Extracting Structured Information from the Textual Description of Geometry Word Problems
PROCEEDINGS OF 2023 7TH INTERNATIONAL CONFERENCE ON NATURAL LANGUAGE PROCESSING AND INFORMATION RETRIEVAL, NLPIR 2023(2023)
摘要
AI (Artificial Intelligence) is playing its role in every field as automation is the way forward. Solving geometry mathematics word problems (MWPs) automatically can help in smart tutoring and has a plethora of applications in many fields. To solve a geometry MWPs, multi-modal methods are needed to parse the question text using Natural Language Processing (NLP) and diagram using Image Processing (IP) techniques and combine the information from both. The information is then to be structured and appropriate axioms and theorems need to be applied to transform the question into intermediate equations which could be solved by equation solvers. In this entire pipeline, text parsing of the question content is a crucial component which is precisely the main focus of this paper. There have been attempts in the literature and techniques to solve geometry MWPs have been proposed, however they rely on input predicates and do not generate them automatically. In cases where they are generated, regular expressions are used which lack generalisation and scalability. This paper models the text parsing problem as a Relation Extraction and Natural Language Generation problem where from the question text structured information is generated in the form of predicates. We basically generate appropriate geometric tags by using a Named Entity Recognition (NER) annotator and then utilize these tags to generate predicates that represent the question in a machine readable formal language. To test the proposed approach, a custom dataset is created containing about 500 questions from standard elementary school-level Indian text books. It has been demonstrated through experiments on the custom dataset that the suggested method is capable of extracting geometric relations. To evaluate the generated predicates, we create ground truth predicates for each of the questions through skilled domain experts. We also design a unique method to evaluate the generated predicates with the METEOR (Metric for Evaluation of Translation with Explicit ORdering) metric. The experimental findings on this dataset indicate precision (P) of 0.70, recall (R) of 0.60, harmonic mean (F-mean) of 0.60, average penalty (p) of 0.12 and final METEOR Score (M) of 0.54. Furthermore, we experiment the proposed technique on another dataset. When tested on Geometry3K data set, a precision of 0.67, recall of 0.64, harmonic mean (F-mean) of 0.64, penalty (p) of 0.27 and final METEOR Score (M) of 0.42 is obtained.
更多查看译文
关键词
Natural Language Processing,Artificial Intelligence,Mathematics (Geometry) word problems,Named Entity Recognition,Predicate Generation
AI 理解论文
溯源树
样例
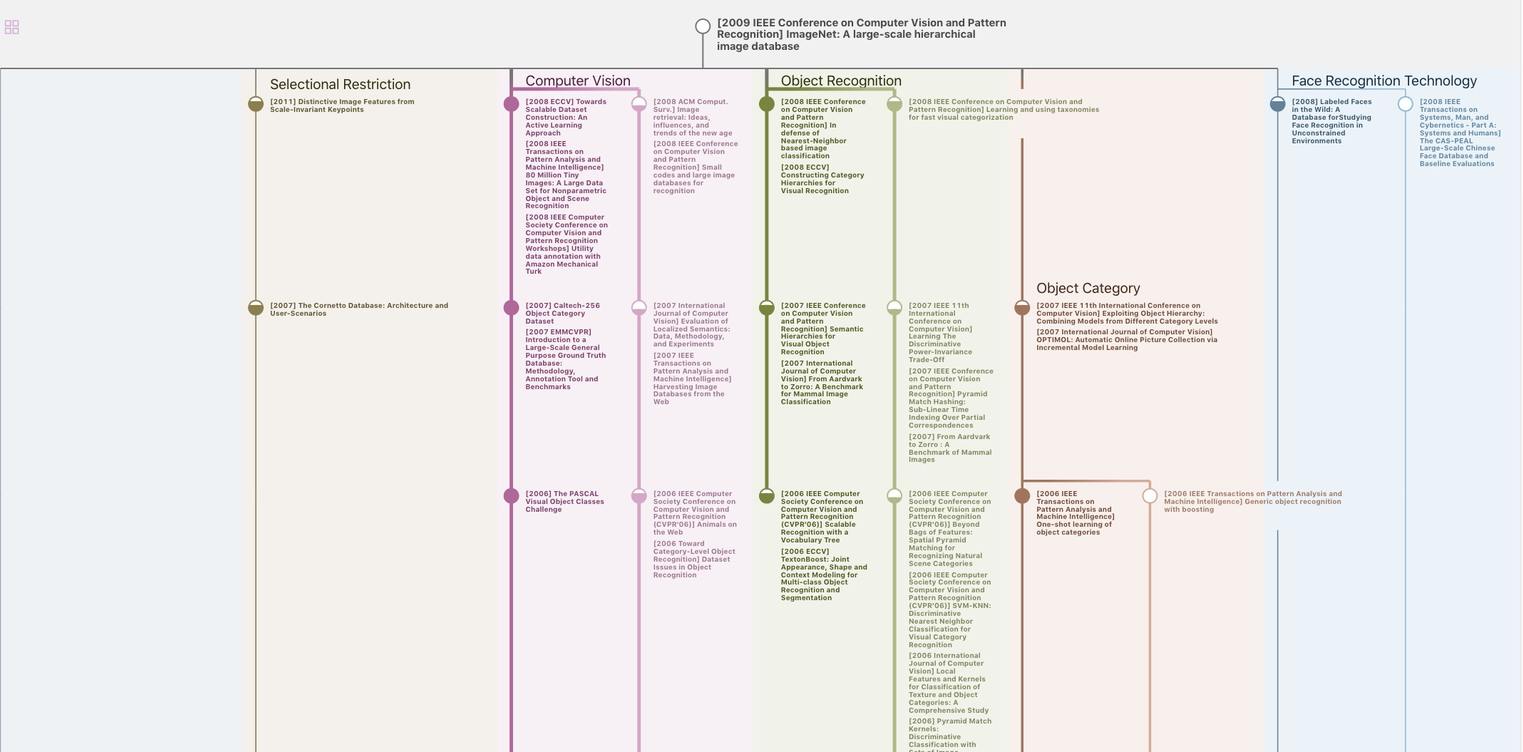
生成溯源树,研究论文发展脉络
Chat Paper
正在生成论文摘要