User Behavior Enriched Temporal Knowledge Graphs for Sequential Recommendation
PROCEEDINGS OF THE 17TH ACM INTERNATIONAL CONFERENCE ON WEB SEARCH AND DATA MINING, WSDM 2024(2024)
摘要
Knowledge Graphs (KGs) enhance recommendations by providing external connectivity between items. However, there is limited research on distilling relevant knowledge in sequential recommendation, where item connections can change over time. To address this, we introduce the Temporal Knowledge Graph (TKG), which incorporates such dynamic features of user behaviors into the original KG while emphasizing sequential relationships. The TKG captures both patterns of entity dynamics (nodes) and structural dynamics (edges). Considering real-world applications with large-scale and rapidly evolving user behavior patterns, we propose an efficient two-phase framework called TKG-SRec, which strengthens Sequential Rec-ommendation with Temporal KGs. In the first phase, we learn dynamic entity embeddings using our novel Knowledge Evolution Network (KEN) that brings together pretrained static knowledge with evolving temporal knowledge. In the second stage, downstream sequential recommender models utilize these time-specific dynamic entity embeddings with compatible neural backbones like GRUs, Transformers, and MLPs. From our extensive experiments over four datasets, TKG-SRec outperforms the current state-of-the-art by a statistically significant 5% on average. Detailed analysis validates that such filtered temporal knowledge better adapts entity embedding for sequential recommendation. In summary, TKG-SRec provides an effective and efficient approach.
更多查看译文
关键词
Sequential Recommendation,Knowledge Graph
AI 理解论文
溯源树
样例
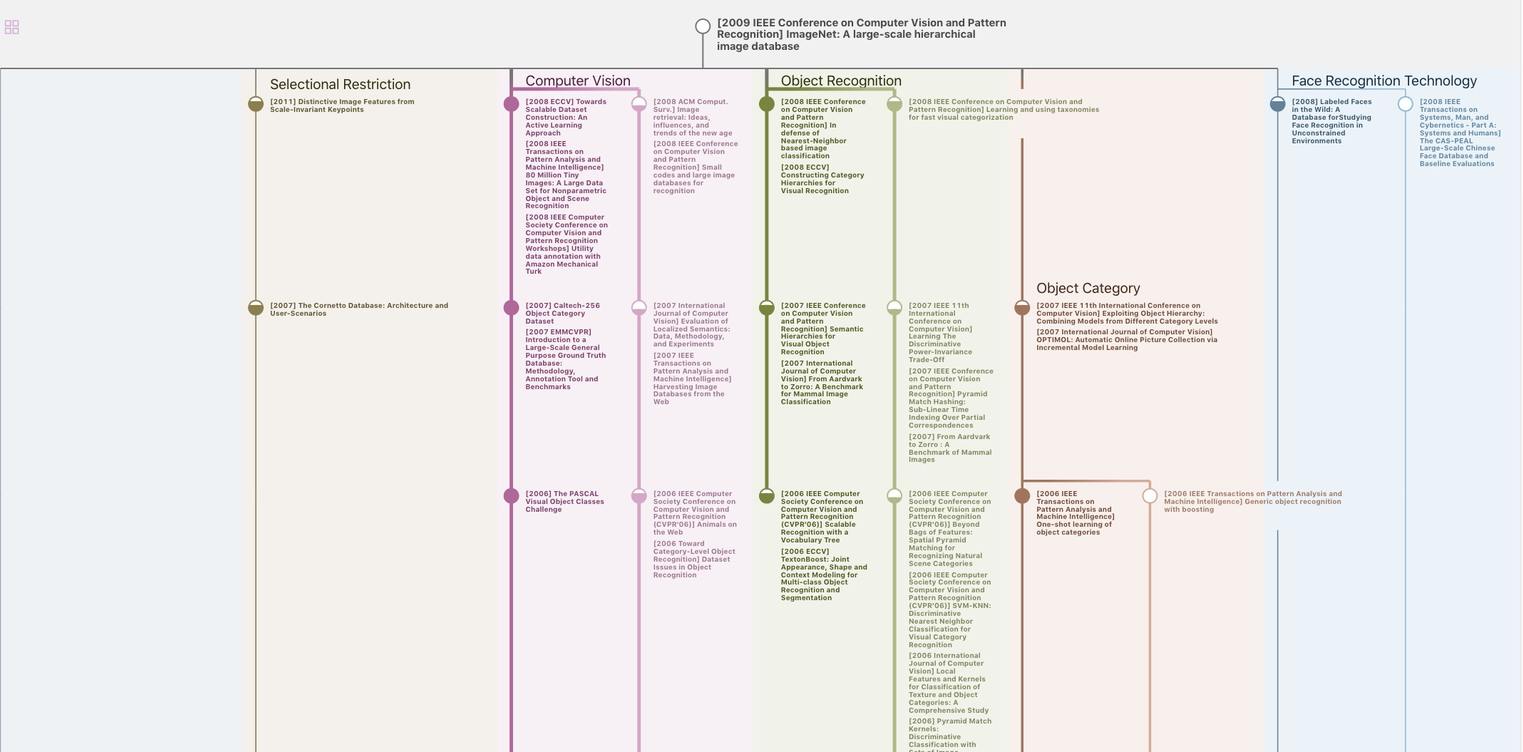
生成溯源树,研究论文发展脉络
Chat Paper
正在生成论文摘要