Authenticating Edge Neural Network through Hardware Security Modules and Quantum-Safe Key Management.
International Conference on VLSI Design(2024)
摘要
In the past decade, the usage and need for Deep Neural Networks (DNNs) have drastically risen across numerous application domains. In order to train these DNNs, a vast amount of data and effort is required, which naturally brings forth the question of Intellectual Property (IP) protection and privacy of the models. While this has been studied in the context of server-based Machine Learning (ML) inference tasks; in this paper, we draw attention to the deployment of a trained Neural Network (NN) in edge computing platforms. The primary challenges towards protecting edge NNs are first, to design a secure key management protocol between the model owner and an authenticated model user; second, to achieve low overhead while executing inference tasks even with security guarantees. We address both of these challenges and present an additional study by integrating post-quantum cryptographic primitives in the key management protocol. Our experimental studies with commercial edge computing platforms, and Hardware Security Modules (HSMs) present an efficient, practical, and robust scenario for the provisioning of ML tasks at the edge.
更多查看译文
关键词
Neural Network,Hardware Security,Hardware Security Module,Deep Neural Network,Low Overhead,Intellectual Property Protection,Types Of Attacks,Deep Neural Network Model,Secret Key,Public Key,Raspberry Pi,MNIST Dataset,Client-side,Threat Model,Key Distribution,Key Size,Encryption And Decryption,Key Exchange,Inference Step,One-time Cost,Cryptographic Keys
AI 理解论文
溯源树
样例
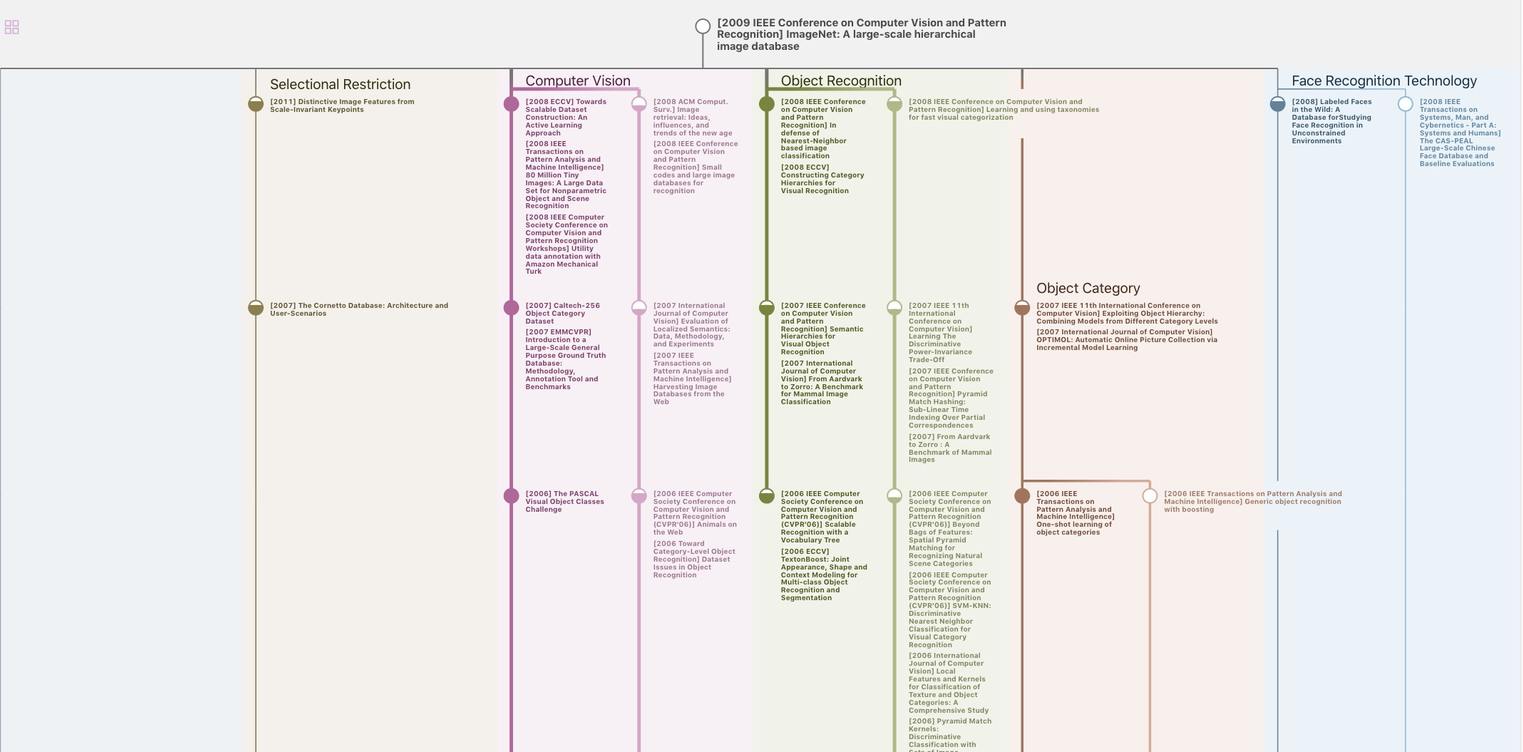
生成溯源树,研究论文发展脉络
Chat Paper
正在生成论文摘要