terms of our defined influences. Based on this, we can then reformulate the evasion attack to GNNs as calculating label influence on LP, which is inherently applicable to any-layer GNNs, while no need to know information about the internal GNN model. Finally, we propose an efficient algorithm to calculate label influence. Experimental results on various graph datasets show that, compared to state-of-the-art white-box attacks, our attack can achieve comparable attack performance, but has a 5-50x speedup when attacking two-layer GNNs. Moreover, our attack is effective to attack multi-layer GNNs1.
更多查看译文
关键词
graph neural network,label propagation,attack,influence function
AI 理解论文
溯源树
样例
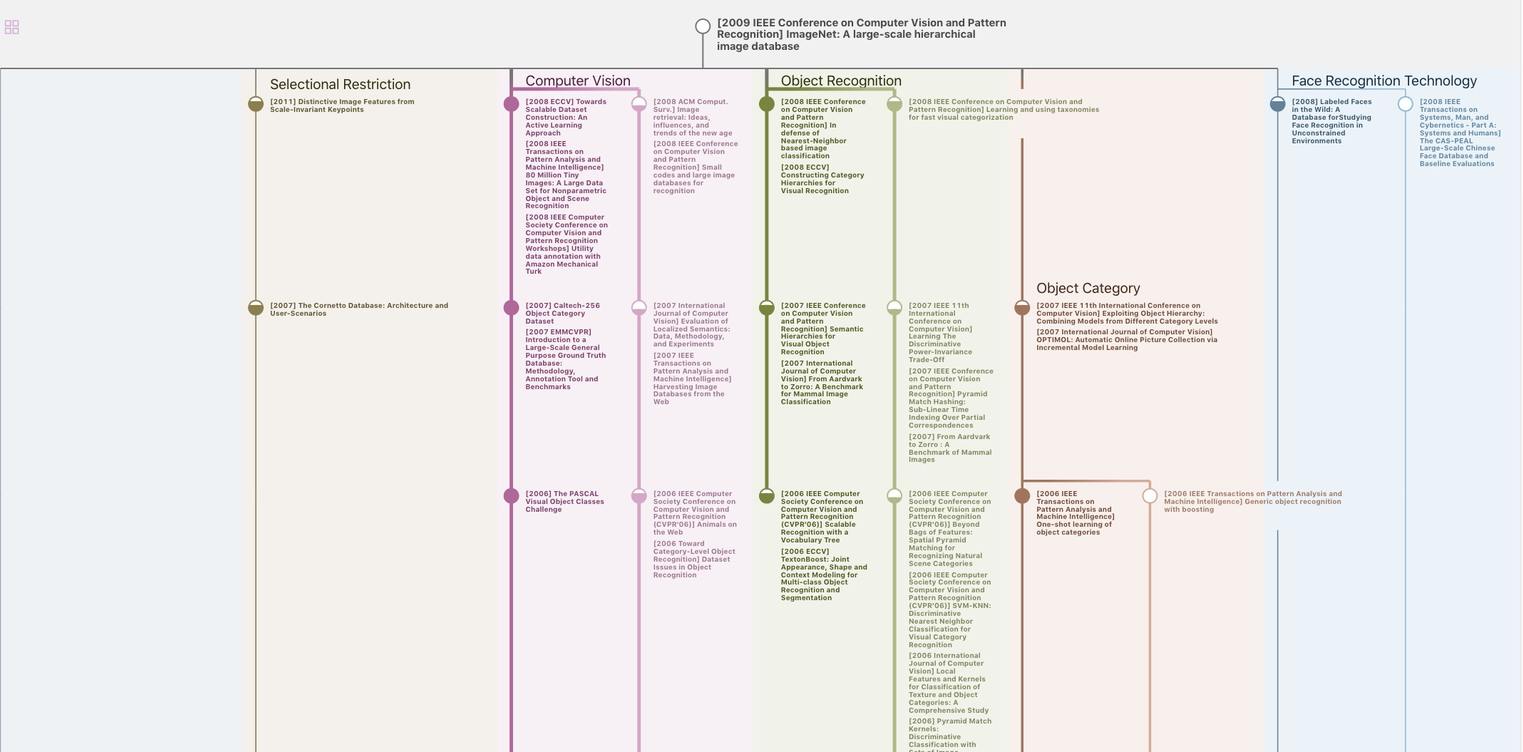
生成溯源树,研究论文发展脉络
Chat Paper
正在生成论文摘要