Quantisation Effects in Adversarial Cyber-Physical Games
DECISION AND GAME THEORY FOR SECURITY, GAMESEC 2023(2023)
摘要
As the complexity in models of cyber-physical systems increases, conventional game-theoretic tools begin to struggle due to the presence of hard-to-evaluate nonconvex game dynamics. Complex adversarial security games often exhibit nonconvex behaviour such as those admitted by a Kuramoto-Sakaguchi system with Lanchester dynamics. We pose this system as a two-player, zero-sum dynamic security game, where players seek to gain a decision advantage over their opponents. By leveraging multi-agent reinforcement learning, we study the impact action space quantisation has on a player's ability to uncover optimal strategies to achieve a decision advantage in a complex decision-making system. A comparison of solutions on continuous and discrete action spaces reveals good agreement across algorithms indicating convergence to some epsilon-Nash equilibrium. Surprisingly, the higher fidelity offered by continuous action spaces also yields computational advantages compared to discrete spaces in the context of the adversarial decision game.
更多查看译文
关键词
Cyber-Physical Systems,Adversarial Games,Multi-Agent Reinforcement Learning,Dynamical Systems
AI 理解论文
溯源树
样例
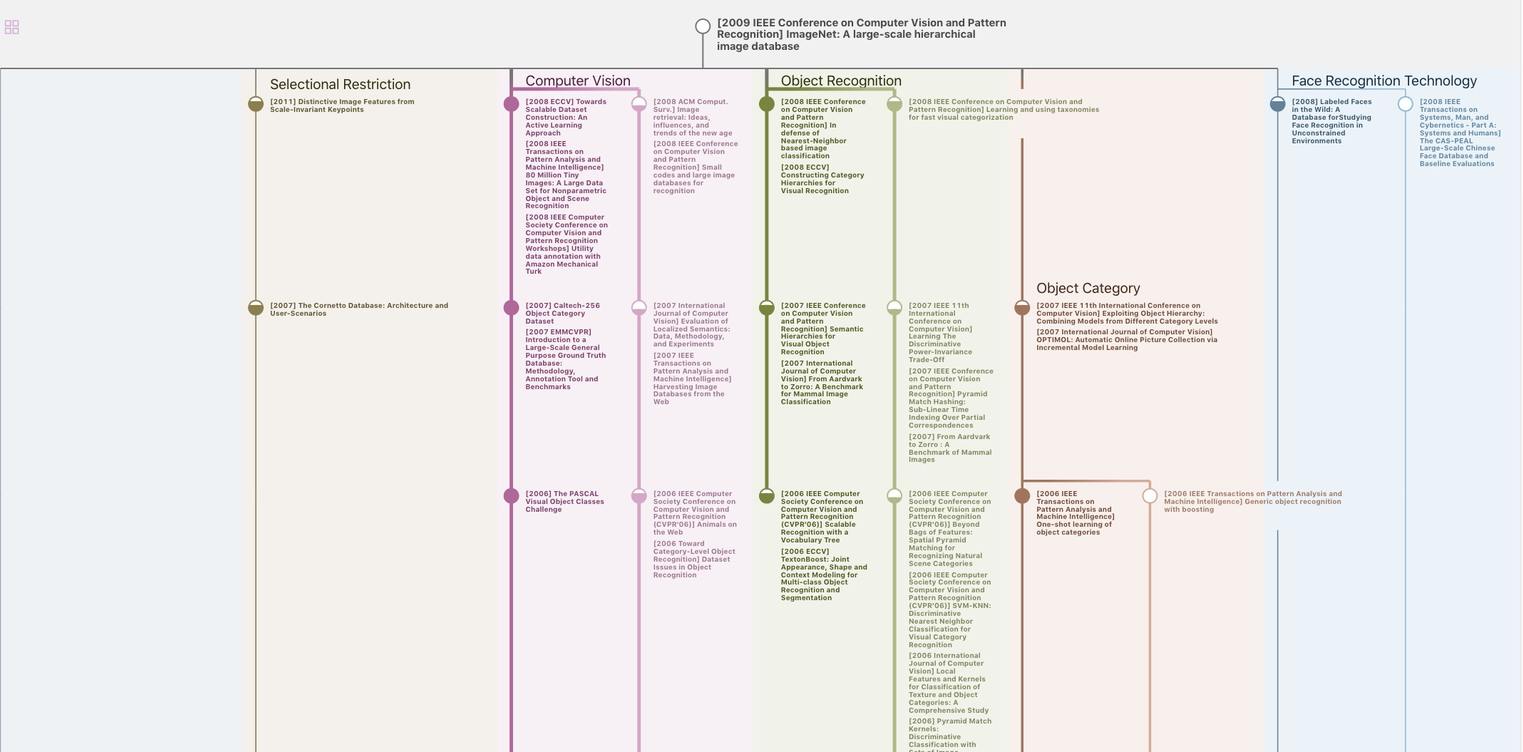
生成溯源树,研究论文发展脉络
Chat Paper
正在生成论文摘要