Reconfigurable Processing-in-Memory Architecture for Data Intensive Applications.
International Conference on VLSI Design(2024)
摘要
Emerging applications reliant on deep neural networks (DNNs) and convolutional neural networks (CNNs) demand substantial data for computation and analysis. Deploying DNNs and CNNs often leads to resource constraints, data movement overheads between memory and compute units. Architectural paradigms like Processing-in-Memory (PIM) have emerged to mitigate these challenges. However, existing PIM architectures necessitate trade-offs involving power, performance, area, energy efficiency, and programmability. Our proposed solution focuses on achieving higher energy efficiency while preserving programmability and flexibility. We introduce a novel multi-core reconfigurable architecture with fine-grained integration within DRAM sub-arrays, resulting in superior performance and energy-efficiency compared to conventional PIM architectures. Each core in our design comprises multiple processing elements (PEs), standalone processors equipped with programmable functional units constructed using high-speed reconfigurable multi-functional look-up-tables (M-LUTs). These M-LUTs enable multiple functional outputs, such as convolution, pooling, and activation functions, in a time-multiplexed manner, eliminating the need for different LUTs for each function. Special function LUTs provide simultaneous outputs, enabling ultra-low latency parallel processing for tasks like multiplication and accumulation, along with functions like activation, pooling, and batch-normalization required for CNN acceleration. This comprehensive approach enhances efficiency and performance, rendering our reconfigurable architecture suitable for demanding Big Data and AI acceleration applications
更多查看译文
AI 理解论文
溯源树
样例
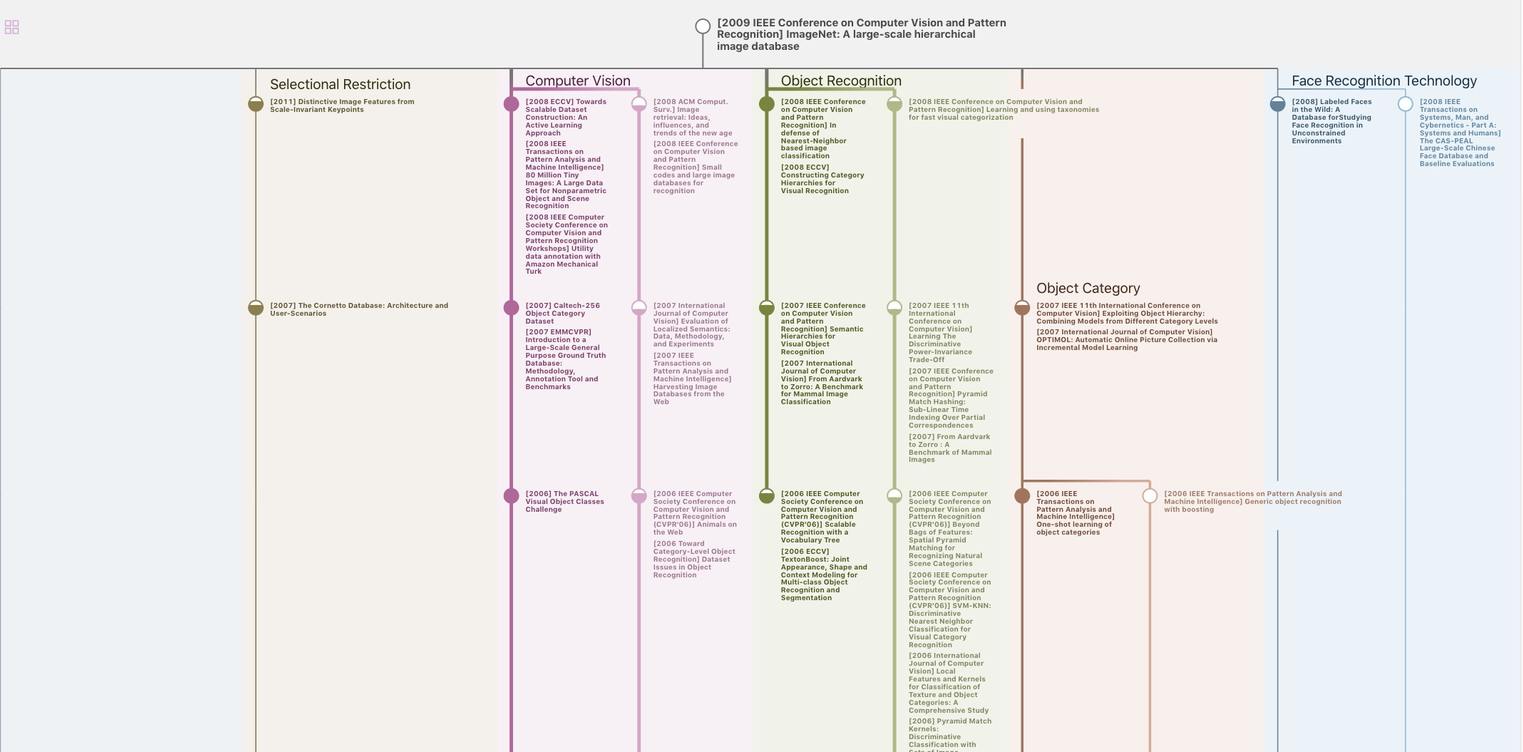
生成溯源树,研究论文发展脉络
Chat Paper
正在生成论文摘要