Examining Performance Loss Mechanisms in Lithium-Ion Batteries with the High-Voltage Mn-Rich Spinel Positive Electrodes
JOURNAL OF THE ELECTROCHEMICAL SOCIETY(2024)
摘要
The high-voltage spinel, with the nominal composition of LiNi0.5-xMn1.5+xO4 (LNMO), could be a sustainable alternative to the layered-oxide positive electrodes used in lithium-ion batteries. However, commercial acceptance has been limited as LNMO cells display rapid performance loss during cycling. To examine reasons for this loss we prepared cells with LNMO-based positive and either graphite or lithium titanate (LTO) based negative electrodes. Our initial cells displayed high impedance and rapid impedance rise during cycling. Adding single walled carbon nanotubes in the positive lowered initial cell impedance but impedance increased during cycling because of coating delamination from the Al foil. Using a primed Al current collector solved the delamination problem: cells with this current collector showed only a small impedance rise. Regarding capacity, cells with the LTO negative showed higher initial capacities and smaller fade. Our data indicate that cell capacity retention is determined by reduction reactions at the negative electrode that lower capacity and oxidation reactions at the positive electrode that increase capacity: both types of reactions deplete the cell electrolyte during extended cycling. All things considered, species generated at high voltages, either in the electrolyte or at the LNMO electrode, drive cell capacity fade. (c) 2024 The Author(s). Published on behalf of The Electrochemical Society by IOP Publishing Limited. This is an open accessarticle distributed under the terms of the Creative Commons Attribution 4.0 License (CC BY, http://creativecommons.org/licenses/by/4.0/), which permits unrestricted reuse of the work in any medium, provided the original work is properly cited.
更多查看译文
关键词
5V spinel,graphite,Li4Ti5O12,single walled carbon nanotubes,primed Aluminum current collector,electrochemical cycling,material characterization
AI 理解论文
溯源树
样例
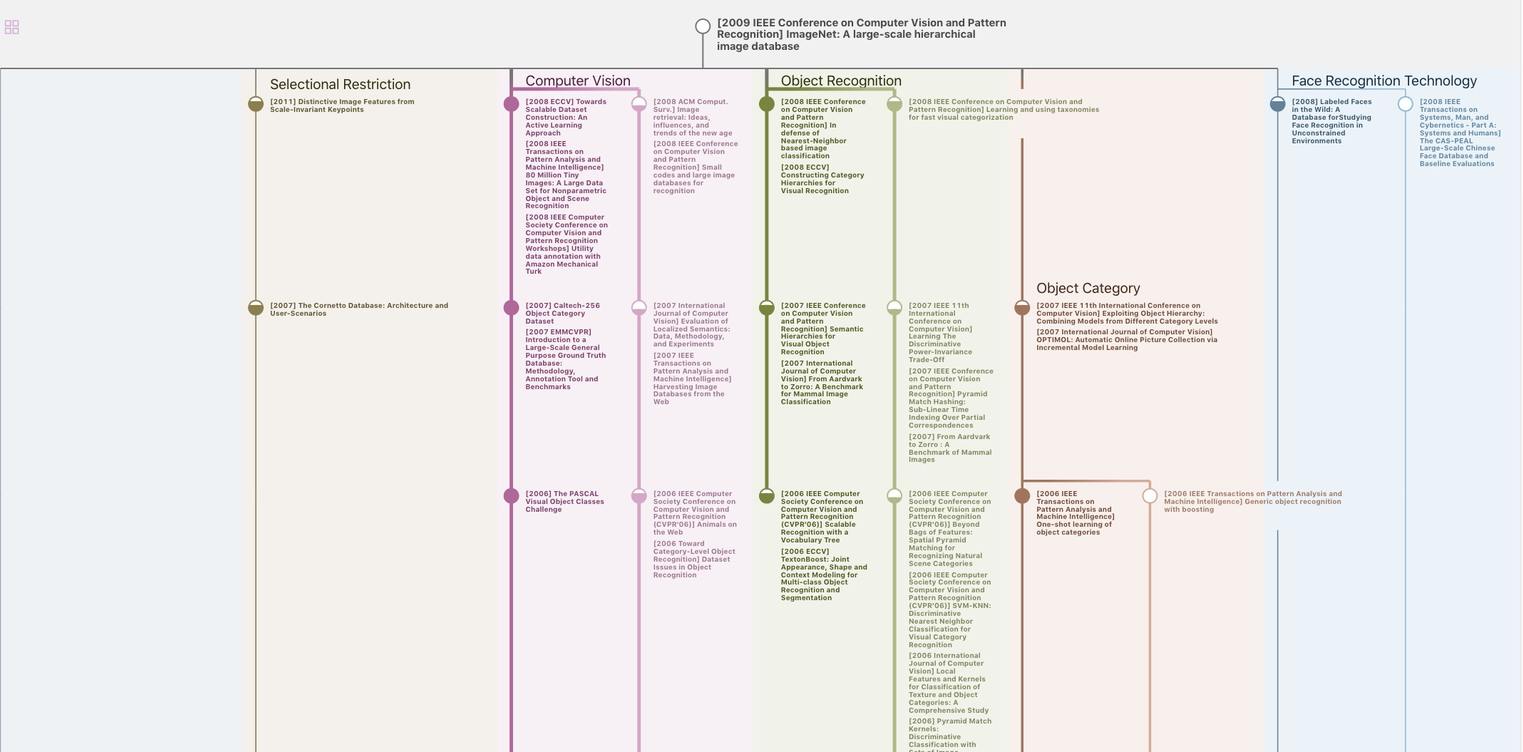
生成溯源树,研究论文发展脉络
Chat Paper
正在生成论文摘要