AI-augmented Automation for Real Driving Prediction: an Industrial Use Case
arxiv(2024)
摘要
The risen complexity of automotive systems requires new development
strategies and methods to master the upcoming challenges. Traditional methods
need thus to be changed by an increased level of automation, and a faster
continuous improvement cycle. In this context, current vehicle performance
tests represent a very time-consuming and expensive task due to the need to
perform the tests in real driving conditions. As a consequence, agile/iterative
processes like DevOps are largely hindered by the necessity of triggering
frequent tests. This paper reports on a practical experience of developing an
AI-augmented solution based on Machine Learning and Model-based Engineering to
support continuous vehicle development and testing. In particular, historical
data collected in real driving conditions is leveraged to synthesize a
high-fidelity driving simulator and hence enable performance tests in virtual
environments. Based on this practical experience, this paper also proposes a
conceptual framework to support predictions based on real driving behavior.
更多查看译文
AI 理解论文
溯源树
样例
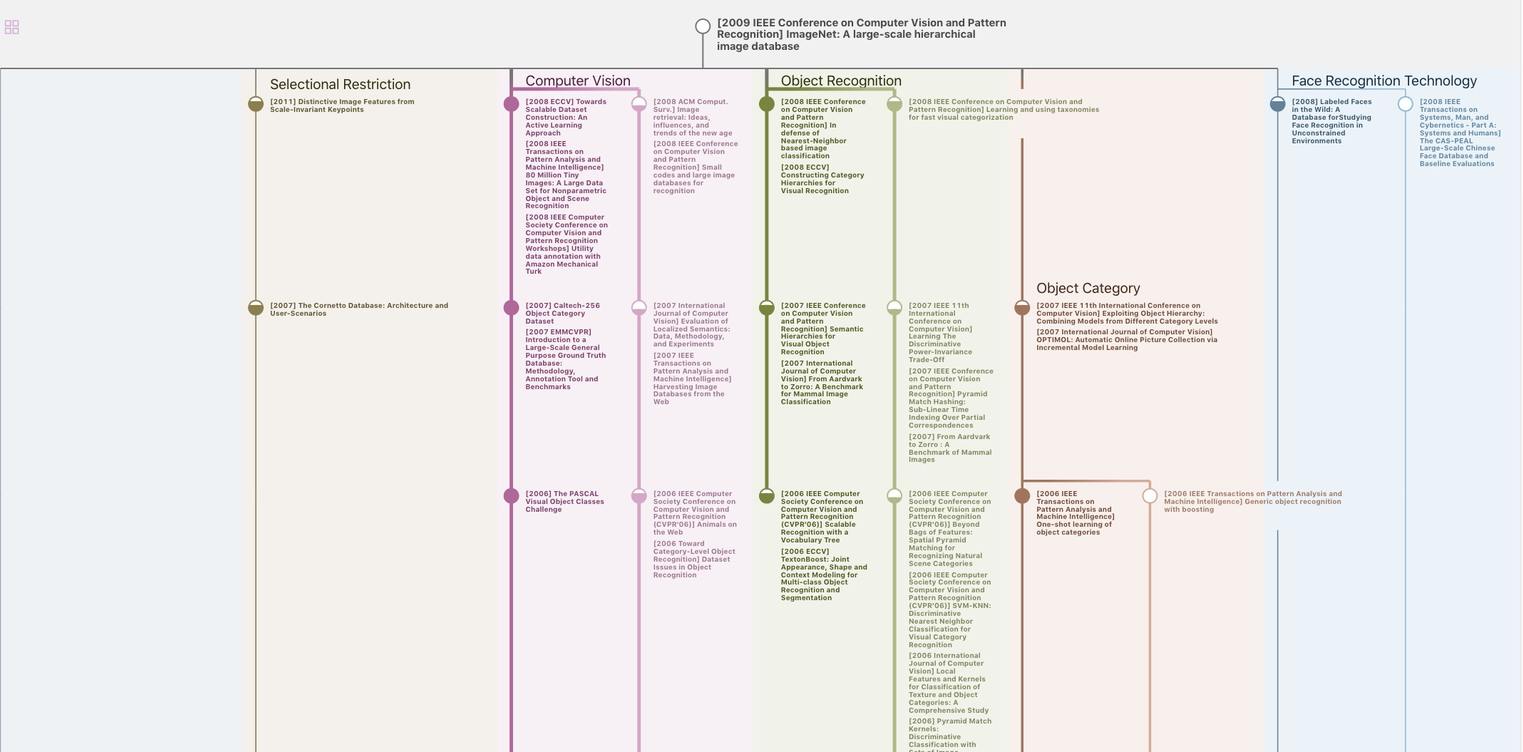
生成溯源树,研究论文发展脉络
Chat Paper
正在生成论文摘要