The Importance of Generalizability in Machine Learning for Systems
IEEE Computer Architecture Letters(2024)
摘要
Using machine learning (ML) to tackle computer systems tasks is gaining popularity. One of the shortcomings of such ML-based approaches is the inability of models to generalize to out-of-distribution data i.e., data whose distribution is different than the training dataset. We showcase that this issue exists in cloud environments by analyzing various ML models used to improve resource balance in Google's fleet. We discuss the trade-offs associated with different techniques used to detect out-of-distribution data. Finally, we propose and demonstrate the efficacy of using Bayesian models to detect the model's confidence in its output when used to improve cloud server resource balance
更多查看译文
AI 理解论文
溯源树
样例
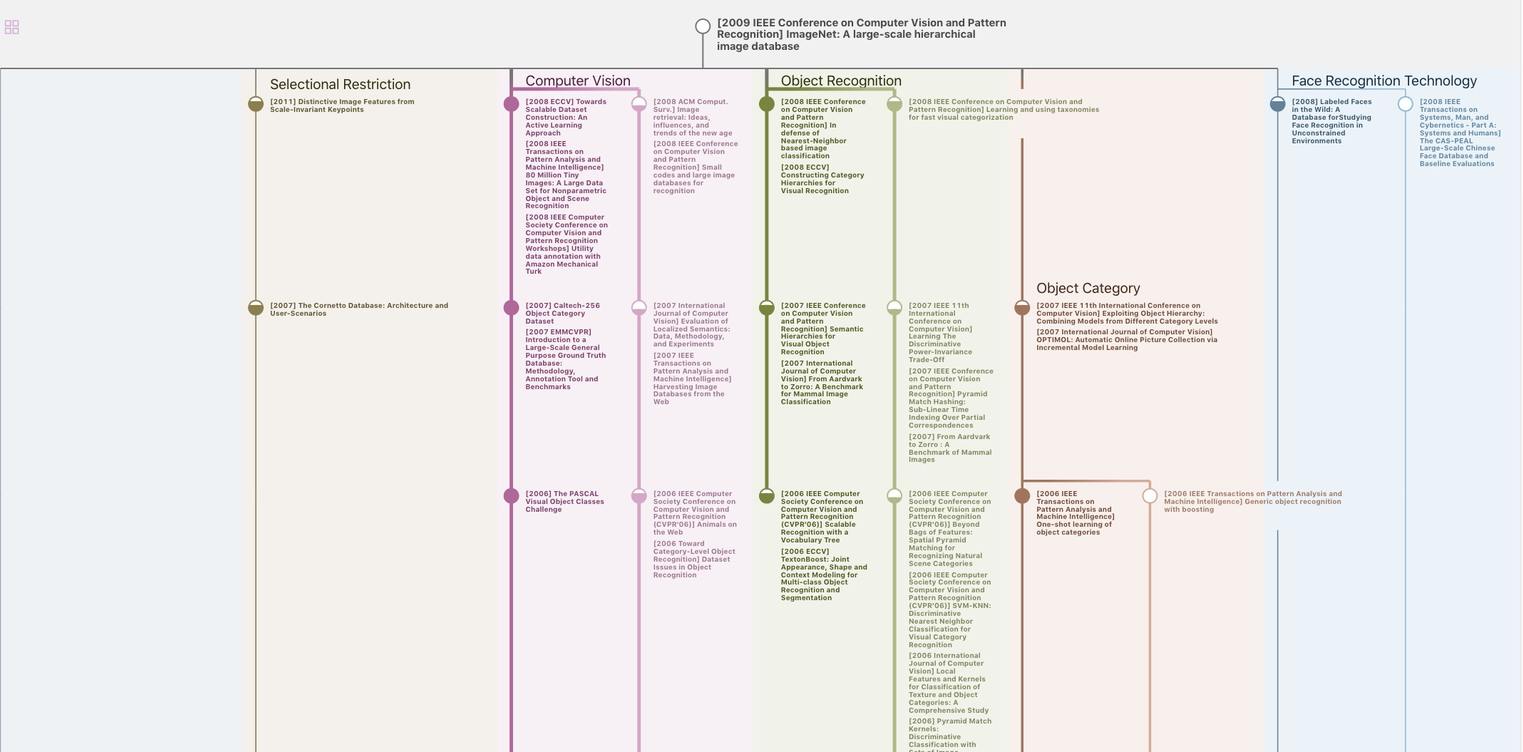
生成溯源树,研究论文发展脉络
Chat Paper
正在生成论文摘要